Editorial: 2021 Best Paper Awards
Vehicular Communications(2023)
摘要
The high need of real-time vehicular applications for self-driving cars to maintain service availability and reachability, and to process huge amount of generated data within a small amount of time, rise the need to improve the vehicular network infrastructure. Fog computing has been introduced to reduce the amount of data sent to cloud by bringing processing power near the edge and reducing latency. In this paper, we overcome the aforementioned limitations by taking advantage of Istio service mesh and kubeadm clustering to handle inter-service communication and provide clusters to serve nearby vehicles, the evolvement of On-Board Units in vehicle supplies enough processing power to run reinforcement learning model in taking action of pushing microservices to cluster, and the efficiency of Hungarian Algorithm to assign microservices to vehicles. We propose in our scheme (1) a service mesh architecture that manages communication between multiple microservices across different clusters and tackle inter-service communication, (2) a Reinforcement Learning model deployed on road side units to predict on-demand placement of microservices on cluster or caching at RSU, and (3) Reformulating the vehicular container placement (VCP) problem as an assignment problem to map microservices to vehicles. Experiments and simulations show that the reinforcement learning model served 90% of requests when deploying microservices which is more efficient than current strategies in the literature. Given that only needed microservices are deployed in limited resource cluster, mesh network shows an improvement of 84% in the deployment time and inter-service communication across clusters, and the usage of Hungarian algorithm in solving the VCP improves Quality of Service (QoS).
更多查看译文
关键词
editorial,paper
AI 理解论文
溯源树
样例
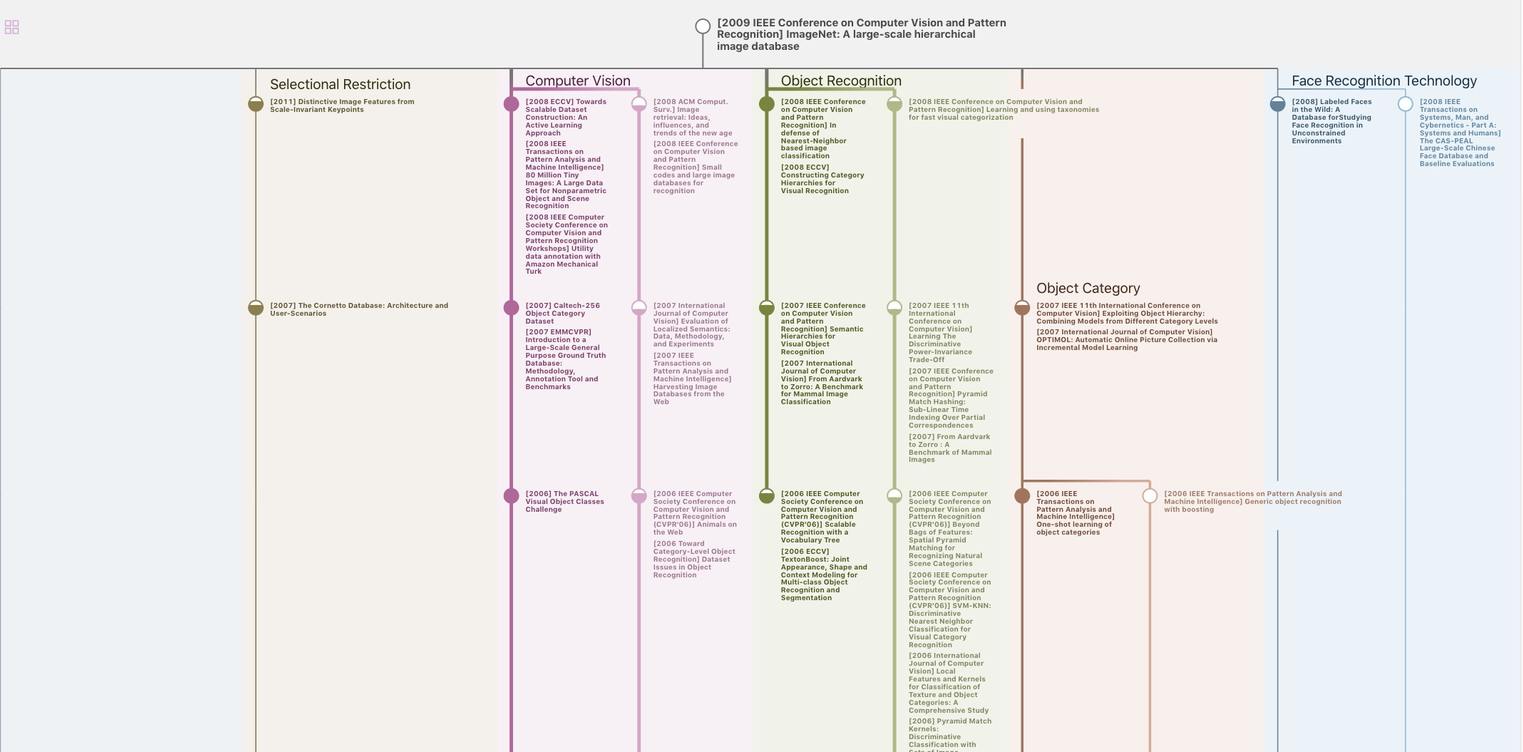
生成溯源树,研究论文发展脉络
Chat Paper
正在生成论文摘要