Strength predictive models of cementitious matrix by hybrid intrusion of nano and micro silica: Hyper-tuning with ensemble approaches
JOURNAL OF MATERIALS RESEARCH AND TECHNOLOGY-JMR&T(2023)
摘要
The incorporation of nanomaterials (NMs) in concrete will produce the utmost properties. Nevertheless, investigational work takes a great deal of time with efforts to measure the strength of concrete. Thus, the process of estimating the properties of cement paste can be accelerated by employing soft machine learning (SML) techniques. Individual machine learning approaches (MLAs) like random forest (RF), decision tree (DT), and support vector machine (SVM) were utilized. These individual MLAs models then ensemble with two approaches namely bagging and boosting. The outcome of the model depicts a robust performance by showing significant correlations (R2) as compared to individual MLAs. Data points with 205 having contribution factors (e.g., curing time (days), water to binder (w/b), micro-silica (%), and nano-silica (%) were used as input parameters, and compressive strength as output for MLAs modeling. To ensure that each model is as accurate as possible, cross-validation with K-folding and statistical error analysis (i.e., MAE, MAE, and RMSE) were utilized. Thus, interpretable approaches with AdaBoost give a strong correlation of R2 ranging from 0.85 to 0.90 respectively with fewer errors. Out of which DT with ensemble Adaboost and RF give a robust performance. Moreover, the results of the crossvalidation show that the response was accurate, with fewer errors. Therefore, the ensemble model represents a robust correlation. Therefore, its usage with individual ML would enhance the model's performance. In addition, the graphical user interface (GUI) is created by training a model that can predict the values of the desired outputs when the necessary input parameters are provided. It simplifies the procedure and provides a handy tool for employing the model's capabilities in the civil engineering domain. (c) 2023 The Author(s). Published by Elsevier B.V. This is an open access article under the CC BY-NC-ND license (http://creativecommons.org/licenses/by-nc-nd/4.0/).
更多查看译文
关键词
Nano-silica,Micro-silica,Machine learning algorithms,Cross-validation,Statistical analysis
AI 理解论文
溯源树
样例
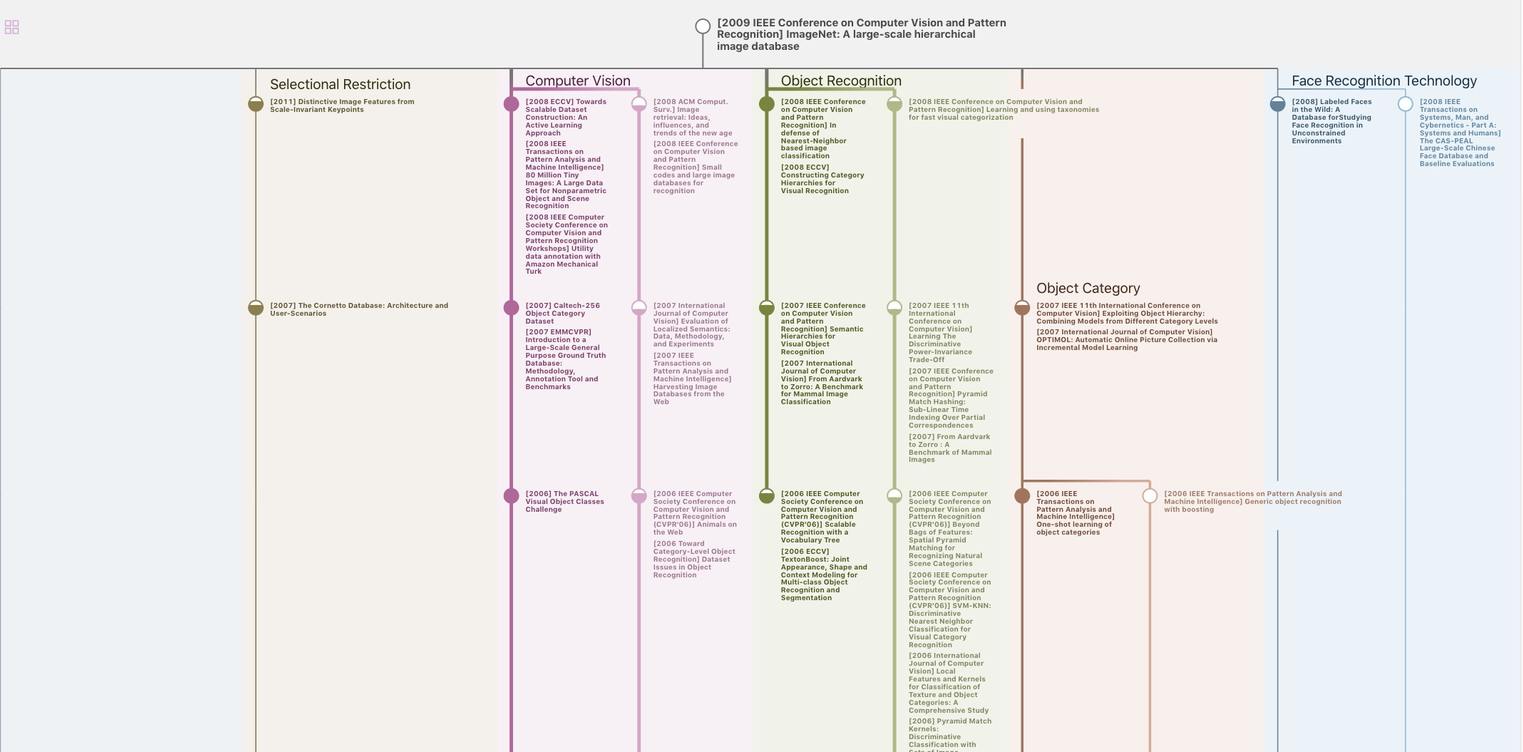
生成溯源树,研究论文发展脉络
Chat Paper
正在生成论文摘要