Co-Optimization of Cell Selection and Data Offloading in Sparse Mobile Crowdsensing.
IEEE Trans. Mob. Comput.(2024)
摘要
Cell selection and data offloading are the keys to obtaining MCS services with low sensing cost and low data processing delay. Due to the spatiotemporal correlation between data and the local-area coverage of edge servers, cell selection and data offloading will affect each other and require co-optimization. To achieve the co-optimization, we design the method OptInter based on the hierarchical reinforcement learning. OptInter can realize the interactive training between cell selection model and data offloading model. Finally, we evaluate our proposed method based on four datasets, each of which composited by real-world (e.g. NO
$_{2}$
concentration, AQI value, Didi order, and Didi trajectory) data and simulated data. Compared with the four baseline methods (e.g., OptMOEA/D, OptStageCD, OptStageDC and OptWeight), the comprehensive performance of our proposed method can be improved by 11.83%, 20.48%, 10.14% and 42.27% on average, respectively.
更多查看译文
关键词
Sparse Mobile Crowdsensing,data offloading,cell selection,Co-optimization,hierarchical reinforcement learning
AI 理解论文
溯源树
样例
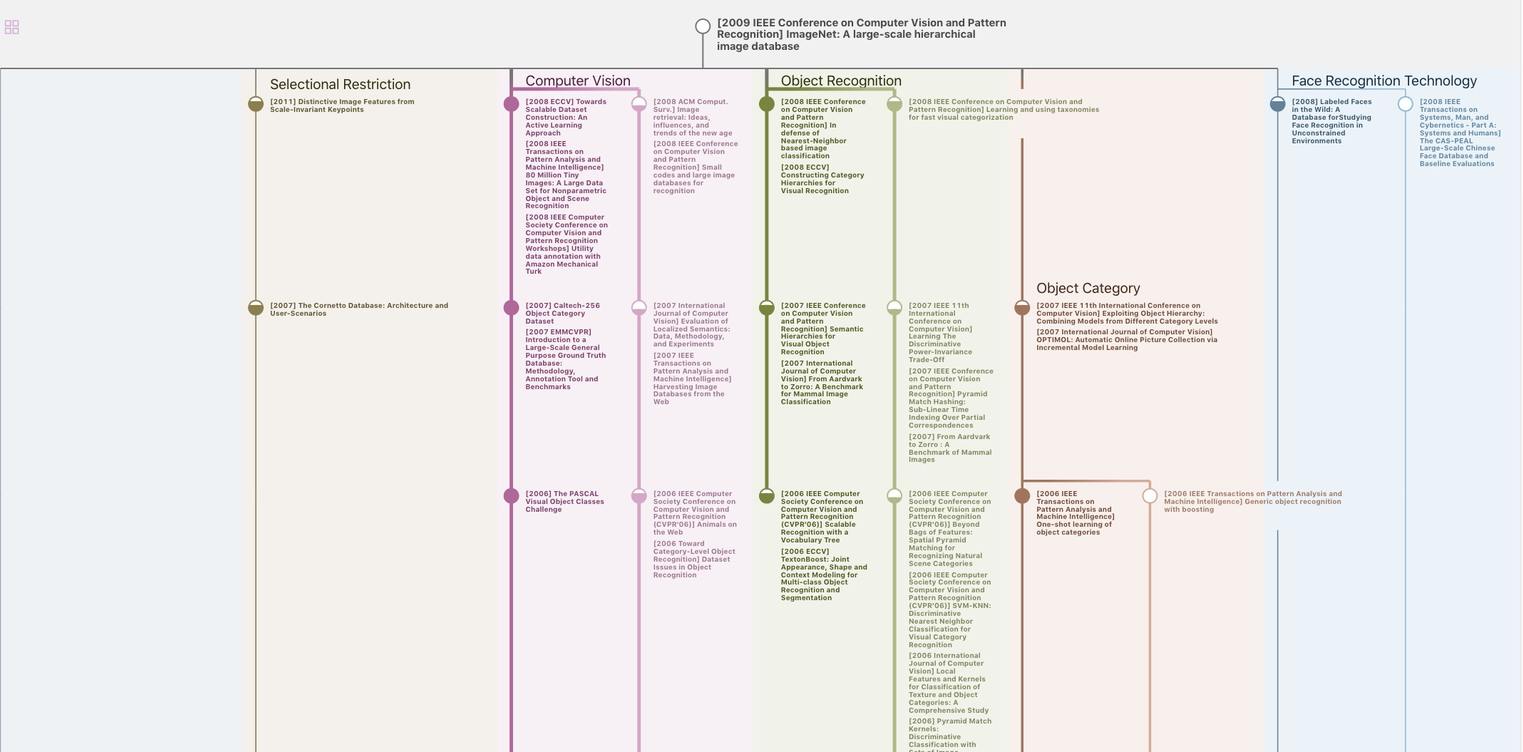
生成溯源树,研究论文发展脉络
Chat Paper
正在生成论文摘要