頭部運動機能特徴に基づく対話者の主観的印象の予測・説明モデルの構築
Transactions of The Japanese Society for Artificial Intelligence(2023)
摘要
An explainable model is proposed to automatically explain when and what kind of behaviors affected interlocutors’ impressions in group discussions. Focusing on self-reported scores on the impressions such as atmosphere and enjoyment felt during the discussions, we tackle a new problem that identifies both influential behaviors and their timings that were related to impression formation. To that end, this paper formulates the problem as the identification of behavioral features, which contributed to the impression prediction and the detection of the timings when such behaviors occurred very frequently. To solve this problem, this paper presents a two-fold framework consisting of a prediction model using random forest regressors, followed by an explanation model using a SHAP analysis. The prediction part employs functional head-movement features, which can capture the various aspects of interactive conversational behaviors. The key feature of the latter explanation part is the temporal decomposition of features’ contributions by integrating the SHAP values and the temporal distributions of the occurrence probabilities of the behavioral features, which were computed by a kernel density estimation method from the detected samples of head movements and their functions. To derive this process, we exploit the dual additivity in the SHAP values and the functional head-movement features over time. Finally, the influential behaviors and their timings are identified from the temporal local maximums of the features’ contribution distributions. Targeting 17-group 4-female discussions included in a SA-Off corpus, this paper shows some case studies and the results of quantitative evaluations, which compared the reported overall scores and two-minute-wise scores at the timings found by the model. These results confirmed the predictability and explainability of the proposed model.
更多查看译文
AI 理解论文
溯源树
样例
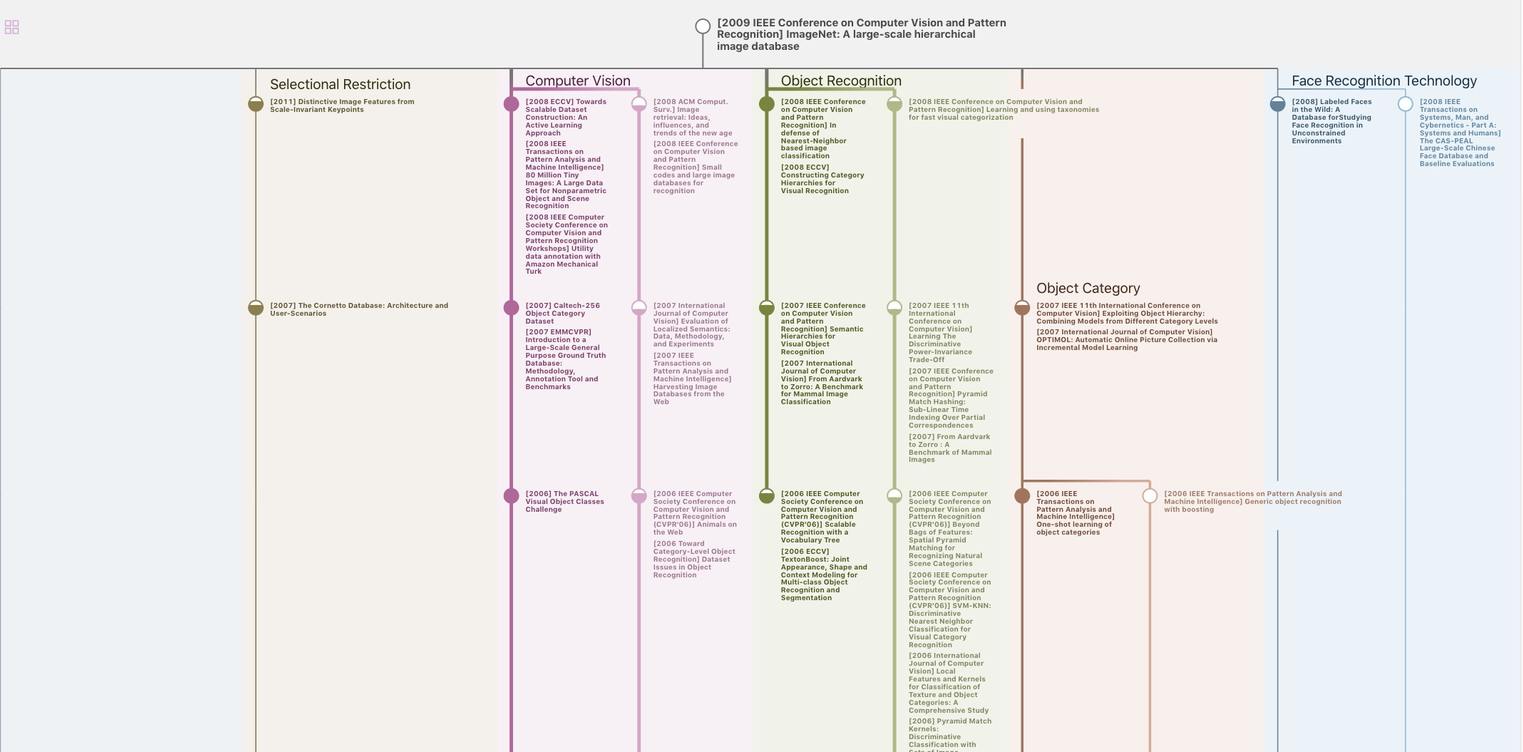
生成溯源树,研究论文发展脉络
Chat Paper
正在生成论文摘要