S235: generation and application of a living t-cell lymphoma patient-derived tumor xenograft biorepository
HemaSphere(2023)
摘要
Topic: 20. Lymphoma Biology & Translational Research Background: T-cell lymphoma (TCL) patients often experience dismal therapeutic responses and poor survival. This is partially due to the absence of known targetable liabilities in many TCL entities. The lack of informative models has hindered improvements. Patient-Derived Tumor-Xenografts (PDX) represent a powerful platform to recapitulate many of the biological features of human cancers. Aims: We constructed a large TCL PDX library to identify novel vulnerabilities and foster new patient-tailored approaches. Methods: We implanted human TCL samples in NSG immunodeficient mice and performed phenotypic and genotypic studies. We executed in-vitro functional experiments, drug screenings using PDX-derived tumor cells, and in-vivo pre-clinical trials. Results: We implanted 326 TCL samples, resulting in the generation of 91 PDX models representative of the most common TCL entities (Fig.1A). Each PDX resembled its corresponding human sample as shown by flow cytometry, immune-histochemistry and T-cell receptor (TCR) gene rearrangement. We used RNAseq to prove a faithful correspondence of human TCL signatures in PDX (Fig 1B) and identified both known fusions (e.g., ALK fusions, DNMT3A-ASXL2, TBL1XR1/TP63) and novel ones (i.e., ACADVL-VAV1, MAZ-NF1, VASP-PPP2R1A, TET3-IMMT). Downstream inhibition of MAZ-NF1 chimeric transcript in an ALK- ALCL PDX using MEK-inhibitor AZD6244 produced a delay in tumor growth in-vivo. Then, we performed whole-exome sequencing (WES) and copy number alteration (CNA) profiling (Fig. 1C). We identified driver mutations and CNAs affecting various pathways among different TCL entities. Clonal evolution analysis showed distinct mutation hierarchies, with ancestor mutations occurring in genes such as STAT3, TP53, IDH2, and KMT2C, followed by JAK1, CDK11B, TET2, and RHOA. Major clones were maintained from primary to PDX, while minor subclones branched and expanded along propagation. Furthermore, we adopted a high-throughput in-vitro drug screening approach targeting ~634 proteins and observed high reproducibility along serial propagations and different response profiles based on tumor identity. Finally, we trained a predictive model based on RNAseq data and viability upon drug exposure and found gene sets predicting response to specific compounds (i.e., ruxolitinib and belinostat). Ultimately, we performed in-vivo pre-clinical trials in 12 PDXs of different TCL subtypes (Fig. 1D) and showed close correspondence with patients’ responses to various drugs, including chemotherapy (CHOP) as well as targeted agents (ALK-inhibitor crizotinib, JAK-inhibitor ruxolitinib, CD30-drug conjugate brentuximab-vedotin). In addition, we tested novel compounds emerging from the drug screenings including JAK/SYK inhibitor cerdulatinib and CDK9 inhibitor AZD4573: mice treated with the combination of the two drugs showed significantly better progression-free survival compared to single agents. Ultimately, we generated a transcriptome-based predictive signature of the response and found that non-responder models had a higher expression for genes linked to TCR signaling, while responders were enriched in genes regulating migration, cytoskeleton, and cell interactions. Summary/Conclusion: We generated the largest TCL PDX bio-repository accounting for >90 models of different subtypes. PDXs mimicked donor lymphomas phenotypically and genotypically. We discovered novel driver fusions, oncogenic mutations, clonal evolution patterns, innovative therapeutics/combinations and response predictors. We anticipate that this library will foster the discovery of new liabilities and therapeutics in TCL patients.Keywords: T cell lymphoma, Clonal expansion, Experimental therapeutics, Murine models
更多查看译文
关键词
t-cell,patient-derived
AI 理解论文
溯源树
样例
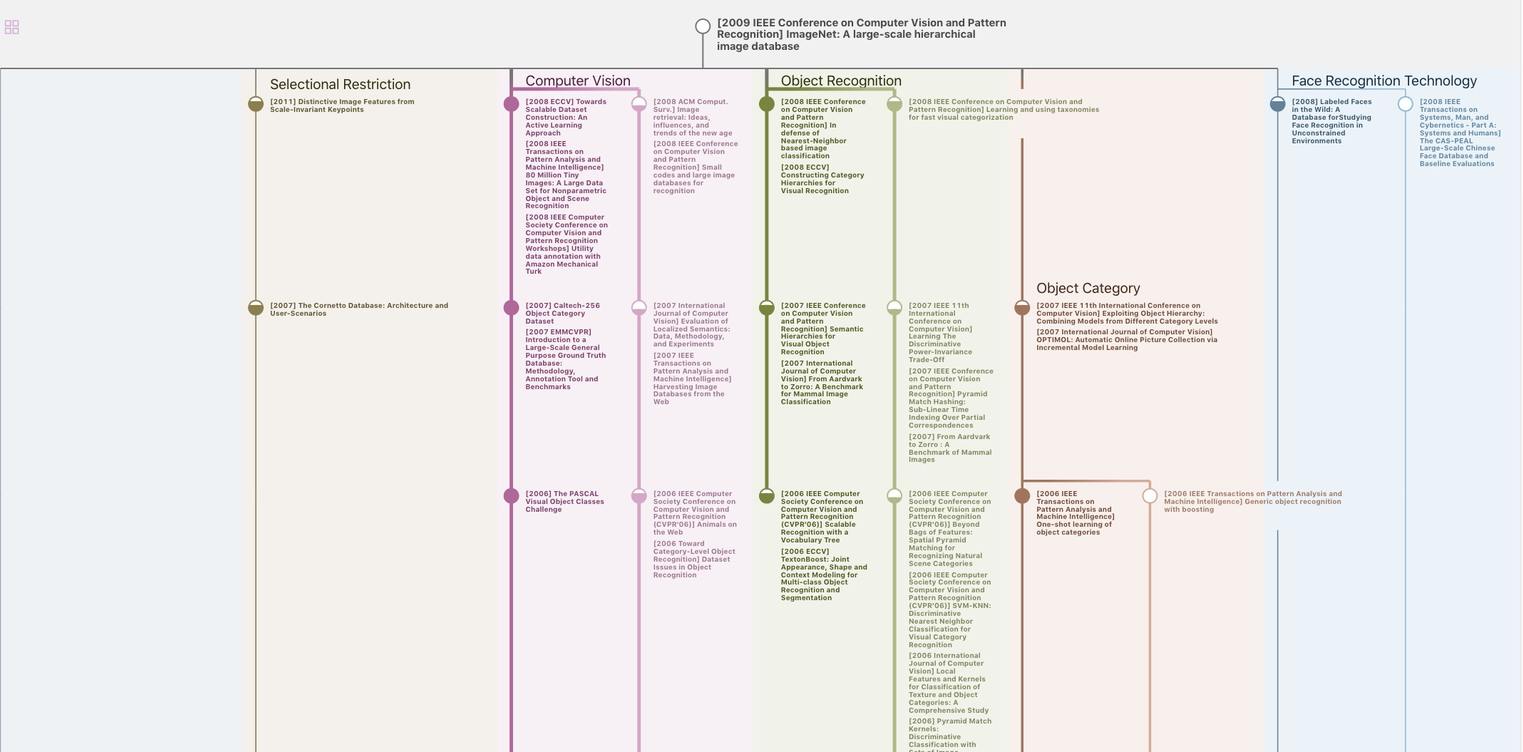
生成溯源树,研究论文发展脉络
Chat Paper
正在生成论文摘要