Optical Remote Sensing Ship Recognition and Classification Based on Improved YOLOv5
REMOTE SENSING(2023)
摘要
Due to the special characteristics of the shooting distance and angle of remote sensing satellites, the pixel area of ship targets is small, and the feature expression is insufficient, which leads to unsatisfactory ship detection performance and even situations such as missed and false detection. To solve these problems, this paper proposes an improved-YOLOv5 algorithm mainly including: (1) Add the Convolutional Block Attention Module (CBAM) into the Backbone to enhance the extraction of target-adaptive optimal features; (2) Introduce a cross-layer connection channel and lightweight GSConv structures into the Neck to achieve higher-level multi-scale feature fusion and reduce the number of model parameters; (3) Use the Wise-IoU loss function to calculate the localization loss in the Output, and assign reasonable gradient gains to cope with differences in image quality. In addition, during the preprocessing stage of experimental data, a median+bilateral filter method was used to reduce interference from ripples and waves and highlight the information of ship features. The experimental results show that Improved-YOLOv5 has a significant improvement in recognition accuracy compared to various mainstream target detection algorithms; compared to the original YOLOv5s, the mean Average Precision (mAP) improved by 3.2% and the Frames Per Second (FPN) accelerated by 8.7%.
更多查看译文
关键词
optical remote sensing images,convolutional block attention module,cross-layer connection channel,lightweight GSConv,Wise-IoU loss function,median plus bilateral filter,object detection
AI 理解论文
溯源树
样例
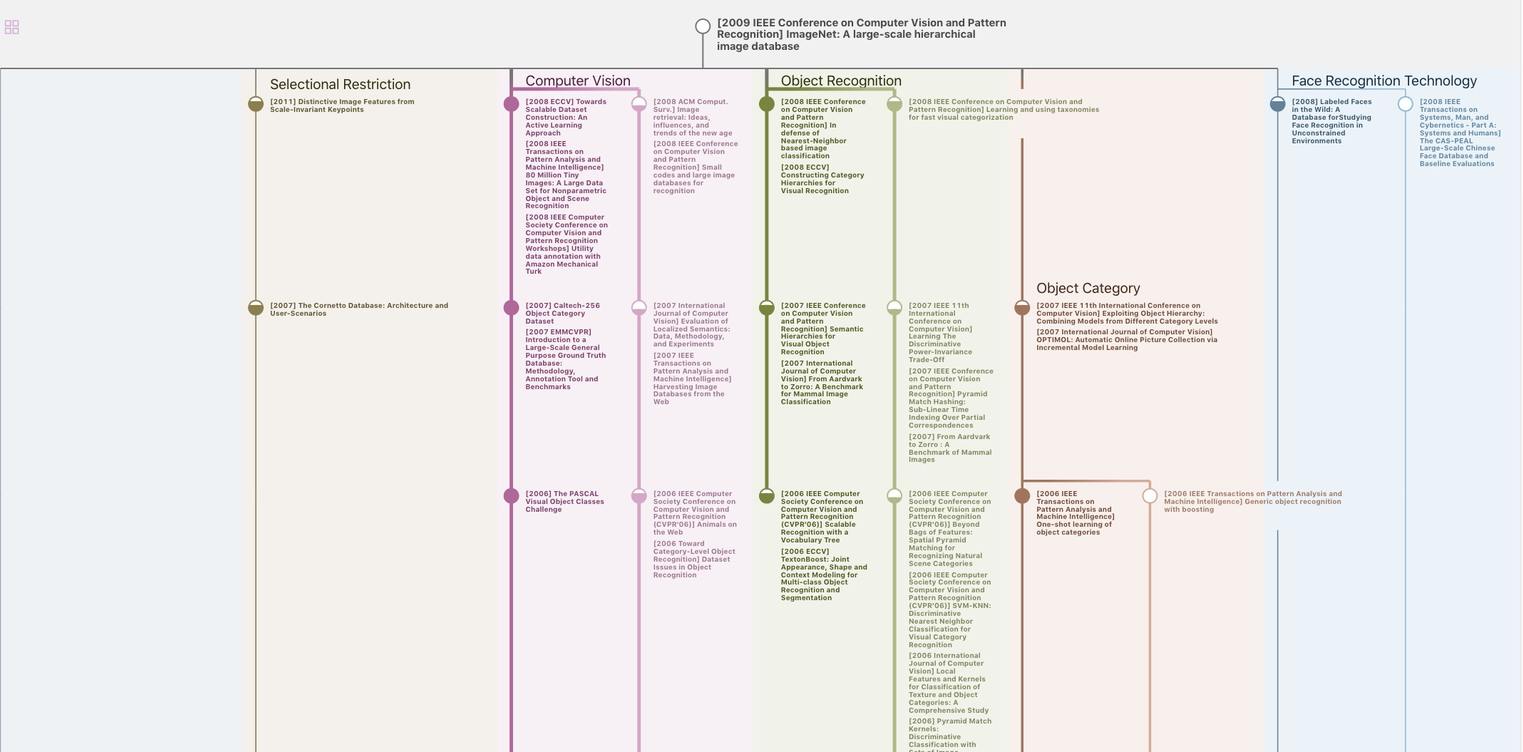
生成溯源树,研究论文发展脉络
Chat Paper
正在生成论文摘要