Competitive Pricing for Resource Trading in Sliced Mobile Networks: A Multi-Agent Reinforcement Learning Approach.
IEEE Trans. Mob. Comput.(2024)
摘要
The emergence of network slicing as a flagship technology in 5G networks has not only enhanced network expansion and flexibility in resource management for service continuity, but also provided an avenue for establishing a viable market for resource sharing. To optimize the network's resource usage, stakeholders are encouraged to take pragmatic steps toward dynamic resource sharing. This paper designs a techno-economic model for the strategic interactions among multiple competing mobile virtual network operators (MVNOs) and their users in a trading marketplace. We formulate the dynamic pricing problem as a two-stage Stackelberg game, where the MVNOs are leaders, and the users are followers. In the first stage, the MVNOs compete to set their differentiated unit prices using a negotiation mechanism while considering system-level network load. Then, the users decide their purchasing volumes to match the prices of the MVNOs. We transform the game-based optimization problem into a stochastic Markov decision process (MDP) problem and propose a multi-agent deep Q-network (MADQN) method that obtains an optimal solution for the formulated game. Simulation results and analysis reveal that the proposed algorithm achieves convergence under the competitive pricing scheme (CPS) and independent pricing scheme (IPS) while enhancing MVNOs and users' utilities at acceptable levels.
更多查看译文
关键词
network slicing,Stackelberg game,resource trading,competitive pricing,MADQN
AI 理解论文
溯源树
样例
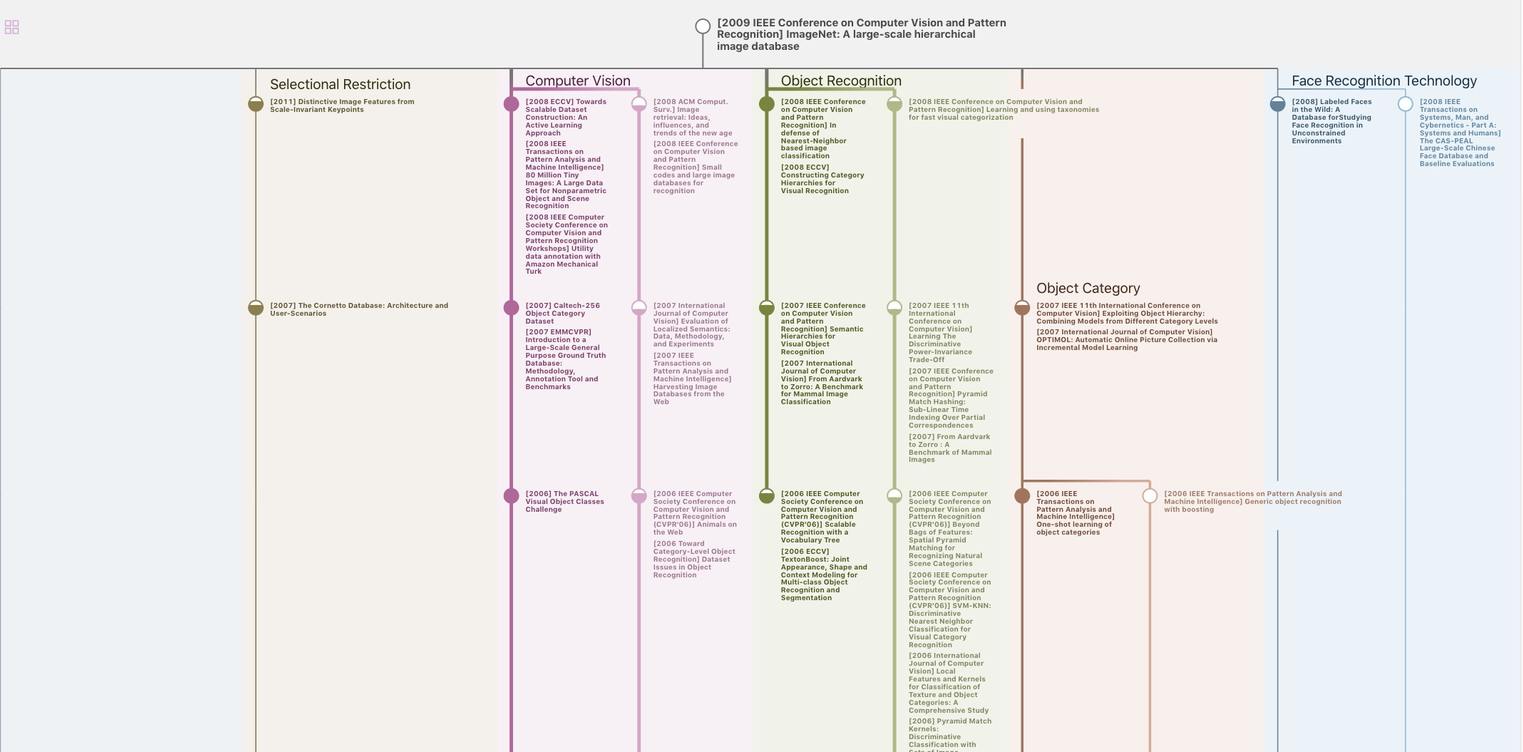
生成溯源树,研究论文发展脉络
Chat Paper
正在生成论文摘要