An analysis of physiological signals as a measure of engagement in rehabilitation using machine learning algorithms
Research Square (Research Square)(2023)
摘要
Abstract Background: Active participation of clients in rehabilitation is a crucial factor for improved outcomes. A few studies have investigated how to measure patient engagement to given rehabilitation therapies compared to intervention developments. In this study, we aimed to examine the possibility of assessing patient engagement by analyzing features computed from autonomic nervous responses with machine learning algorithms, such as naïve bayes classifier (NB), random forest (RF), support vector machine (SVM), and neural network (NN). Methods: Autonomic nervous responses were collected from 56 older adults with moderate to mild cognitive impairment using a wearable device during rehabilitation therapy sessions under two different conditions designed to induce different levels of engagement. 32 features were obtained from the autonomic nervous system responses and used to determine the client engagement levels. In addition, feature selection methods were applied to reduce redundant information. Client engagement during rehabilitation therapy sessions was rated by therapists using Pittsburgh Rehabilitation Participation Scale (PRPS). In addition, subjective feelings were quantified by participants using Self-Assessment Manikin (SAM). The data collected under the different conditions were labelled as having a higher or lower degree of engagement, and classification performance was tested with the algorithms. Results: It was found that the mean scores of PRPS and the valence dimension of the participants were significantly different between the conditions confirming the successful induction of varying levels of engagement from the participants. The worst performance was observed when the classifiers were trained with all features (mean classification accuracy: 81.6%) whereas the highest mean accuracy was observed when classifiers were trained with the top 5 features (85.6%) selected by minimum-Redundancy-Maximum-Relevance (mRMR) than other combinations of feature sets. The best classification accuracy of different degrees of rehabilitation participation was achieved with SVM (87.7%) in combination with the top 5 ranked features, which indicates that the feature selection methods employed in this study did not only lead to a reduction in the number of features, but also a slight improvement in the classification performance. On the other hand, RF consistently performed better than NB and NN when combined with the mRMR feature selection method, suggesting RF is the most robust classifier among the tested classifiers for determining the degree of client engagement in rehabilitation. Conclusions: Engagement in rehabilitation can be realized by utilizing autonomic nervous responses and machine learning algorithms as a way of involving clients in the process of engagement assessment while requiring their minimal cognitive efforts.
更多查看译文
关键词
rehabilitation,physiological signals,machine learning algorithms
AI 理解论文
溯源树
样例
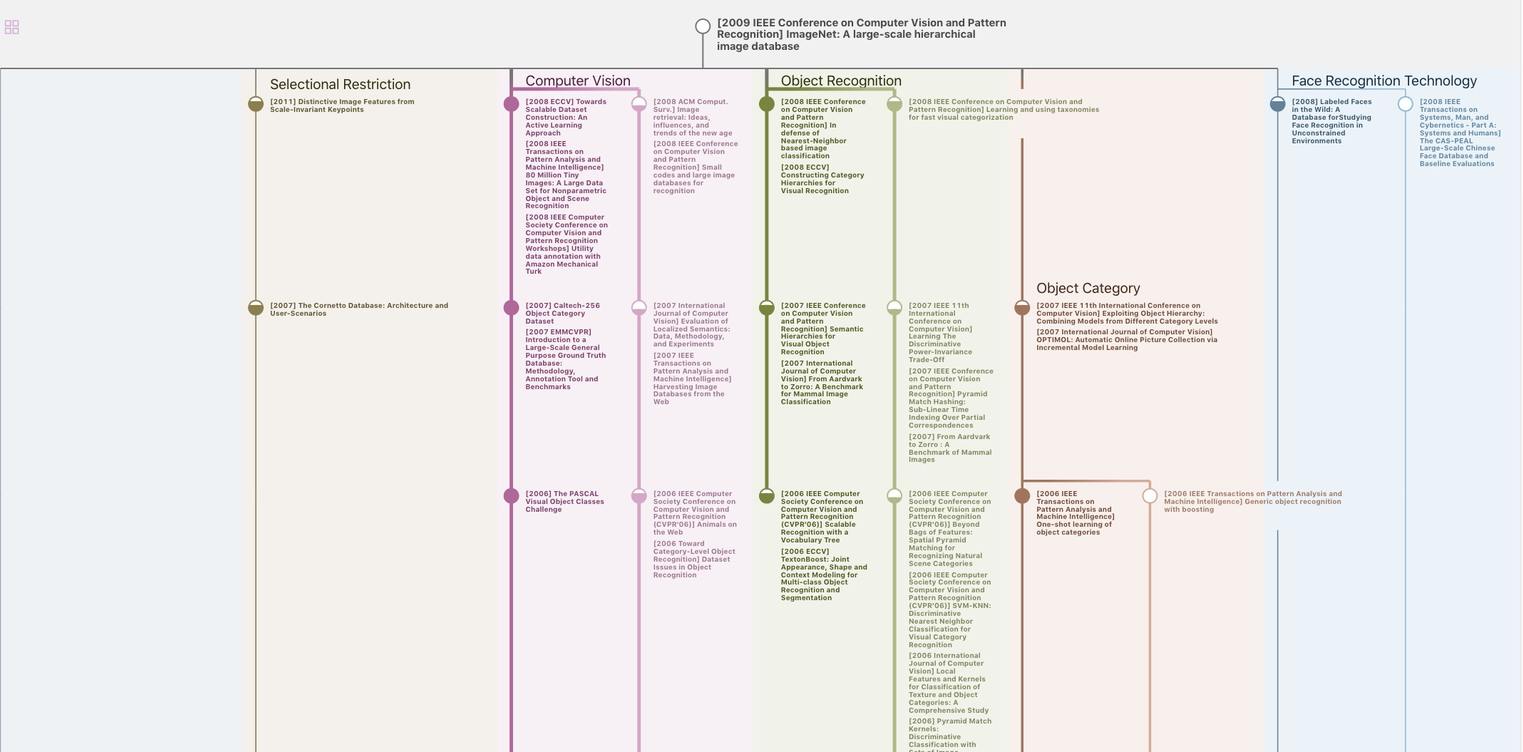
生成溯源树,研究论文发展脉络
Chat Paper
正在生成论文摘要