AJENet: Adaptive Joints Enhancement Network for Abnormal Behavior Detection in Office Scenario
IEEE TRANSACTIONS ON CIRCUITS AND SYSTEMS FOR VIDEO TECHNOLOGY(2024)
摘要
With the increasing popularity of intelligent surveillance systems, abnormal behavior detection of human beings based on computer vision is attracting more attention. It aims to classify and locate the abnormal behaviors and coordinates of human beings, respectively, and is a fundamental technology for intelligent security. Existing approaches mainly focus on exploring abnormal behavior features through object detectors. However, in office scenarios, almost all abnormal behaviors are closely associated with the fine-grained feature around the nose, wrist, elbow, and other human joint points regions. Detectors for generic objects cannot adequately capture such differences between abnormal behaviors, resulting in sub-optimal performance. In this paper, we focus on human joints and take one step further to enable effective behavior characteristics learning in office scenarios. In particular, we propose a novel Adaptive Joints Enhancement Network (AJENet), which includes two closely-related components, Joints Predict block (JP) and Adaptive Key Joints Enhancement block (AKJE). JP block is used to predict the human joints and facilitates the feature learning around them implicitly. By inputting the features around joints, the AKJE block enhances the feature representations of key joints according to the abnormal behavior characteristics adaptively. Experimental results demonstrate that our method outperforms other state-of-the-art methods on the collected real office scenario Office Behavior Dataset. Besides, to verify the generalization capabilities and potential of AJENet, we construct comparisons on another generic dataset PASCAL VOC 2012 Action.
更多查看译文
关键词
Abnormal behavior detection,object detection,joint points,feature enhancement
AI 理解论文
溯源树
样例
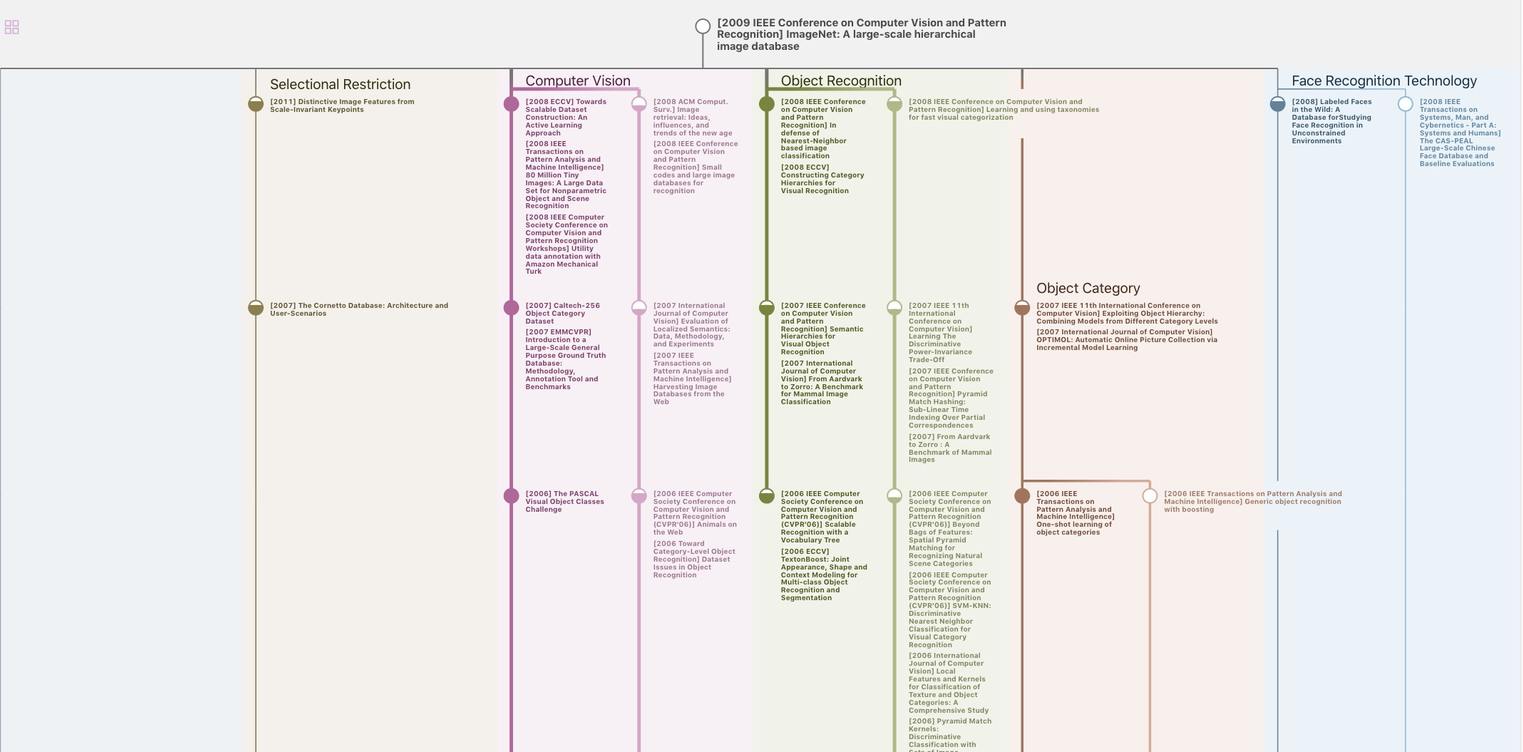
生成溯源树,研究论文发展脉络
Chat Paper
正在生成论文摘要