DiffCLIP: Leveraging Stable Diffusion for Language Grounded 3D Classification
arXiv (Cornell University)(2023)
摘要
Large pre-trained models have had a significant impact on computer vision by enabling multi-modal learning, where the CLIP model has achieved impressive results in image classification, object detection, and semantic segmentation. However, the model's performance on 3D point cloud processing tasks is limited due to the domain gap between depth maps from 3D projection and training images of CLIP. This paper proposes DiffCLIP, a new pre-training framework that incorporates stable diffusion with ControlNet to minimize the domain gap in the visual branch. Additionally, a style-prompt generation module is introduced for few-shot tasks in the textual branch. Extensive experiments on the ModelNet10, ModelNet40, and ScanObjectNN datasets show that DiffCLIP has strong abilities for 3D understanding. By using stable diffusion and style-prompt generation, DiffCLIP achieves an accuracy of 43.2\% for zero-shot classification on OBJ\_BG of ScanObjectNN, which is state-of-the-art performance, and an accuracy of 80.6\% for zero-shot classification on ModelNet10, which is comparable to state-of-the-art performance.
更多查看译文
关键词
diffclip,stable diffusion,classification,3d
AI 理解论文
溯源树
样例
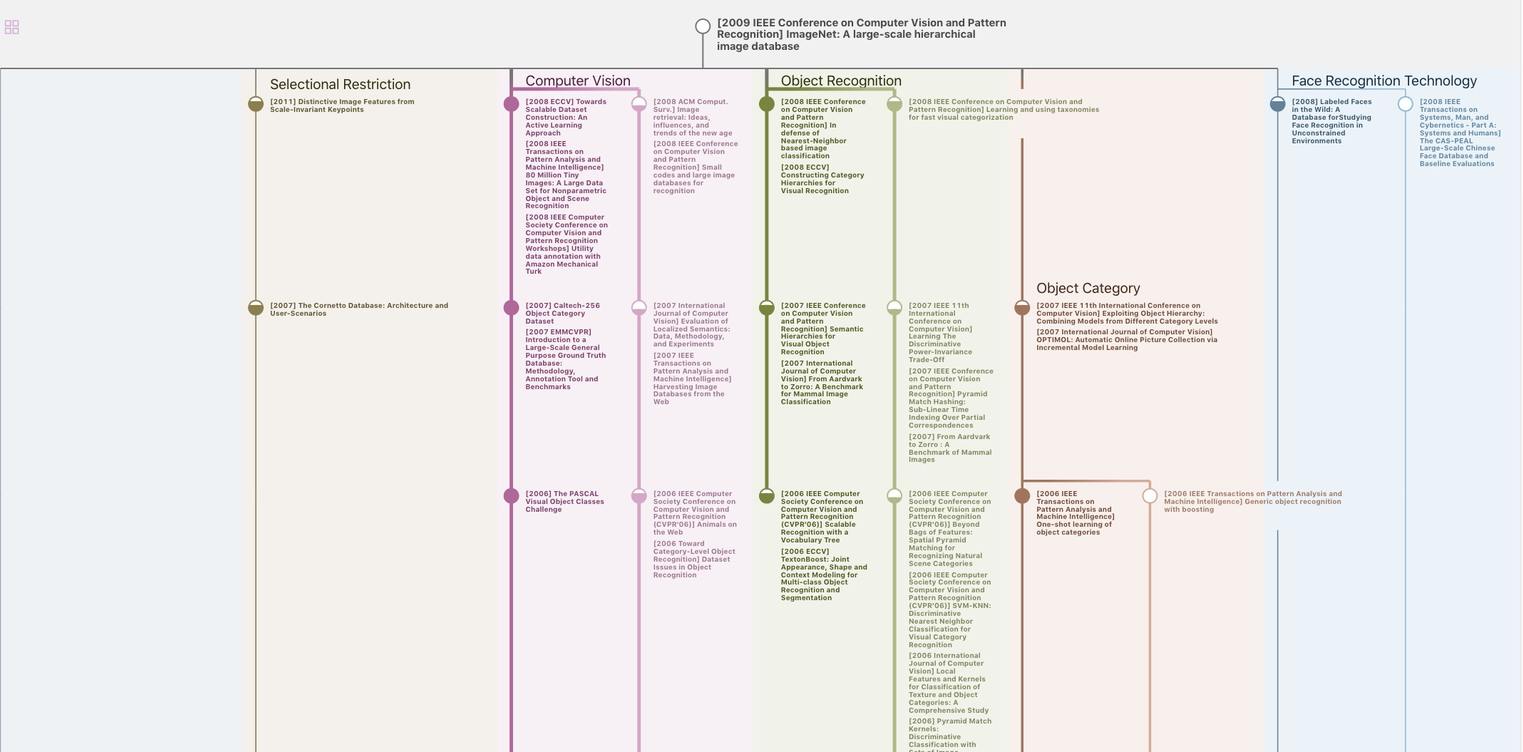
生成溯源树,研究论文发展脉络
Chat Paper
正在生成论文摘要