Pseudo-shot Learning for Soil Classification With Laser-Induced Breakdown Spectroscopy.
IEEE Trans. Artif. Intell.(2024)
摘要
Laser-induced breakdown spectroscopy (LIBS) has become an emerging analytical technique for soil analysis. The application of machine learning for quantitative and qualitative analysis has made LIBS more promising. However, the emission line distribution can be highly variable due to the soil samples' varied physical properties and/or chemical composition. It may cause spectra distribution change and make the training spectra not accurately reflect the test spectra distribution. Hence the test performance is deteriorated by applying an ML model trained on samples from the training distribution to the test distribution. To handle the spectra distribution problem, we propose using pseudo-shot learning with Siamese networks, a domain adaptation (DA) method to incorporate pseudo-labelled samples based on similarity confidence into the parameter estimation procedure. Considering the domain transfer differences among classes, we categorize the classes as hard, normal, and easy to reflect the class transfer difficulties in DA. We mainly focus on the hard classes as samples from these classes are not representative of the source domain and can easily be misclassified in the prediction phase. Few-shot learning is used to find the spectra from hard classes but misclassified into their similar classes. These spectra are included to co-train the model with source samples to improve the test performance of hard classes. Our framework is tested with the EMSLIBS dataset, which shows that it can effectively overcome the spectra distribution shift and achieves 94.12% test accuracy. It beats the current best-performing model using the same dataset.
更多查看译文
关键词
Artificial intelligence in agriculture,domain adaptation,LIBS,neural networks,pseudo-shot learning,self-learning,siamese networks,similarity learning,soil classification
AI 理解论文
溯源树
样例
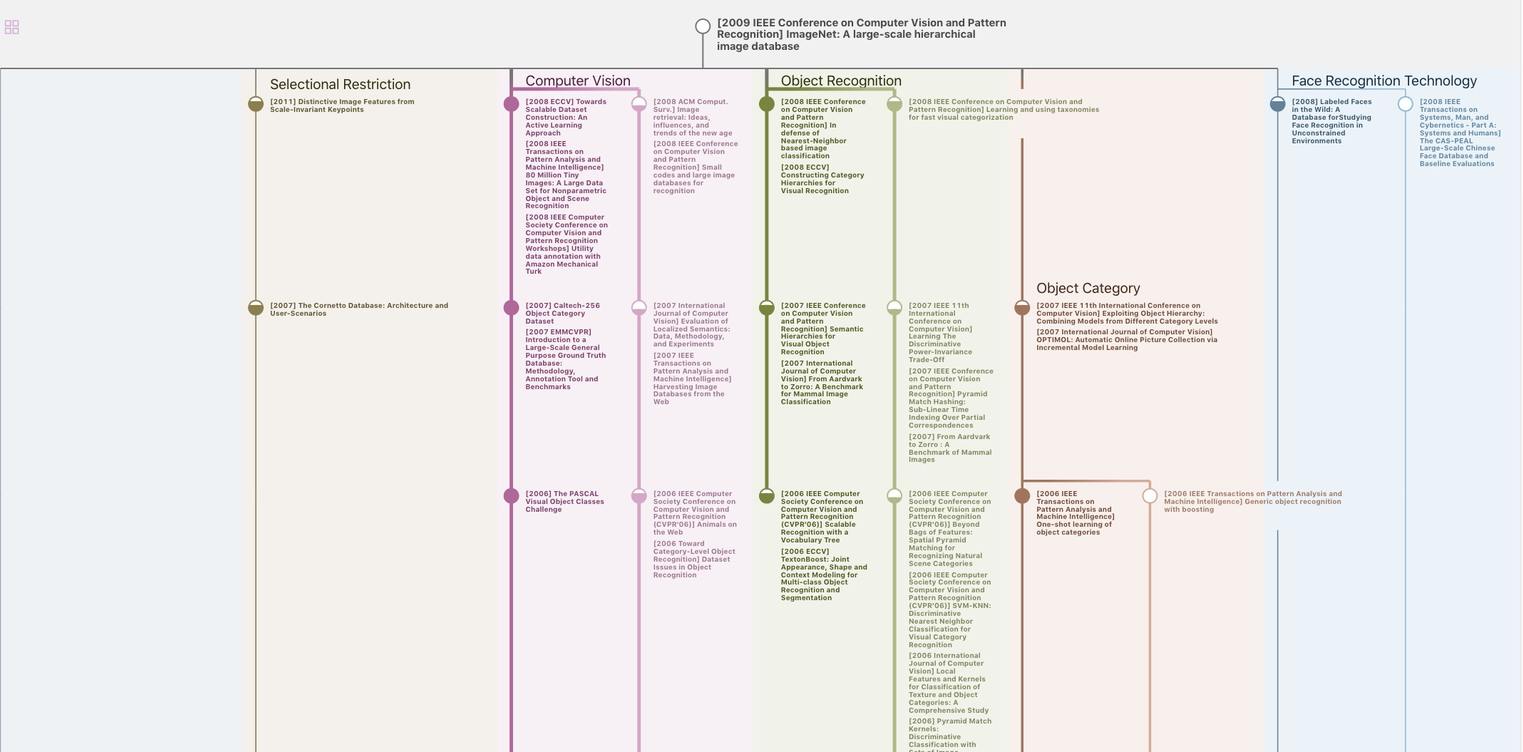
生成溯源树,研究论文发展脉络
Chat Paper
正在生成论文摘要