Heterogeneous Graph Convolutional Network for E-commerce Product Recommendation with Adaptive Denoising Training
IEEE Transactions on Consumer Electronics(2023)
摘要
As shopping styles become more diversified, online shopping has gradually become the mainstream method of shopping. The internet has emerged as a popular platform for consumers to share their ideas, opinions, and experiences related to products and services. To enhance the shopping experience, e-commerce platforms recommend products that may interest consumers. However, the recommendation system currently faces three challenges. (1) Consumers of the recommended products are not interested. (2) The recommendation algorithm is not robust to noisy data. (3) With the increasing complexity of data types, some information has not been fully utilized. To overcome these challenges, we propose a novel recommendation model, named Heterogeneous Graph Convolutional Network Recommendation with Adaptive Denoising Training (HGCRD). We introduce a meta-path-based random walk strategy to generate meaningful node sequences for network embedding. We then use graph convolutional networks (GCNs) to learn the node embeddings for the obtained node networks. To mitigate noise in the dataset, we incorporate an adaptive denoising training (ADT) strategy during training. We conducted extensive experiments on three public datasets, and our model outperformed the competitive baseline by a significant margin.
更多查看译文
关键词
Recommendation system,e-commerce shopping,heterogeneous information networks,adaptive denoising training
AI 理解论文
溯源树
样例
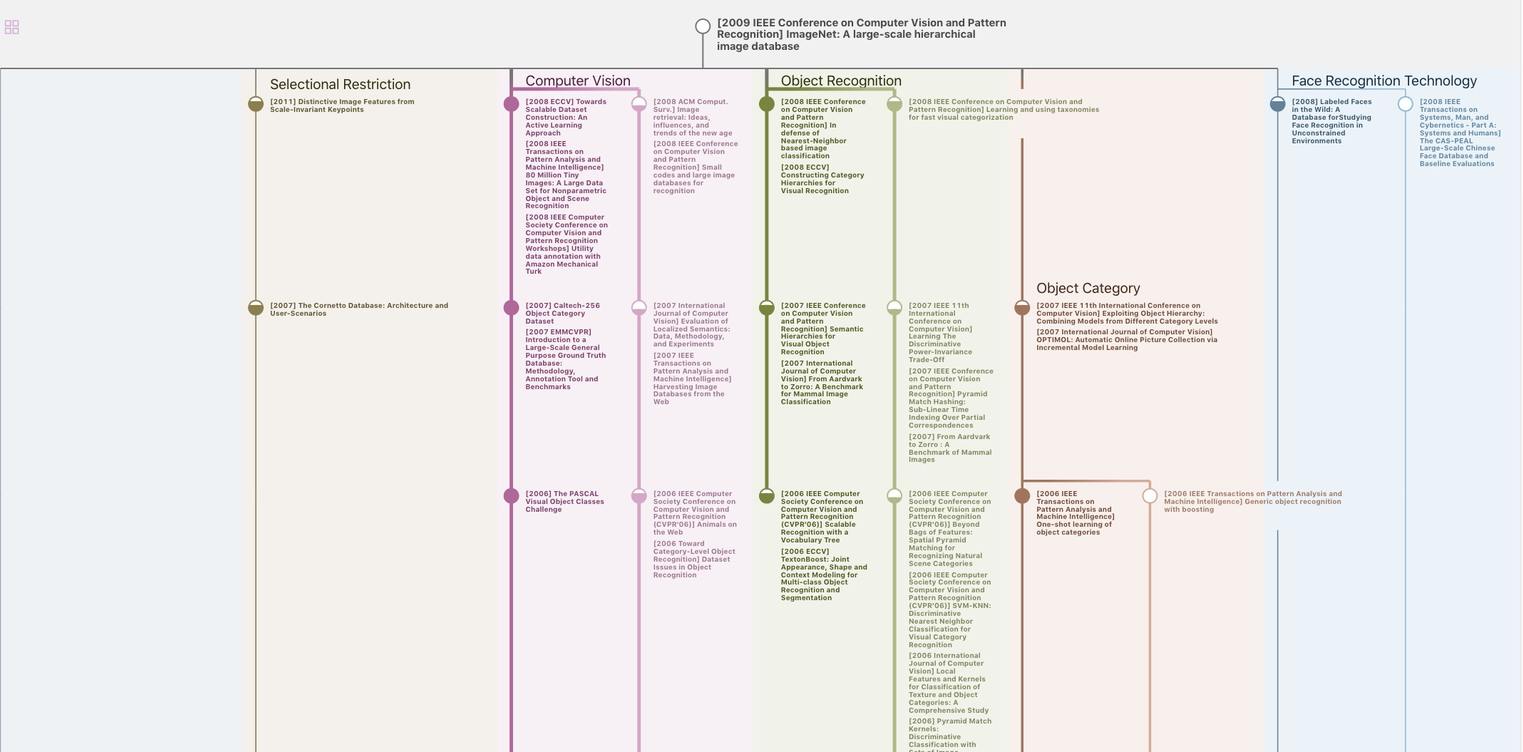
生成溯源树,研究论文发展脉络
Chat Paper
正在生成论文摘要