Neighborhood-Oriented Decentralized Learning Communication in Multi-Agent System
ARTIFICIAL NEURAL NETWORKS AND MACHINE LEARNING, ICANN 2023, PT III(2023)
摘要
Partial observations are one of the critical obstacles in multiagent systems (MAS). The Centralized Training Decentralized Execution (CTDE) paradigm has been widely studied to integrate global observations into the training process. However, the traditional CTDE paradigm suffers from local observations during the execution phase, and numerous efforts have been made to study the communication efficiency among agents to promote cognitive consistency and better cooperation. Furthermore, training still operates in a centralized manner, requiring agents to communicate with each other in a broadcast fashion. As a consequence, this centralized broadcast-based training process is not feasible when being applied to more complex scenarios. To address this issue, in this paper we propose a neighborhood-based learning approach to enable agents to perform training and execution in a decentralized manner based on information received from their neighboring nodes. In particular, we design a novel encoder network and propose a two-stage decision model to improve the performance of this decentralized training. To evaluate the method, we further implement a prototype and perform a series of simulation-based experiments to demonstrate the effectiveness of our method in multi-agent cooperation compared to selected existing multiagent methods to achieve the best rewards and drastically reduce data transmission during training.
更多查看译文
关键词
Multi-Agent System,Deep Reinforcement Learning,Learning Communication
AI 理解论文
溯源树
样例
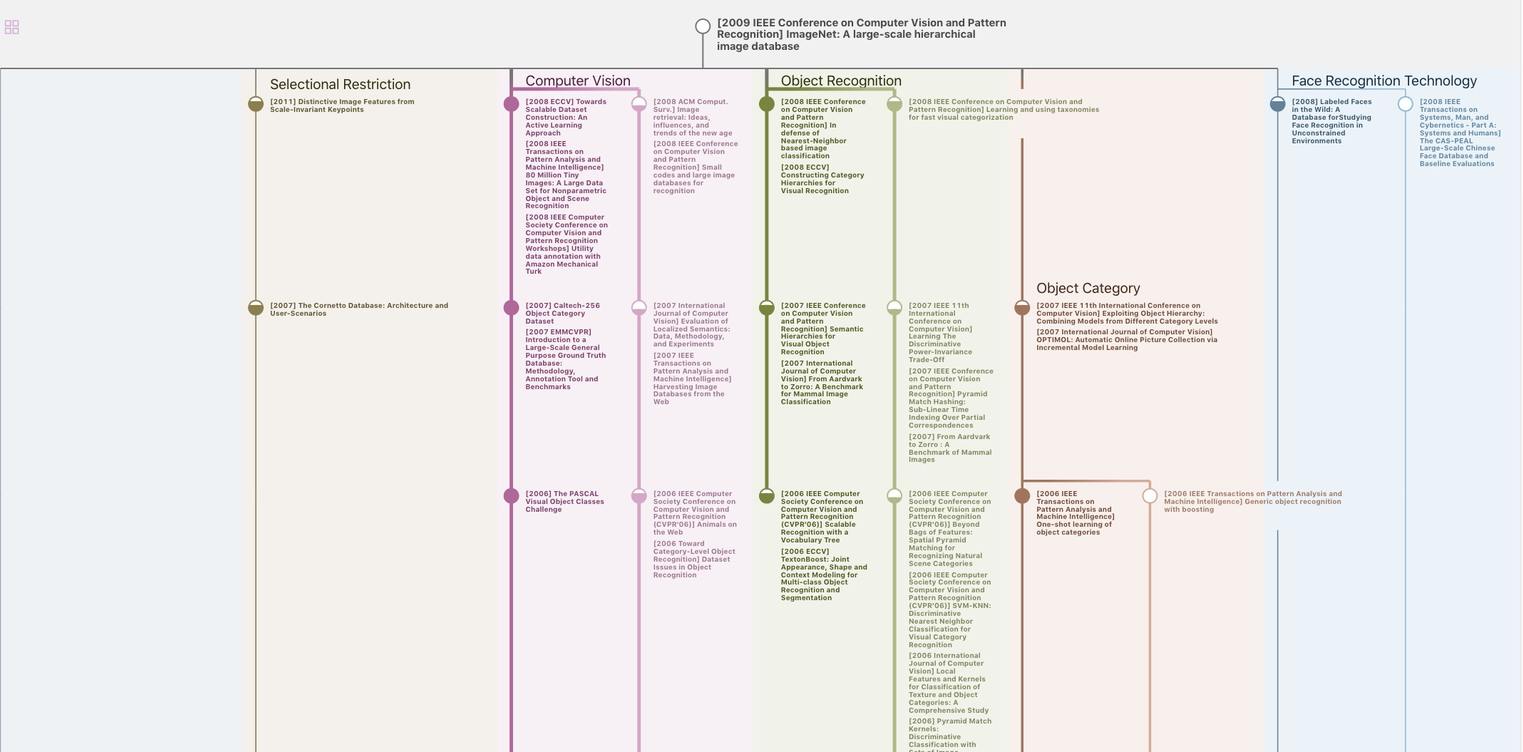
生成溯源树,研究论文发展脉络
Chat Paper
正在生成论文摘要