Gait speed estimation via inertial sensors and machine learning
Gait & Posture(2023)
摘要
Changes in gait are a well-documented symptom for several conditions, such as degenerative diseases, stroke, foot conditions, neurological conditions (i.e., Parkinson’s) [1]. Gait speed is thus a well-known indicator for assessing an individual’s functional mobility [2]. Machine learning (ML) shows great potential in estimating gait speed due to its ability to reveal hidden patterns in large datasets and has already shown promising results over standard strap-down integration methods for foot-mounted inertial sensors (IMU) [3]. For the present investigation, we evaluated various supervised ML models to estimate gait speed based on IMU data collected on the most dominant lower limb. The Gait Events Data Set by Miraldo et al. [4] was used for the analysis. This dataset consists of metadata and inertial measurements (tri-axial accelerometer and gyroscope @6.75ms/sample) from sensors fixed to the flat part of the tibia on the dominant side, collected from 22 healthy subjects. Each subject walked barefoot six times at three randomly ordered, self-paced speeds (comfortable, slow, fast) on a 40-m long 2-m wide walkway, without curves, with a flat and rigid surface. The trials lasted 30-to-60 seconds. Overall, the dataset contains 9,661 gait strides. The IMU data for each trial was segmented into strides by the method proposed in [5]. Several features (Table 1) were extracted from each stride [6] and then averaged over the length of the trial to be used as input to several multiple linear regression and tree-based models along with participant anthropometrics. The number of trials were split 80%/20% into training and test sets with data from the same subject appearing only in either one or the other. A grid-search was performed on the training set using leave-one-subject-out cross-validation (LOSO-CV) to find the best performing model and mean absolute percentage error (MAPE) reported as the validation metric. The selected model was compared to a dummy regression model used as a baseline. Tables 2-3 show the results for the different models. Ridge regression showed the best results with MAE equal to 0.12 m/s and MAPE to 9.13%, almost three times lower compared to baseline. Fig. 1 shows the difference between ground truth and predicted speed across different subjects, the related Bland-Altman plot, and a sample of the obtained error for one trial. Results obtained are comparable to results in [5,6]. Download : Download high-res image (674KB)Download : Download full-size image This investigation shows that Ridge regression using features extracted from detected gait events in IMU data and participant anthropometrics is an appropriate approach to estimate gait speed, outperforming several other linear and tree-based regression models. The final model achieved promising results with a considerably lower error than the baseline, although further investigation is needed to confirm efficacy at atypical gait speeds, i.e., with populations with movement disorders. Funding: This work was supported in part by Enterprise Ireland (EI) and Abbvie, Inc. under agreement IP 2017 0625; and in part by the Science Foundation Ireland which are Co-Funded through the European Regional Development Fund under Grant 12/RC/2289-P2-INSIGHT.
更多查看译文
关键词
inertial sensors,gait,speed,machine learning
AI 理解论文
溯源树
样例
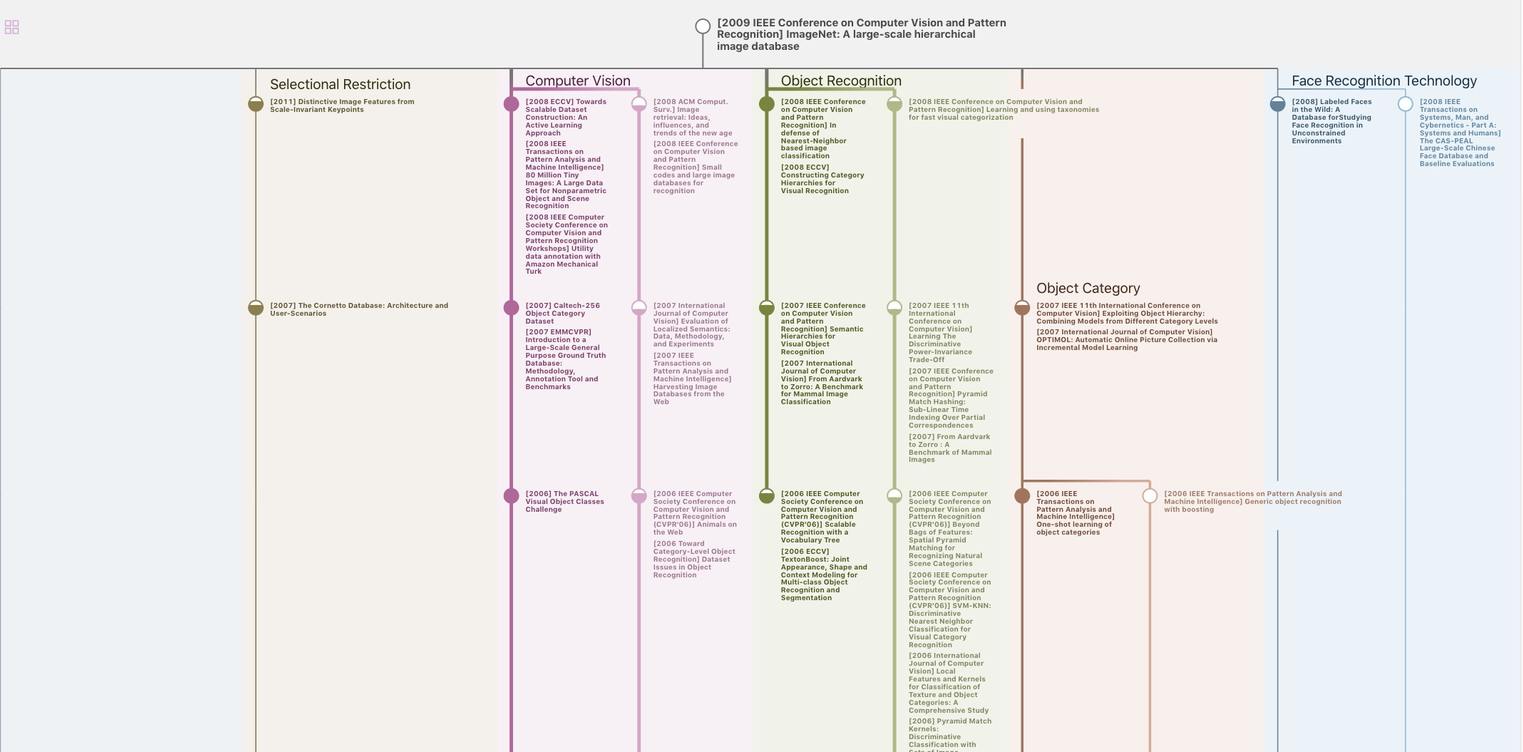
生成溯源树,研究论文发展脉络
Chat Paper
正在生成论文摘要