Distribution-Balanced Federated Learning for Fault Identification of Power Lines
IEEE TRANSACTIONS ON POWER SYSTEMS(2024)
摘要
The state-of-the-art centralized machine learning applied to fault identification trains the collected data from edge devices on the cloud server due to the limitation of computing resources on edge. However, data leakage possibility increases considerably when sharing data with other devices on the cloud server, while training performance may degrade without data sharing. The study proposes a federated fault identification scheme, named DBFed-LSTM, by combining the distribution-balanced federated learning with the attention-based bidirectional long short-term memory, which can efficiently transfer training processes from the cloud server to edge devices. Under data privacy protections, local devices and the cloud server are specialized for storage and calculation as well as for updating the global model of learning vital time-frequency characteristics, respectively. Given that different device data for monitoring a small probability event are generally non-independent identically distributed (non-IID), a global-model pre-training method and improved focal loss are accordingly proposed. It is verified by the case study that the DBFed-LSTM can be effectively implemented to challenge centralized training with data sharing while preserving privacy and alleviating cloud server computation pressure even for non-IID data. Furthermore, it represents a much preferable performance and robust model to centralized training without data sharing.
更多查看译文
关键词
Data models,Fault diagnosis,Training,Computational modeling,Servers,Object recognition,Internet of Things,Fault identification,federated learning,long short-term memory,data distribution
AI 理解论文
溯源树
样例
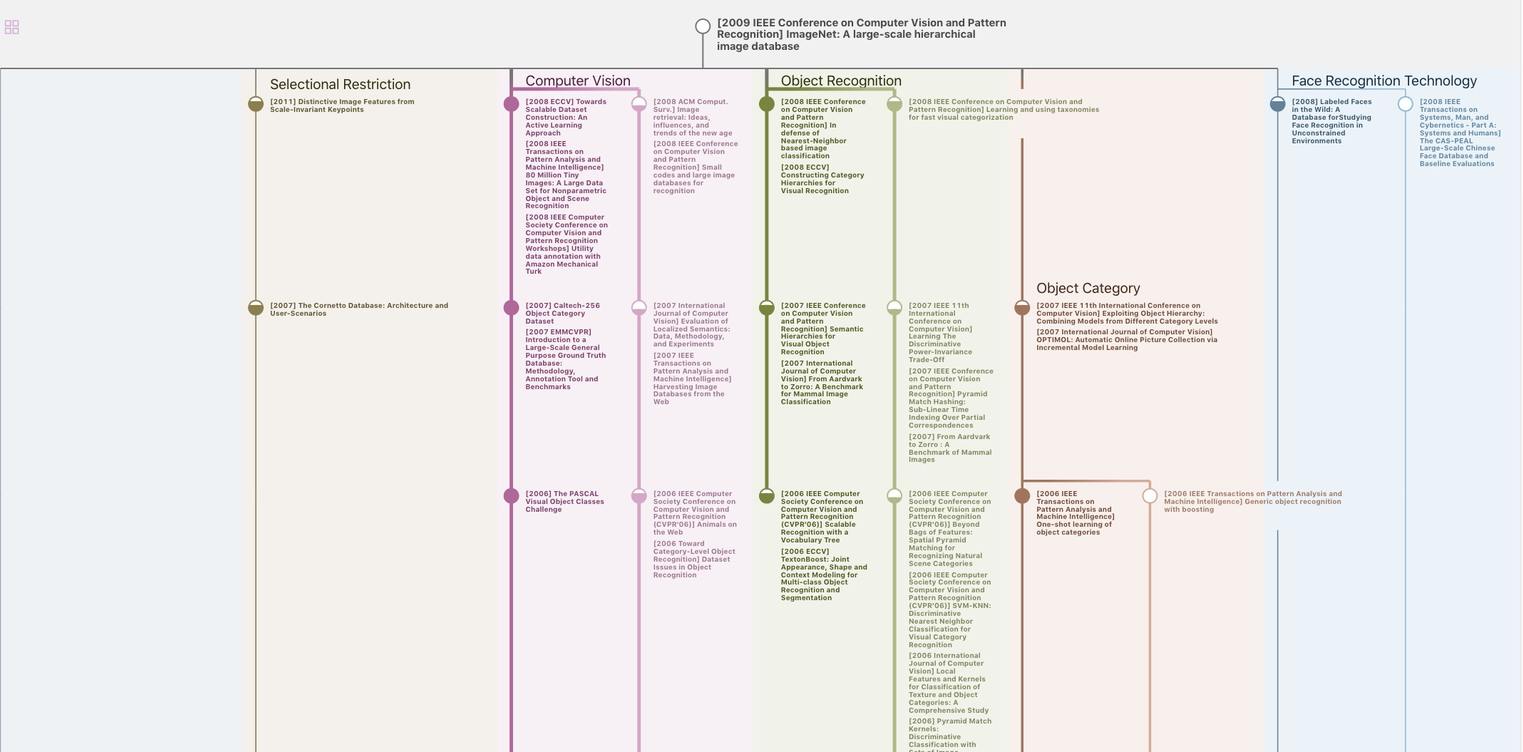
生成溯源树,研究论文发展脉络
Chat Paper
正在生成论文摘要