Unified Convergence Theory of Stochastic and Variance-Reduced Cubic Newton Methods
arXiv (Cornell University)(2023)
摘要
We study stochastic Cubic Newton methods for solving general possibly non-convex minimization problems. We propose a new framework, which we call the helper framework, that provides a unified view of the stochastic and variance-reduced second-order algorithms equipped with global complexity guarantees. It can also be applied to learning with auxiliary information. Our helper framework offers the algorithm designer high flexibility for constructing and analyzing the stochastic Cubic Newton methods, allowing arbitrary size batches, and the use of noisy and possibly biased estimates of the gradients and Hessians, incorporating both the variance reduction and the lazy Hessian updates. We recover the best-known complexities for the stochastic and variance-reduced Cubic Newton, under weak assumptions on the noise. A direct consequence of our theory is the new lazy stochastic second-order method, which significantly improves the arithmetic complexity for large dimension problems. We also establish complexity bounds for the classes of gradient-dominated objectives, that include convex and strongly convex problems. For Auxiliary Learning, we show that using a helper (auxiliary function) can outperform training alone if a given similarity measure is small.
更多查看译文
关键词
newton,stochastic,methods,variance-reduced
AI 理解论文
溯源树
样例
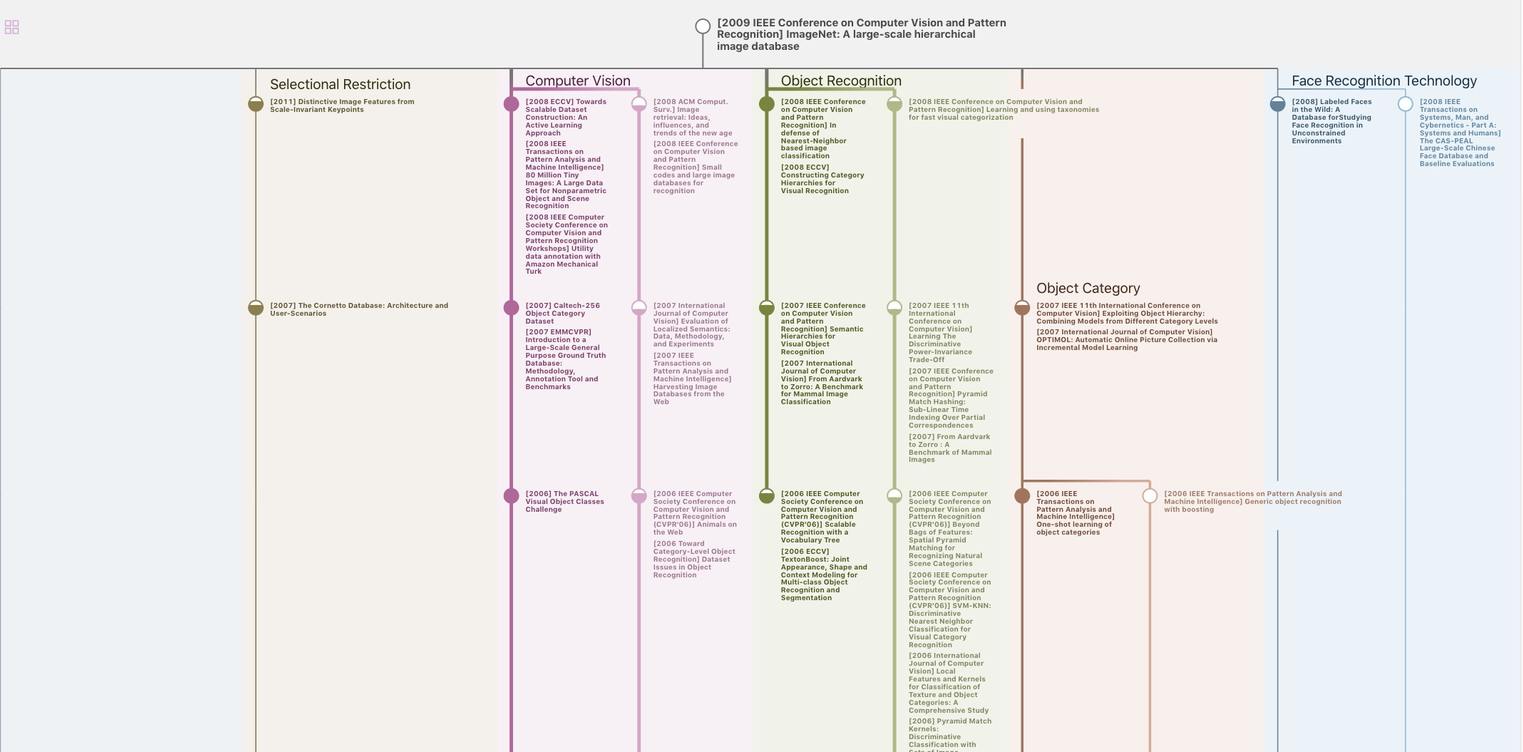
生成溯源树,研究论文发展脉络
Chat Paper
正在生成论文摘要