Predicting HER2 expression status in patients with gastric cancer using 18F-FDG PET/CT radiomics
Research Square (Research Square)(2023)
摘要
Abstract Background Immunohistochemistry (IHC) is the main method used to detect human epidermal growth factor receptor 2 (HER2) expression levels. However, IHC is invasive and cannot dynamically reflect HER2 expression status. The aim of this study was to construct and verify three types of radiomics models based on 18 F-FDG PET/CT imaging and to evaluate the predictive ability of radiomics models for the expression status of HER2 in patients with gastric cancer (GC). Methods A total of 118 patients with GC who underwent 18 F-FDG PET/CT imaging before treatment were enrolled in this study. The LIFEx software package was applied to extract PET and CT radiomics features. The minimum absolute contraction and selection operator (LASSO) algorithm was employed to select the best radiomics features. Three machine learning methods, logistic regression (LR), support vector machine (SVM), and random forest (RF) models, were constructed and verified. The Synthetic Minority Oversampling Technique (SMOTE) was applied to address data imbalance. Results In the training and test sets, the area under the curve (AUC) values of the LR, SVM, and RF models were 0.809 and 0.761, 0.861 and 0.628, and 0.993 and 0.717, respectively, and the Brier scores were 0.118, 0.214, and 0.143, respectively. Among the three models, the LR model exhibited the best prediction performance. The AUC values of the three models significantly improved after SMOTE balanced the data. Conclusion 18 F-FDG PET/CT-based radiomics models demonstrated good performance in predicting HER2 expression status in patients with GC and can be used to preselect patients who may benefit from HER2-targeted therapy.
更多查看译文
关键词
gastric cancer,her2 expression status,f-fdg
AI 理解论文
溯源树
样例
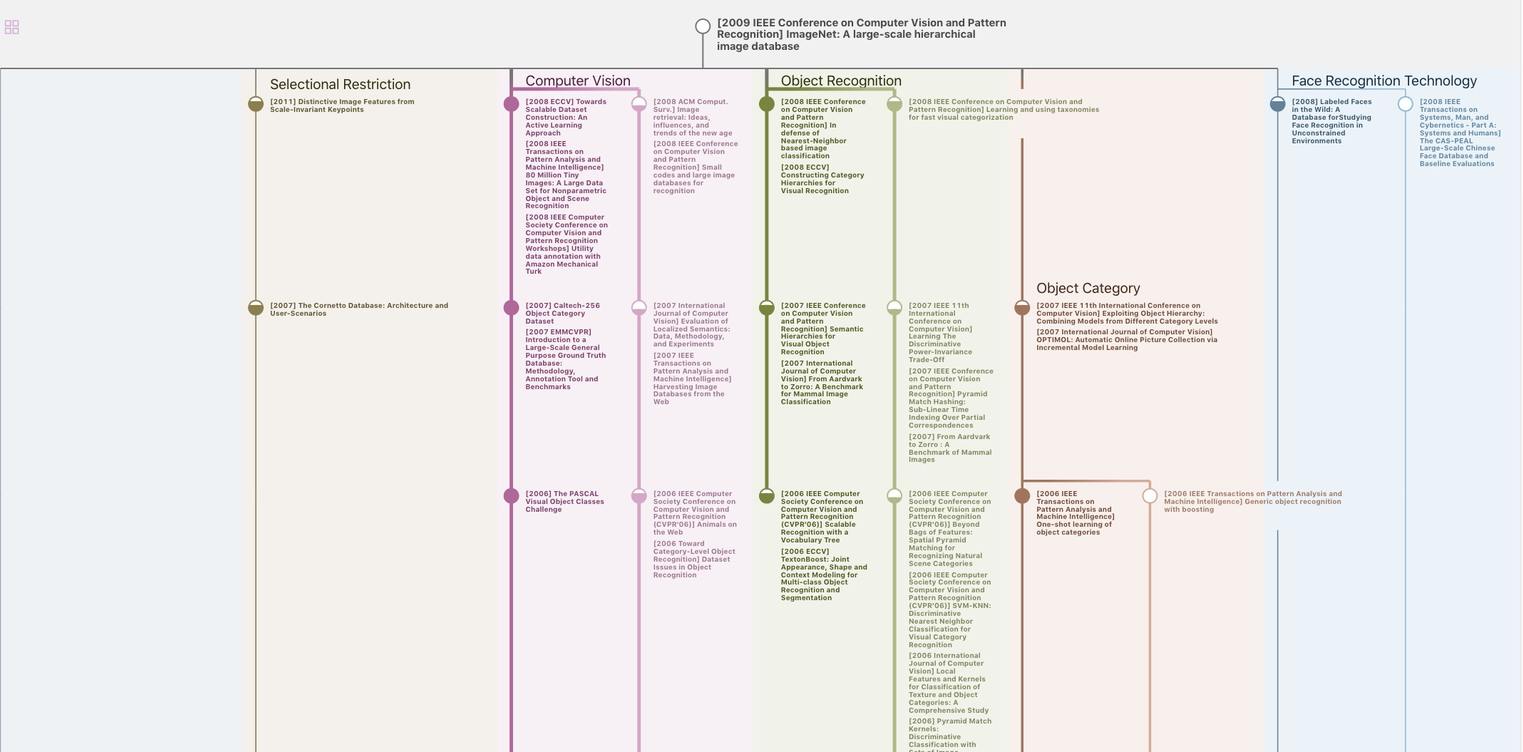
生成溯源树,研究论文发展脉络
Chat Paper
正在生成论文摘要