Attention-Based Highway Safety Planner for Autonomous Driving via Deep Reinforcement Learning
IEEE TRANSACTIONS ON VEHICULAR TECHNOLOGY(2024)
摘要
In this article, a motion planning for autonomous driving on highway is studied. A high-level motion planning controller with discrete action space is designed based on deep Q network (DQN). An occupancy grid based state presentation aiming at specific scenarios is proposed and then a novel attention mechanism named external spatial attention (ESA) is designed for occupancy grid to improve the network performance. Considering both computational complexity and interpretability, a lightweight data-driven safety layer consisting of two-dimensional linear biased support vector machine (2D-LBSVM) is proposed to improve safety. The advantages of this controller and the role of each module are illustrated by experiments. In addition, the superior performance of occupancy grid state and the interpretability of safety layer are further analyzed.
更多查看译文
关键词
Safety,Planning,Autonomous vehicles,Training,Deep learning,Vehicle dynamics,Trajectory,deep reinforcement learning,attention,safety layer
AI 理解论文
溯源树
样例
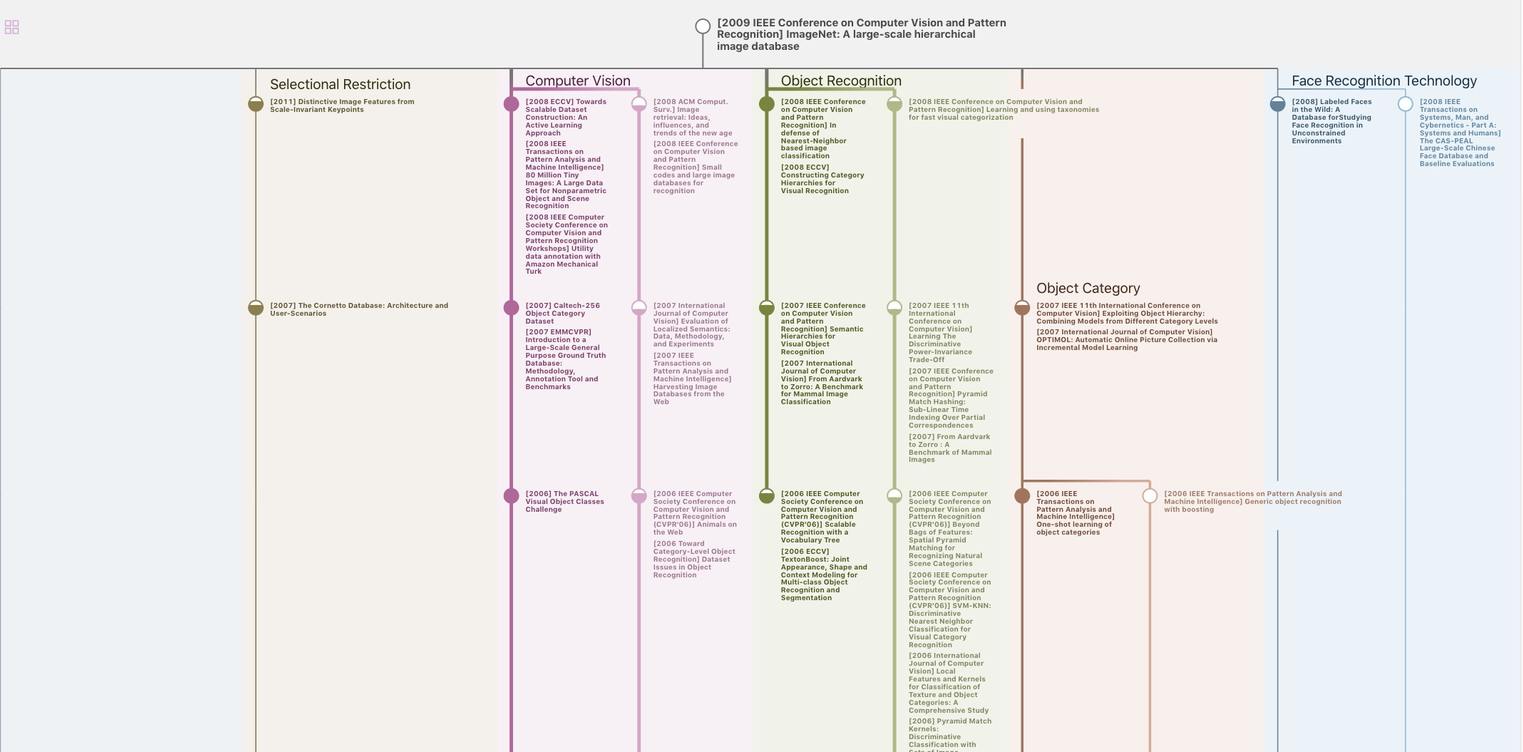
生成溯源树,研究论文发展脉络
Chat Paper
正在生成论文摘要