Abstract 4284: Enhancing subclonal reconstruction algorithm for resolving complex tumor phylogenies from multi-sample tumor DNA sequencing
Cancer Research(2023)
摘要
Abstract Cancer is characterized by the ongoing accumulation of somatic mutations, providing selective advantages that may lead to dysregulated cellular proliferation. While the cancer genome at diagnosis has been extensively studied, many cancer types still lack strong prognostic biomarkers. The continuous acquisition and selection for driver mutations in a population of cancer cells acts as a Darwinian process, resulting in clonal expansions of progressively more aberrant and fit phenotypes. Reconstructing tumor evolution allows us to understand key events that drive cancer progression and patterns of mutation co-occurrence within clones. These evolutionary features guide our understanding of fundamental mechanisms that lead to disease lethality. Inferring tumor evolution from DNA sequencing data is becoming part of routine analysis in cancer research. As sequencing costs drop, sequencing multiple tumor samples from a patient becomes routine. These multiple samples can represent different spatial regions of a tumor, longitudinal samples from a single region or a combination of both. This provides an opportunity to study tumor evolution in much greater detail and accuracy than was previously feasible through single-sample datasets. The most widely used methods to reconstruct the subclonal evolution of a tumor utilize stochastic-search algorithms. These approaches iterate through a parameter space to select phylogenetic solutions that maximize the likelihood of observed sequencing data. They are optimized for low complexity cases, where the size and number or subclones are relatively limited. As tumor subclonal structure increases in complexity, the parameter space grows exponentially, and stochastic-search algorithms become computationally intractable. For instance, recent benchmarking studies have revealed that many methods fail to reconstruct clone trees for data with as few as ten subclones. To circumvent current computational limitations, we developed a deterministic algorithm for subclonal reconstruction that leverages fundamental principles of cancer biology to encode heuristics that reduce the solution space to biologically plausible phylogenies. When applied to samples (4-36 tumors; median 16) from 12 patients with metastatic breast cancer, our method reduced the average runtime ten-fold. We were able to delineate the evolutionary history of up to 57 distinct subclones per patient, which is infeasible with most current methods. Benchmarking using methods developed for the SMCHet DREAM challenge on real and simulated datasets further quantifies the accuracy, resolution, and scalability. We have thus presented a novel method for rapid and optimized reconstruction of tumor evolutionary histories. Citation Format: Helena Winata, Daniel Knight, Juber A. Patel, Nicholas K. Wang, Pier Selenica, Stefan E. Eng, Caroline Kostrzewa, Jaron Arbet, Yingjie Zhu, Ronglai Shen, Jorge Reis-Filho, Pedram Razafi, Paul C. Boutros. Enhancing subclonal reconstruction algorithm for resolving complex tumor phylogenies from multi-sample tumor DNA sequencing. [abstract]. In: Proceedings of the American Association for Cancer Research Annual Meeting 2023; Part 1 (Regular and Invited Abstracts); 2023 Apr 14-19; Orlando, FL. Philadelphia (PA): AACR; Cancer Res 2023;83(7_Suppl):Abstract nr 4284.
更多查看译文
关键词
complex tumor phylogenies,subclonal reconstruction algorithm,multi-sample
AI 理解论文
溯源树
样例
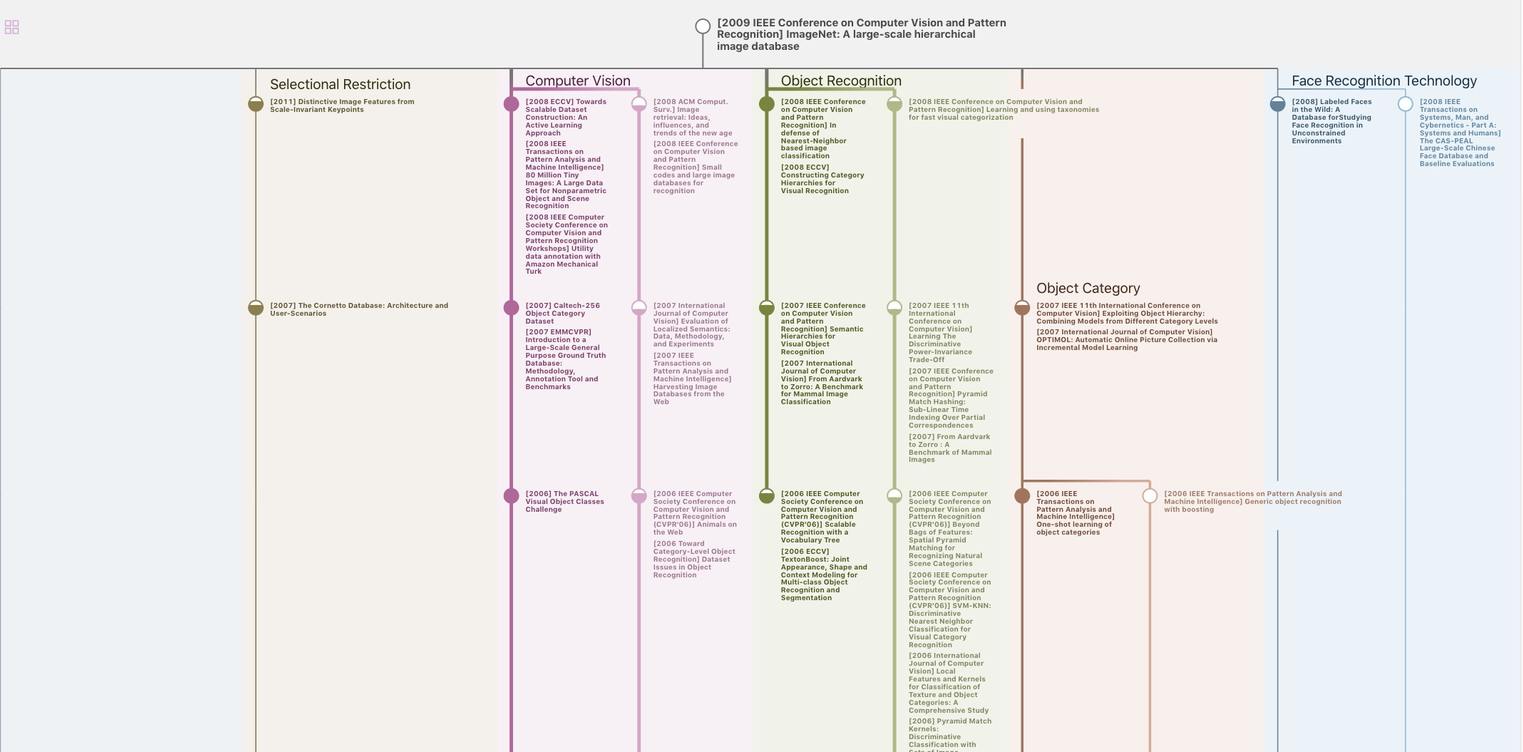
生成溯源树,研究论文发展脉络
Chat Paper
正在生成论文摘要