DeepGEEP: Data-Driven Prediction of Bacterial Biofilm Gene Expression Profiles
bioRxiv (Cold Spring Harbor Laboratory)(2023)
摘要
Abstract Predicting the gene expression profile (GEEP) of bacterial biofilms in response to spatial, temporal, and concentration profiles of stimulus molecules holds significant potential across microbiology, biotechnology, and synthetic biology domains. However, the resource and time-intensive nature of experiments within Petri dishes presents significant challenges. Data-driven methods offer a promising avenue to replace or reduce such experiments given sufficient data. Through wellcrafted data generation techniques, the data scarcity issue can be effectively addressed. In this paper, an innovative methodology is presented for generating GEEP data over Petri dish resulting from a specific chemical stimulus release profile. We subsequently introduce a two-dimensional convolutional neural network (2DCNN) architecture to leverage the synthesized dataset to predict GEEP variations across bacterial biofilms within the Petri dish. Our approach, known as DeepGEEP, is applied to data generated by a particle-based simulator (PBS), affording us the flexibility to evaluate its efficacy. Our proposed method attains a significant level of accuracy in comparison to established benchmark models such as Linear SVM, Radial Basis Function SVM, Decision Tree, and Random Forest.
更多查看译文
关键词
bacterial,gene expression,prediction,data-driven
AI 理解论文
溯源树
样例
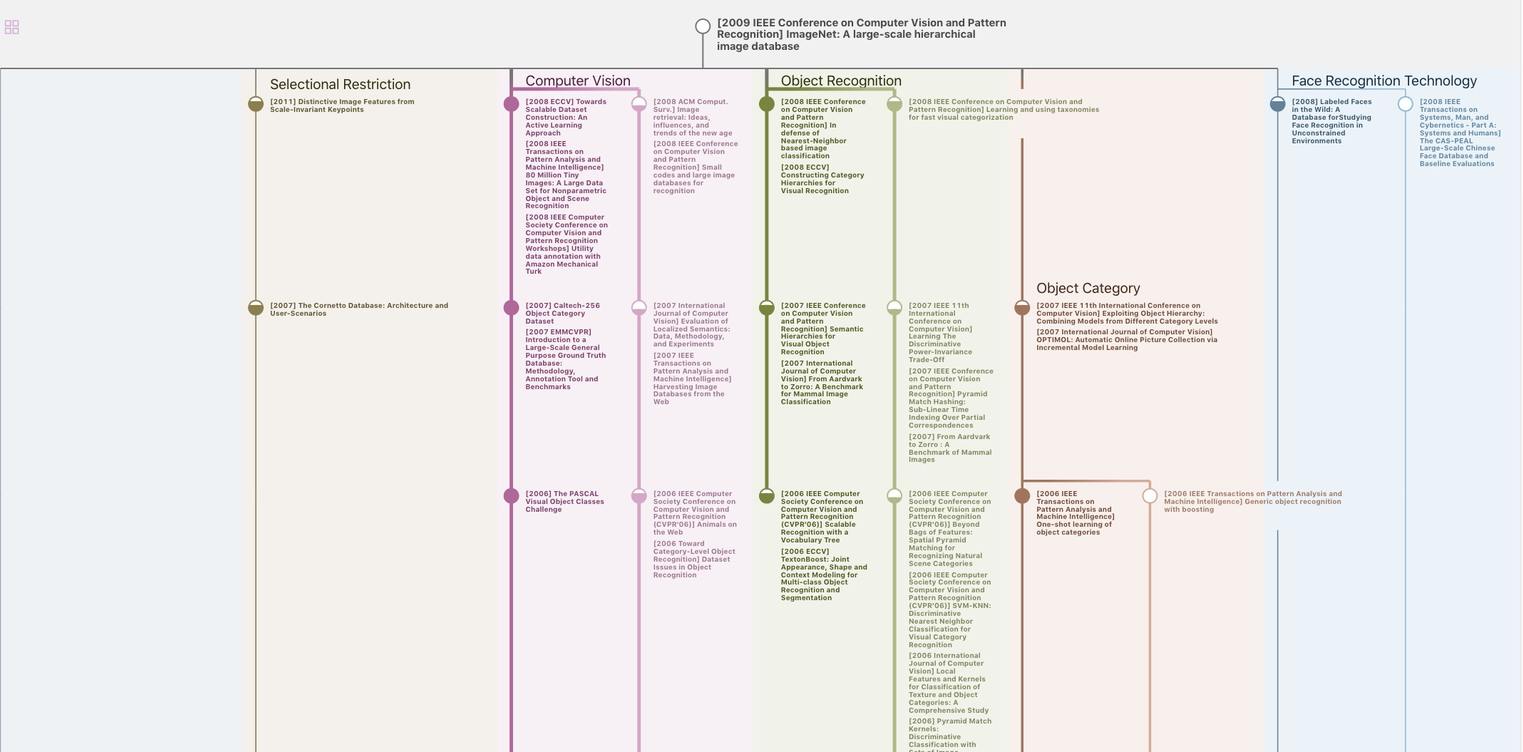
生成溯源树,研究论文发展脉络
Chat Paper
正在生成论文摘要