Ensemble deep neural models for automated abnormality detection and classification in precision care applications
Elsevier eBooks(2023)
摘要
Radiological imaging is one of the most relied upon modalities in the clinical diagnosis and treatment planning process. Conventional diagnosis involves the manual analysis of radiology images by experienced radiologists, which is often a time-consuming and labor-intensive process. The scarcity of experienced radiologists and necessity of large-scale X-rays image analysis given the huge diagnosis workload at most hospitals stresses the need for automated clinical diagnosis systems capable of fast and accurate identification of abnormalities, disease characteristic identification, disease classification, and others. Such automated methods are thus a fundamental requirement in clinical workflow management applications. In this work, we present an approach for multitask clinical objectives such as disease classification and detection of abnormalities. The proposed model leverages the predictive power of deep neural models for enabling evidence-based diagnosis. During validation experiments, the model achieved an accuracy of 89.58% along with sensitivity and specificity of 85.83% and 90.83%, respectively, with an AUC (area under the ROC curve) of 95.84% for normal/no findings versus COVID-19 chest radiograph classification and an accuracy of 73.19% for upper extremity musculoskeletal images. The performance of the model for the classification and abnormality identification tasks, when benchmarked over multiple standard datasets, emphasizes its suitability and adaptability in real-world clinical settings, with significant improvements in radiology-based diagnosis workflow and patient care.
更多查看译文
关键词
ensemble deep neural models,abnormality detection,classification,precision
AI 理解论文
溯源树
样例
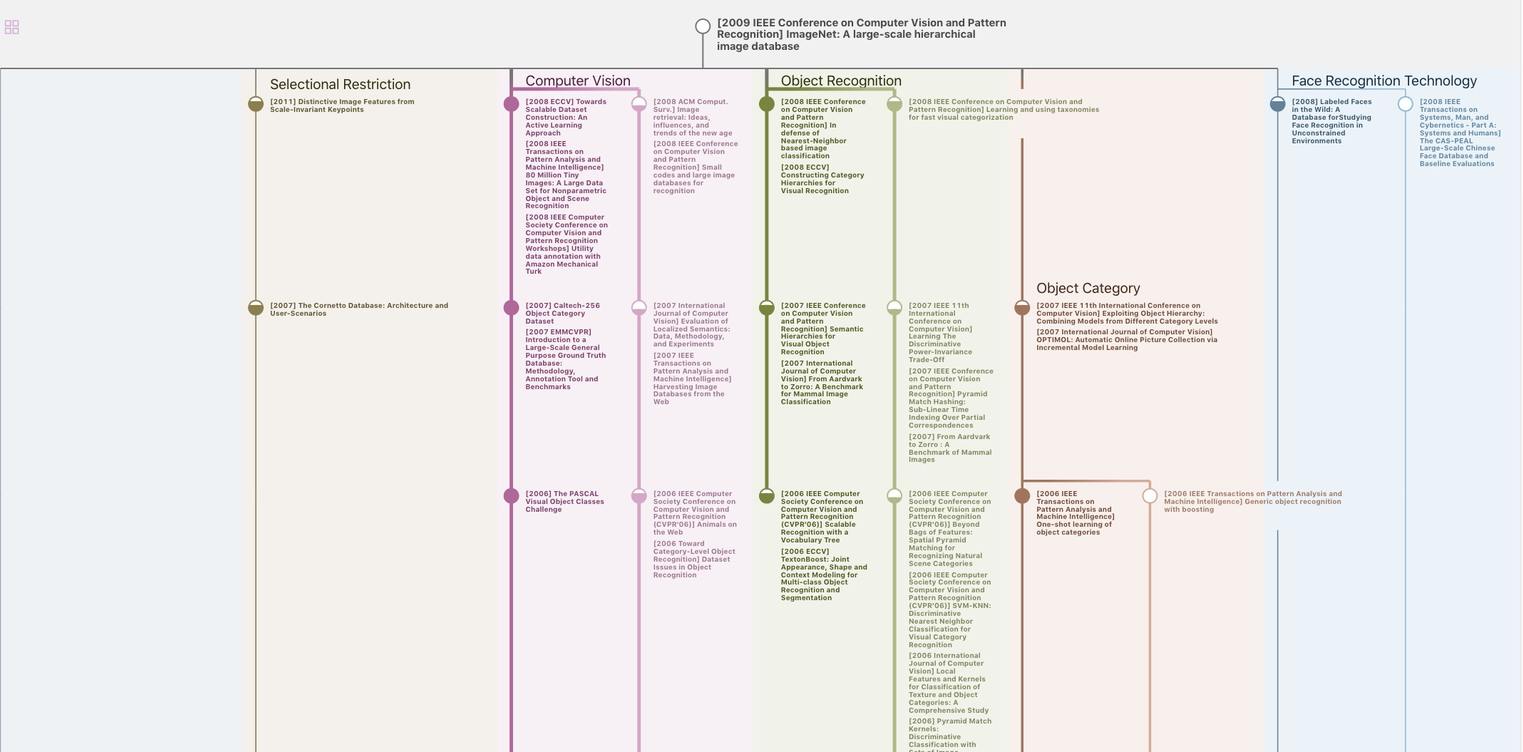
生成溯源树,研究论文发展脉络
Chat Paper
正在生成论文摘要