Memory-efficient low-compute segmentation algorithms for edge ultrasound devices: continuous bladder monitoring
Research Square (Research Square)(2023)
摘要
Abstract Post-operative urinary retention is a medical condition where patients cannot urinate despite having a full bladder. Ultrasound imaging of the bladder is intermittently used to estimate urine volume for early diagnosis and management of urine retention. Moreover, the use of bladder ultrasound can reduce the need for an indwelling urinary catheter and the risk of catheter-associated urinary tract infection. Wearable ultrasound devices combined with machine-learning based bladder volume estimation algorithms reduce the burdens of nurses in hospital settings and improve outpatient care. However, existing algorithms are memory and computation intensive, thereby demanding the use of expensive GPUs. In this paper, we develop and validate a low-compute memory-efficient deep learning model for accurate bladder region segmentation and urine volume calculation. B-mode ultrasound bladder images of 360 patients were divided into training and validation sets; another 74 patients were used as the test dataset. Our 1-bit quantized models with 4-bits and 6-bits skip connections achieved an accuracy within 3.8% and 2.6%, respectively, of a full precision state-of-the-art neural network (NN) without any floating-point operations and with an 11.5× and 9.0× reduction in memory requirements to fit under 150 kB. The means and standard deviations of the volume estimation errors, relative to estimates from ground-truth clinician annotations, were 5.0±33 ml and 6.8±29 ml, respectively. This lightweight NN can be easily integrated on the wearable ultrasound device for automated and continuous monitoring of urine volume. Our approach can potentially be extended to other clinical applications such as monitoring blood pressure and fetal heart rate.
更多查看译文
关键词
edge ultrasound devices,continuous bladder monitoring,segmentation,memory-efficient,low-compute
AI 理解论文
溯源树
样例
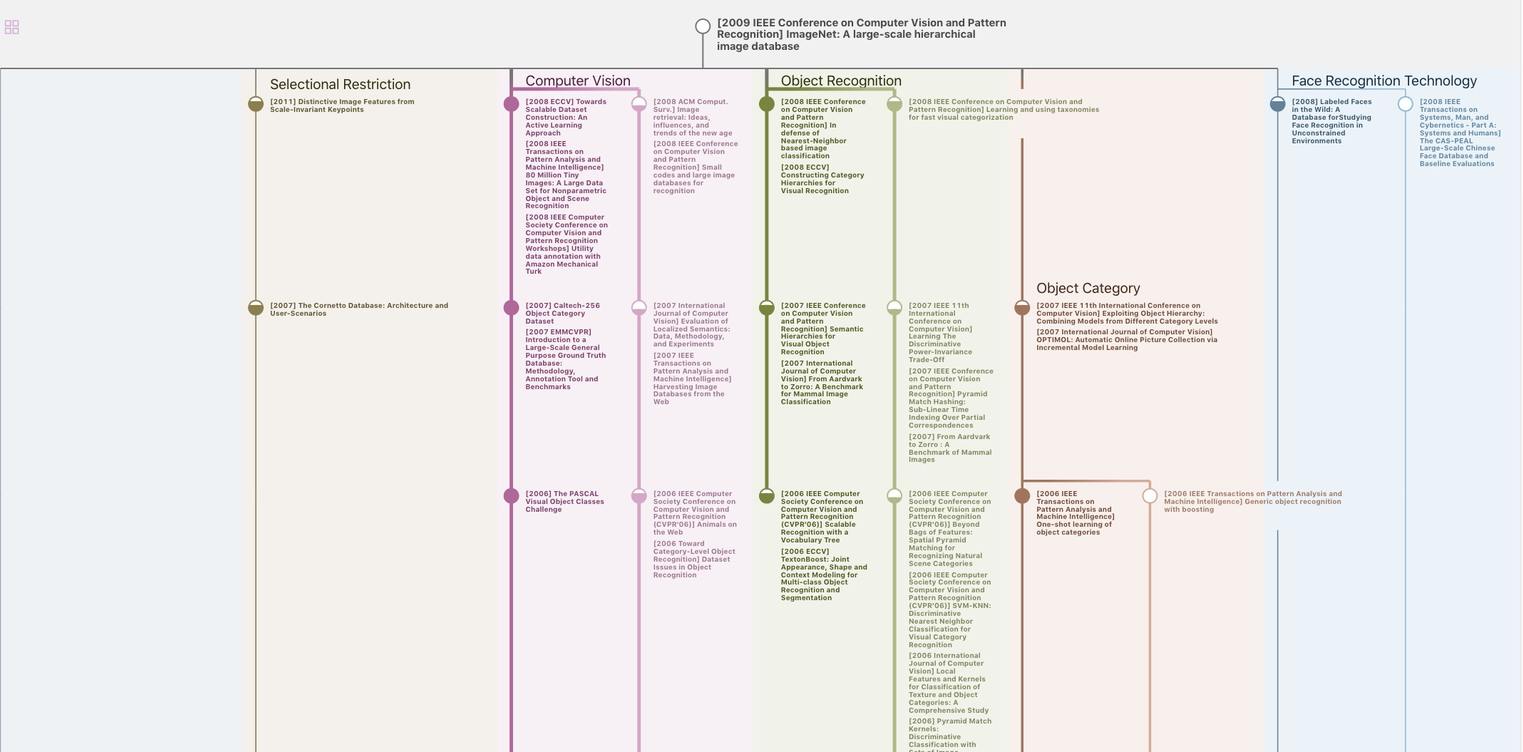
生成溯源树,研究论文发展脉络
Chat Paper
正在生成论文摘要