Accelerated PALM for Nonconvex Low-Rank Matrix Recovery with Theoretical Analysis.
IEEE transactions on circuits and systems for video technology(2024)
摘要
Low-rank matrix recovery is a major challenge in machine learning and computer vision, particularly for large-scale data matrices, as popular methods involving nuclear norm and singular value decomposition (SVD) are associated with high computational costs and biased estimators. To overcome this challenge, we propose a novel approach to learning low-rank matrices based on the matrix volume and a nonconvex logarithmic function. The matrix volume is the product of all the nonzero singular values of a matrix and has unique geometric properties and connections with other convex and nonconvex functions. We establish a generalized nonconvex regularization problem using the penalty function strategy and introduce an accelerated proximal alternating linearized minimization (AccPALM) algorithm with double acceleration, which combines Nesterov’s acceleration and power strategy. The algorithm reduces computational costs and has provable convergence results under the Kurdyka-Łojasiewicz (KŁ) inequality with mild conditions. Our approach shows superior accuracy, efficiency, and convergence behavior compared to other low-rank matrix learning methods on robust matrix completion (RMC) and low-rank representation (LRR) tasks. We analyze the impact of algorithm parameters on convergence and performance and present visually appealing results to further demonstrate the effectiveness of our approach. The proposed methodology represents a promising advance in the field of low-rank matrix recovery, and its effectiveness has been validated via extensive numerical experiments. The source code for the proposed algorithms is accessible at https://github.com/ZhangHengMin/AccPALMcodes.
更多查看译文
关键词
Linear matrix inequalities,Minimization,Optimization,Matrix decomposition,Computational modeling,Convergence,Sparse matrices,Nonconvex rank relaxations,matrix volume,proximal alternating linearized minimization,robust matrix completion,low-rank representation
AI 理解论文
溯源树
样例
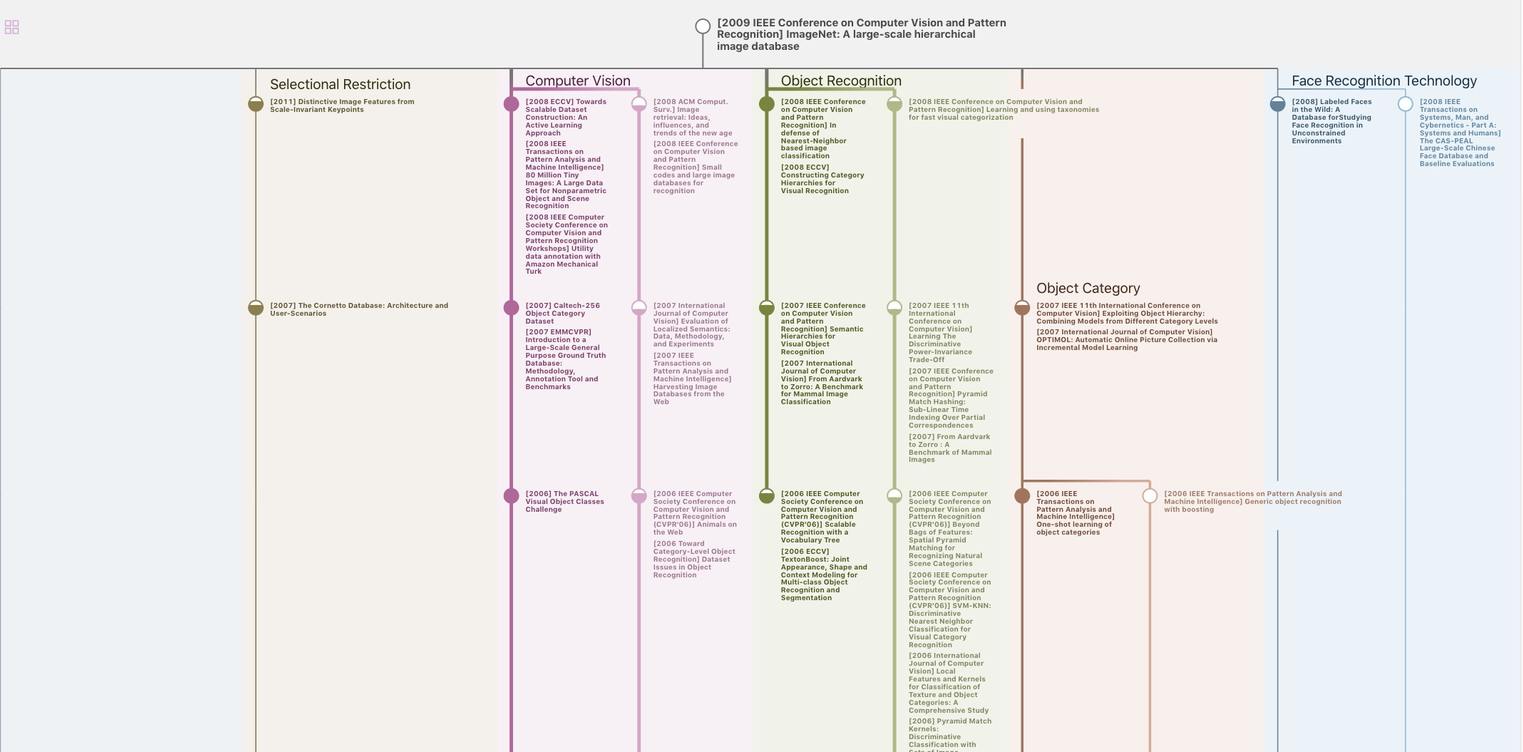
生成溯源树,研究论文发展脉络
Chat Paper
正在生成论文摘要