DraftFed: A Draft-Based Personalized Federated Learning Approach for Heterogeneous Convolutional Neural Networks.
IEEE Trans. Mob. Comput.(2024)
摘要
In conventional federated learning, each device is restricted to train a network model of a same structure. This greatly hinders the application of federated learning in edge devices and IoT scenarios where the data and devices are quite heterogeneous because of their different hardware equipment and communication networks. At the same time, most of the existing studies about federated learning of heterogeneous models are limited to horizontal heterogeneity which share a highly homogeneous vertical structure. Little work has been done on vertical heterogeneity such as models with different number of functional layers or different connection methods within them, not to mention the integrated heterogeneity scenarios. In DraftFed, a novel draft-based approach is proposed to implement personalized federated learning for integrated heterogeneous models. Unlike traditional federated learning in which the parameters/gradients are exchanged, DraftFed uses drafts as key knowledge to guide mutual learning of models, which makes it suitable for model structure personalization application scenarios..
更多查看译文
关键词
Distributed networks,heterogeneous (hybrid) systemsarticle,neural models,personal computing
AI 理解论文
溯源树
样例
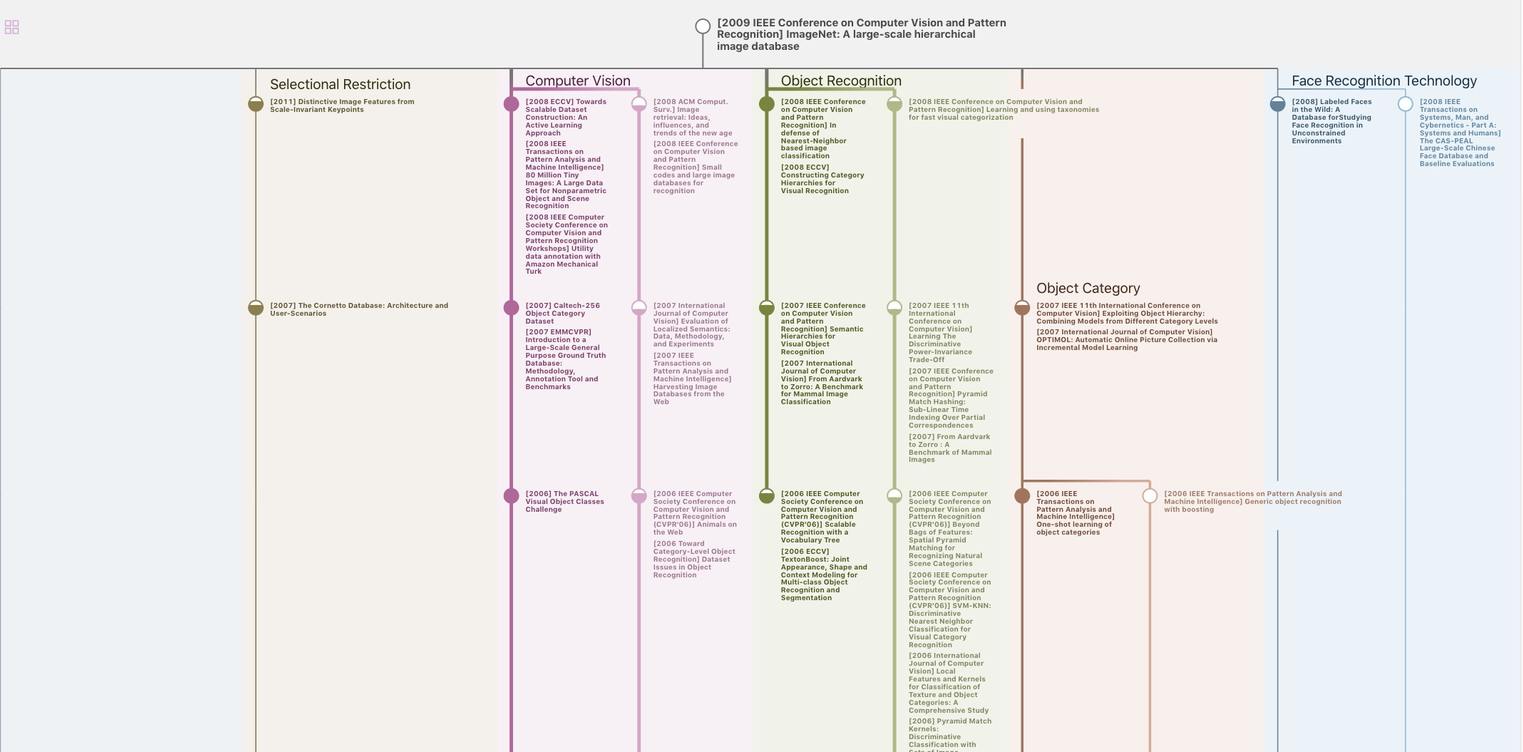
生成溯源树,研究论文发展脉络
Chat Paper
正在生成论文摘要