Design automation of microfluidic single and double emulsion droplets with machine learning.
Nature communications(2024)
摘要
Droplet microfluidics enables kHz screening of picoliter samples at a fraction of the cost of other high-throughput approaches. However, generating stable droplets with desired characteristics typically requires labor-intensive empirical optimization of device designs and flow conditions that limit adoption to specialist labs. Here, we compile a comprehensive droplet dataset and use it to train machine learning models capable of accurately predicting device geometries and flow conditions required to generate stable aqueous-in-oil and oil-in-aqueous single and double emulsions from 15 to 250 μm at rates up to 12000 Hz for different fluids commonly used in life sciences. Blind predictions by our models for as-yet-unseen fluids, geometries, and device materials yield accurate results, establishing their generalizability. Finally, we generate an easy-to-use design automation tool that yield droplets within 3 μm (<8%) of the desired diameter, facilitating tailored droplet-based platforms and accelerating their utility in life sciences.
更多查看译文
AI 理解论文
溯源树
样例
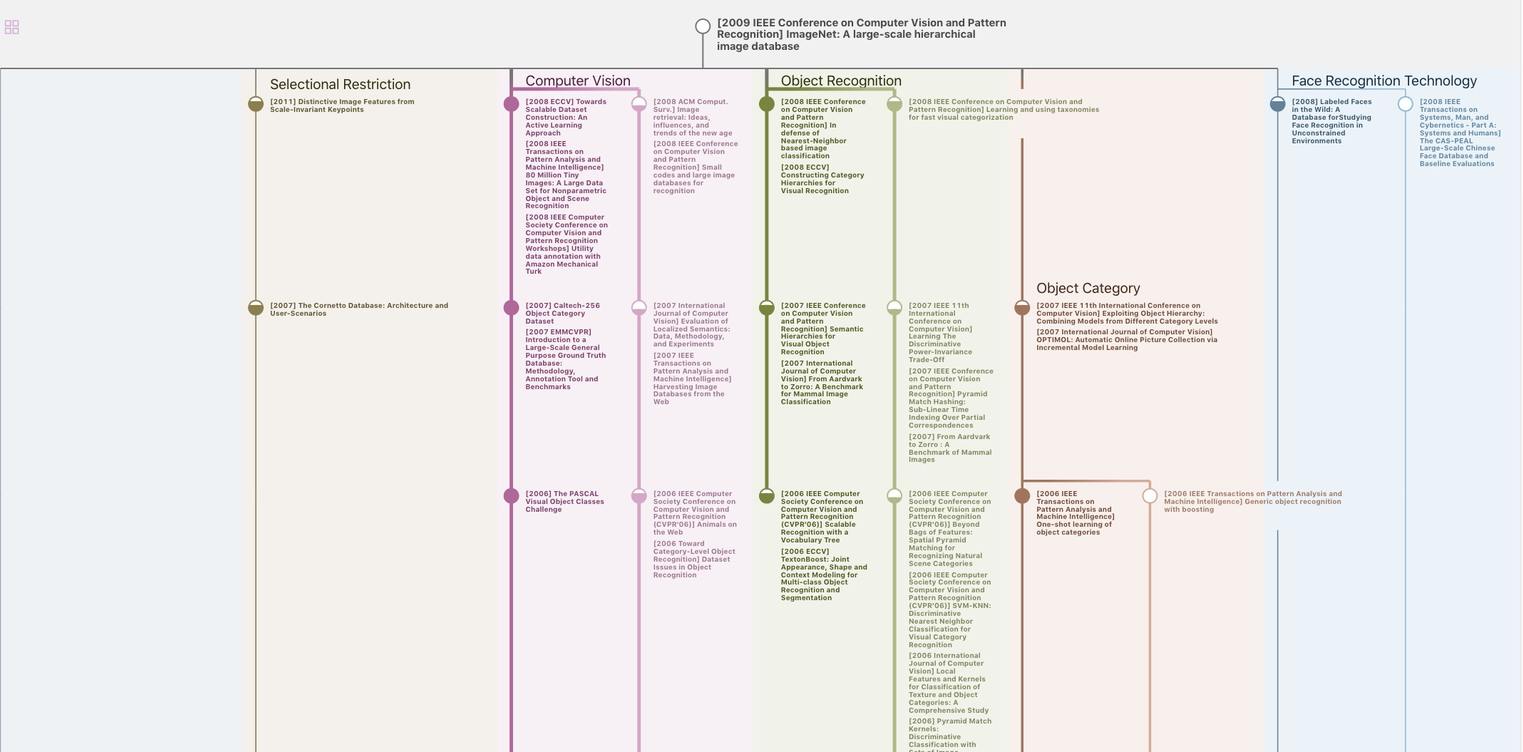
生成溯源树,研究论文发展脉络
Chat Paper
正在生成论文摘要