High dimensional predictions of suicide risk in 4.2 million US Veterans using ensemble transfer learning
Scientific Reports(2024)
摘要
We present an ensemble transfer learning method to predict suicide from Veterans Affairs (VA) electronic medical records (EMR). A diverse set of base models was trained to predict a binary outcome constructed from reported suicide, suicide attempt, and overdose diagnoses with varying choices of study design and prediction methodology. Each model used twenty cross-sectional and 190 longitudinal variables observed in eight time intervals covering 7.5 years prior to the time of prediction. Ensembles of seven base models were created and fine-tuned with ten variables expected to change with study design and outcome definition in order to predict suicide and combined outcome in a prospective cohort. The ensemble models achieved c-statistics of 0.73 on 2-year suicide risk and 0.83 on the combined outcome when predicting on a prospective cohort of ∼ 4.2 M veterans. The ensembles rely on nonlinear base models trained using a matched retrospective nested case-control (Rcc) study cohort and show good calibration across a diversity of subgroups, including risk strata, age, sex, race, and level of healthcare utilization. In addition, a linear Rcc base model provided a rich set of biological predictors, including indicators of suicide, substance use disorder, mental health diagnoses and treatments, hypoxia and vascular damage, and demographics.
更多查看译文
AI 理解论文
溯源树
样例
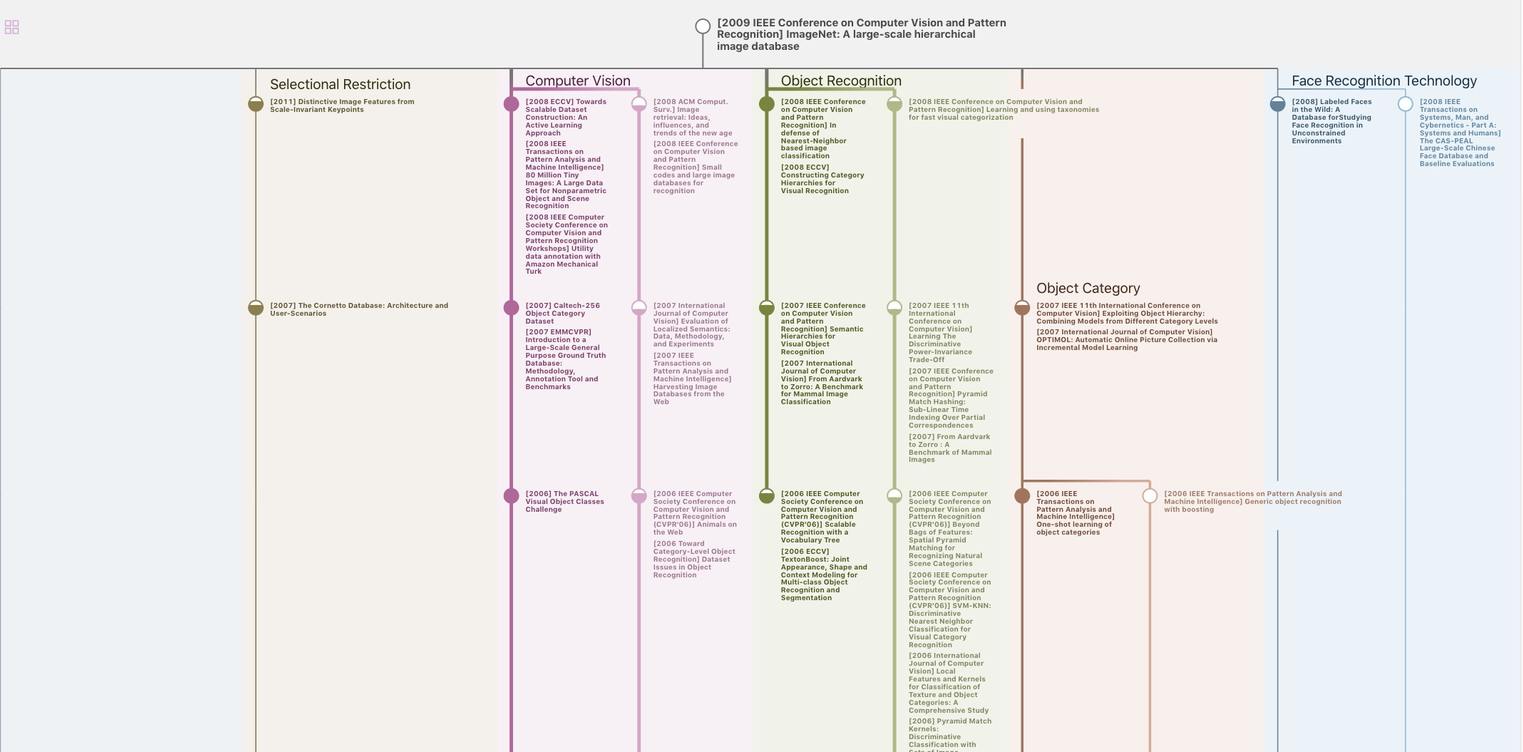
生成溯源树,研究论文发展脉络
Chat Paper
正在生成论文摘要