DSFFNet: Tire Defect Detection Based on Deep and Shallow Feature Fusion
Research Square (Research Square)(2023)
摘要
Abstract Recently, object detection based on deep learning has made great progress in the field of defect detection. Due to its complex texture background and varied defect features, existing defect detection methods based on object detection are face great challenges for the detection of tire internal defects. In this paper, a tire defect detection model based on deep and shallow feature fusion is proposed. Firstly, a multi-head feature extraction module is proposed, which extract abundant effective information from multiple dimensions. Secondly, a spatial semantic fusion upsampling module is proposed to alleviate the problem of information loss in the upsampling process. Finally, a novel prediction head is designed to expand the receptive field by compressing the size of the feature map to improve the detection accuracy of large defects. Experimental results show that the mAP of the proposed method achieves 94.03% on the tire internal defect dataset, the average detection time is 36.74ms, which can meet the needs of industrial online detection.
更多查看译文
关键词
tire defect detection,shallow feature fusion,dsffnet
AI 理解论文
溯源树
样例
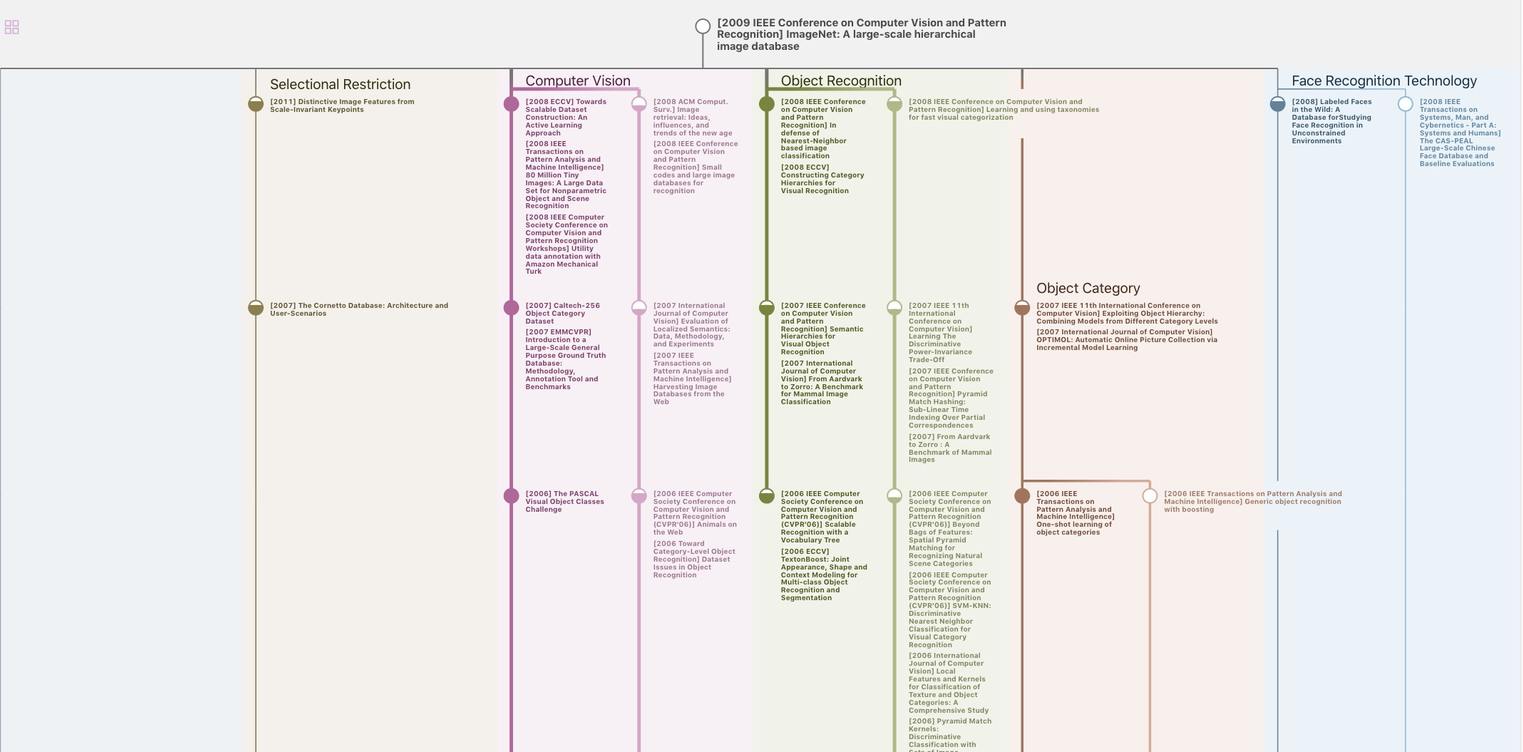
生成溯源树,研究论文发展脉络
Chat Paper
正在生成论文摘要