GroupMO: A Memory-augmented Meta-optimized Model for Group Recommendation
Research Square (Research Square)(2023)
摘要
Abstract Group recommendation aims to suggest desired items for a group of users. Existing methods can achieve inspiring results in predicting the group preferences in data-rich groups. However, they could be ineffective in supporting cold-start groups due to their sparsity interactions, which prevents the model from understanding their intent. Although cold-start groups can be alleviated by meta-learning, we cannot apply it by using the same initialization for all groups due to their varying preferences. To tackle this problem, this paper proposes a memory-augmented meta-optimized model for group recommendation, namely GroupMO. Specifically, we adopt a clustering method to assemble the groups with similar profiles into the same cluster and design a representative group profile memory to guide the preliminary initialization of group embedding network for each group by utilizing those clusters. Besides, we also design a group shared preference memory to guide the prediction network initialization at a more refined granularity level for different groups, so that the shared knowledge can be better transferred to groups with similar preferences. Moreover, we incorporate those two memories to optimize the meta-learning process. Finally, extensive experiments on two real-world datasets demonstrate the superiority of our model.
更多查看译文
关键词
groupmo,recommendation,memory-augmented,meta-optimized
AI 理解论文
溯源树
样例
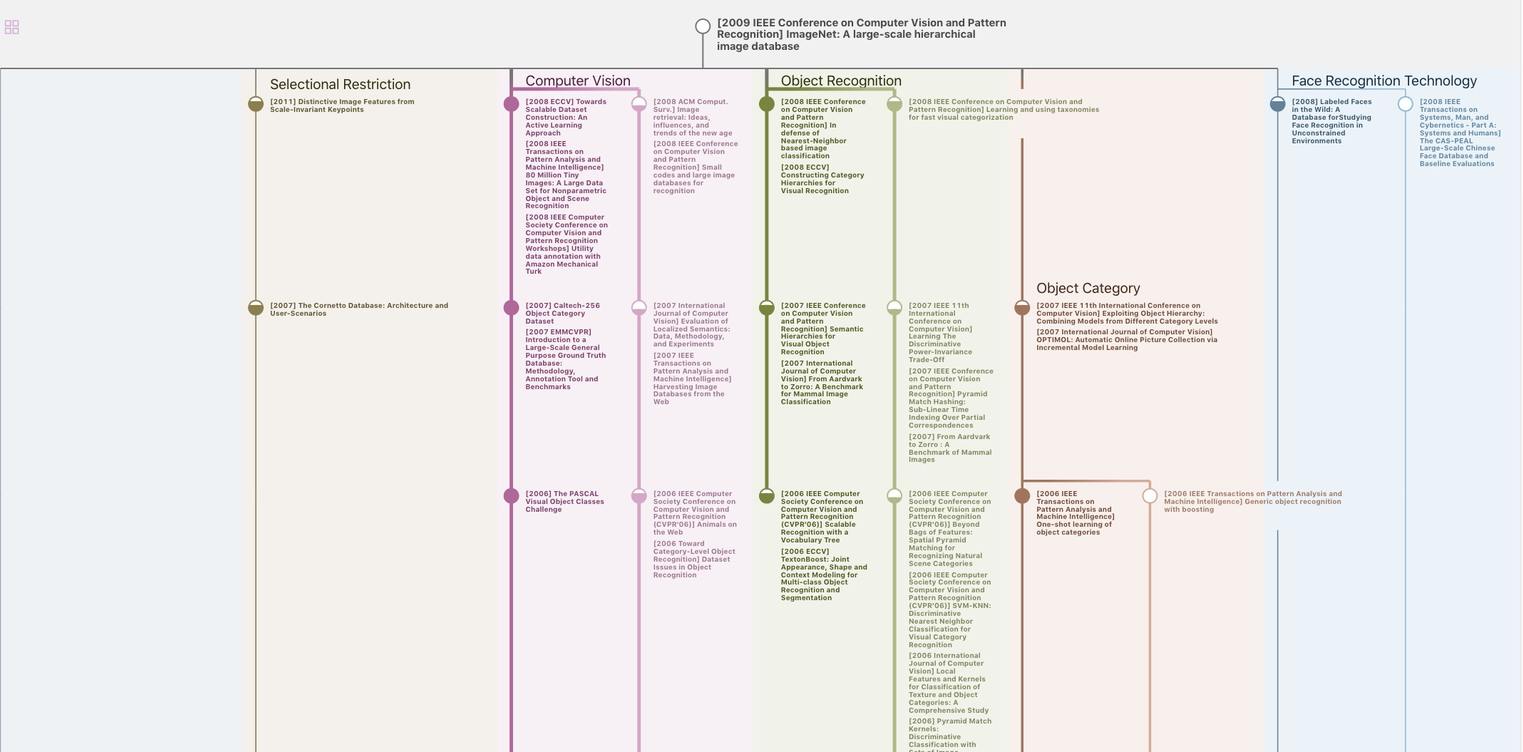
生成溯源树,研究论文发展脉络
Chat Paper
正在生成论文摘要