Prediction of the SYM-H Index Using a Bayesian Deep Learning Method With Uncertainty Quantification
SPACE WEATHER-THE INTERNATIONAL JOURNAL OF RESEARCH AND APPLICATIONS(2024)
摘要
We propose a novel deep learning framework, named SYMHnet, which employs a graph neural network and a bidirectional long short-term memory network to cooperatively learn patterns from solar wind and interplanetary magnetic field parameters for short-term forecasts of the SYM-H index based on 1- and 5-min resolution data. SYMHnet takes, as input, the time series of the parameters' values provided by NASA's Space Science Data Coordinated Archive and predicts, as output, the SYM-H index value at time point t + w hours for a given time point t where w is 1 or 2. By incorporating Bayesian inference into the learning framework, SYMHnet can quantify both aleatoric (data) uncertainty and epistemic (model) uncertainty when predicting future SYM-H indices. Experimental results show that SYMHnet works well at quiet time and storm time, for both 1- and 5-min resolution data. The results also show that SYMHnet generally performs better than related machine learning methods. For example, SYMHnet achieves a forecast skill score (FSS) of 0.343 compared to the FSS of 0.074 of a recent gradient boosting machine (GBM) method when predicting SYM-H indices (1 hr in advance) in a large storm (SYM-H = -393 nT) using 5-min resolution data. When predicting the SYM-H indices (2 hr in advance) in the large storm, SYMHnet achieves an FSS of 0.553 compared to the FSS of 0.087 of the GBM method. In addition, SYMHnet can provide results for both data and model uncertainty quantification, whereas the related methods cannot. In the past several years, machine learning and its subfield, deep learning, have attracted considerable interest. Computer vision, natural language processing, and social network analysis make extensive use of machine learning algorithms. Recent applications of these algorithms include the prediction of solar flares and the forecasting of geomagnetic indices. In this paper, we propose an innovative machine learning method that utilizes a graph neural network and a bidirectional long short-term memory network to cooperatively learn patterns from solar wind and interplanetary magnetic field parameters to provide short-term predictions of the SYM-H index. In addition, we present techniques for quantifying both data and model uncertainties in the output of the proposed method. SYMHnet is a novel deep learning method for making short-term predictions of the SYM-H index (1 or 2 hr in advance) With Bayesian inference, SYMHnet can quantify both aleatoric (data) and epistemic (model) uncertainties in making its prediction SYMHnet generally performs better than related machine learning methods for SYM-H forecasting
更多查看译文
关键词
bayesian deep learning method,uncertainty quantification
AI 理解论文
溯源树
样例
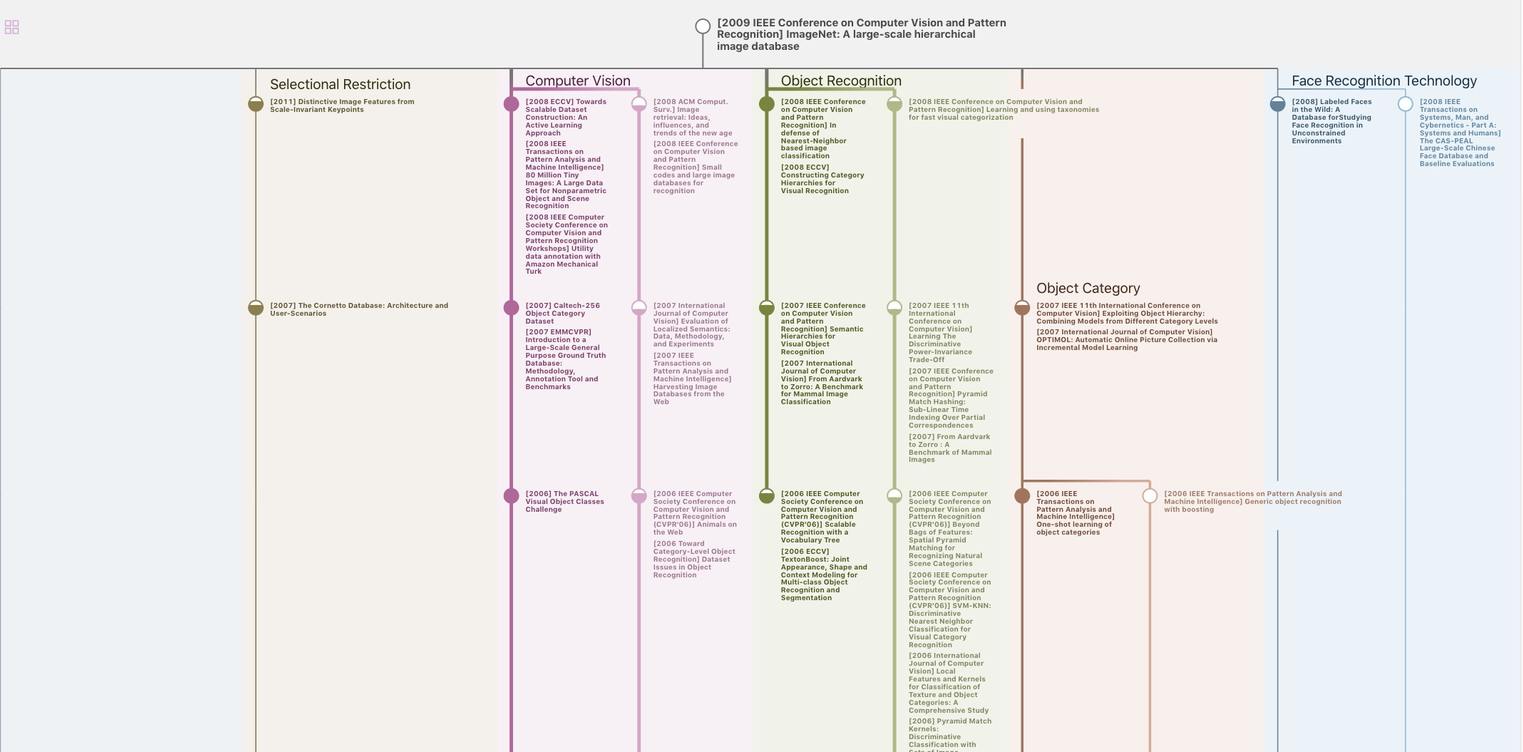
生成溯源树,研究论文发展脉络
Chat Paper
正在生成论文摘要