0148 Early Diagnosis of Post-Traumatic Stress Disorder in Nurses based on Physical Behavior and Sleep Actigraphy using Machine Learning
Sleep(2023)
摘要
Abstract Introduction Disturbances in sleep/wake patterns and circadian rhythms are common in post-traumatic stress disorder (PTSD). Nurses are one population particularly susceptible to both sleep/circadian disruption and PTSD, given they frequently engage in shift work and experience high occupational stress. However, the specific types of sleep and circadian disruptions in PTSD have not been identified in nurses using validated measures of sleep, limiting potential intervention efforts. Methods To address this gap, we developed a machine learning algorithm for calculating risk of PTSD in nurses based on sleep, light, and physical activity patterns derived from actigraphy, in conjunction with inflammatory biomarkers. Participants were 392 nurses (mean age = 39.54, 92% female, 78% White) recruited from two Dallas-area hospitals for a larger longitudinal study on sleep and vaccine response. At baseline, nurses reported on demographics and PTSD symptoms using the PTSD Checklist for DSM-5 (PCL-5). Nurses were categorized as having probable PTSD if they reported scores >31 on the PCL-5. Nurses wore actigraphy devices for two weeks to track sleep/wake, activity counts, and light exposure. In the middle of the two-week period, nurses also had a blood draw to determine inflammatory markers interleukin-6 (IL-6), C-reactive protein (CRP), tumor necrosis factor-alpha (TNF-α), IL-1 beta (IL-1β), and interferon gamma-induced protein 10 (IP-10). Results 4.7% of nurses met criteria for probable PTSD. Using the actigraphy and inflammatory biomarker data, a Recurrent Neural Network (RNN) was developed to detect PTSD risk. Furthermore the Ridge Classifier was tested using activity counts, light exposure, and sleep/wake data to obtain an accuracy score of 0.97 and an F1 score (ranging between 0 and 1) of 0.96, indicating that the model was able to balance and maximize precision and determine PTSD risk successfully. Conclusion Using a novel machine learning algorithm, we show that actigraphy data can be used to accurately and non-invasively detect PTSD risk among nurses. Although additional validation is needed, results tentatively highlight the utility of harnessing real-time data to inform just-in-time adaptive interventions for PTSD among nurses. Given the essential role nurses play in the healthcare system, finding ways to support their well-being is of critical public health importance. Support (if any) NIH/NIAID R01AI128359
更多查看译文
关键词
sleep actigraphy,stress,nurses,physical behavior,post-traumatic
AI 理解论文
溯源树
样例
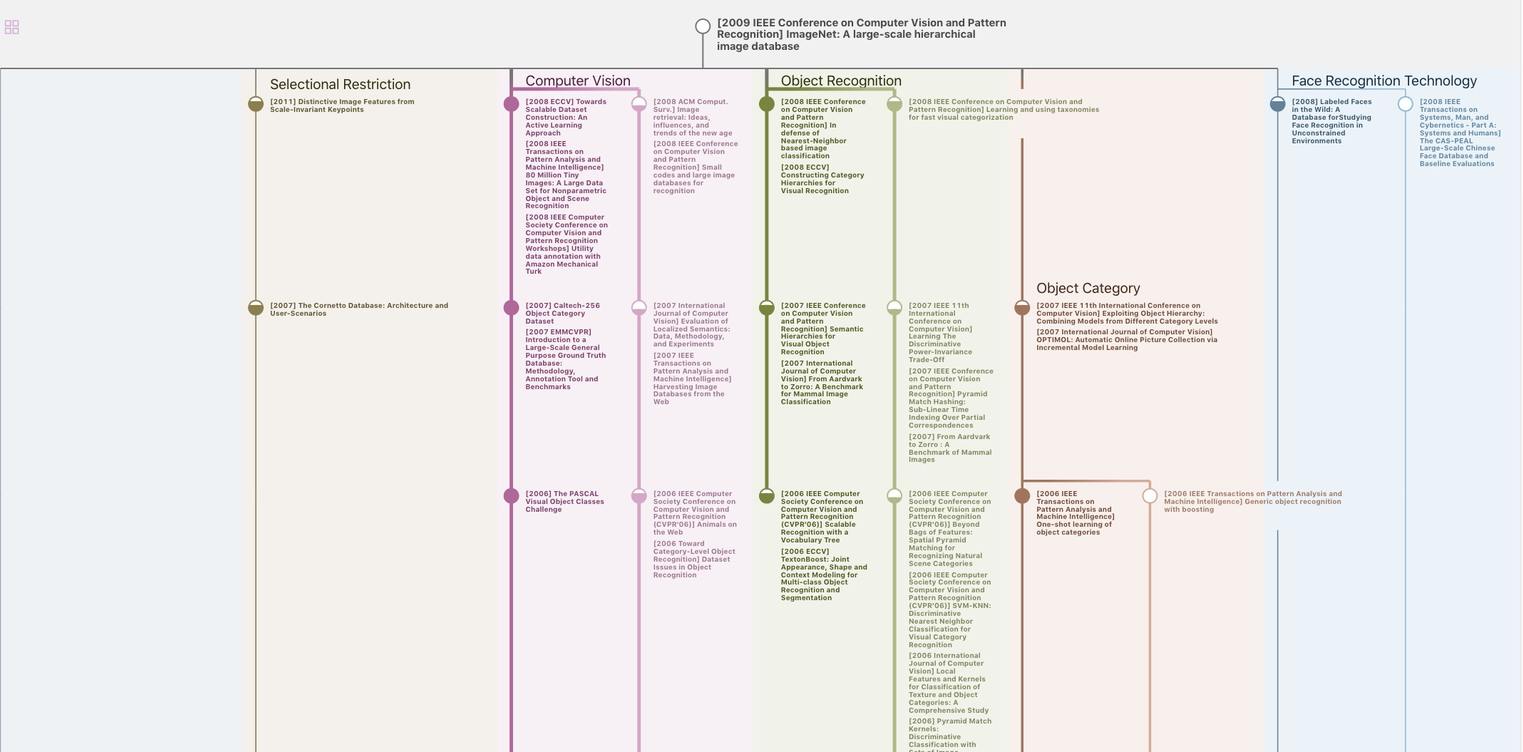
生成溯源树,研究论文发展脉络
Chat Paper
正在生成论文摘要