Tuning of Generalized K-Omega Turbulence Model by Using Adjoint Optimization and Machine Learning for Gas Turbine Combustor Applications
openalex(2023)
摘要
For swirl-stabilized gas turbine combustors, turbulence modelling is generally undertaken using Scale-Resolved Simulations (SRS) methods such as Large Eddy Simulations (LES). However, LES is expensive due to large mesh sizes and small time-step size required to estimate the correct large-scale turbulence motion generated within the combustor domain. While Reynolds Averaged Navier Stokes (RANS) models are computationally very efficient in predicting qualitative behaviour, such models lack fidelity to predict complex flow characteristics such as swirl accurately. In previous studies with commercial Computational Fluid Dynamics (CFD) code, the Generalized k-omega (GEKO) based RANS model has been used for simulation of turbulent flows. The free-coefficients of the GEKO model are tuned to predict the characteristics of potential flow undergoing separation. In this paper, LES predictions are used as baseline results which are used to generate a sensitivity field by using an Adjoint solver. Machine learning (ML) via Neural Network (NN) Training is utilized to create a correlation between suitable turbulent flow features and optimal GEKO parameters, aiding the trained model’s generalization. This allows computationally faster simulations of swirling flow with optimized GEKO model compared to LES. In this paper, an industrial configuration named as the DLR PRECCINSTA burner has been used considering a cold non-reacting flow. The computations are performed with LES, and the results match the experiments for directional velocities at different sections within the combustor. The LES solution is used to train the GEKO sensitivity by using an Adjoint solver. The objective function defined in this work is based on the linear combination of difference in velocity components, and a Neural Network is trained to obtain an optimized GEKO model. The results with optimized GEKO agree qualitatively and quantitatively with LES and experiments. The generalization of the NN model is tested on different flow conditions, including a range of Reynolds numbers as well as a reacting flow scenario. The results are showing significant improvements over the baseline solution as compared to the experiment. An optimized workflow is established, which can be used in future studies concerning different geometries with similar flow fields.
更多查看译文
关键词
gas turbine combustor applications,gas turbine,adjoint optimization,machine learning,k-omega
AI 理解论文
溯源树
样例
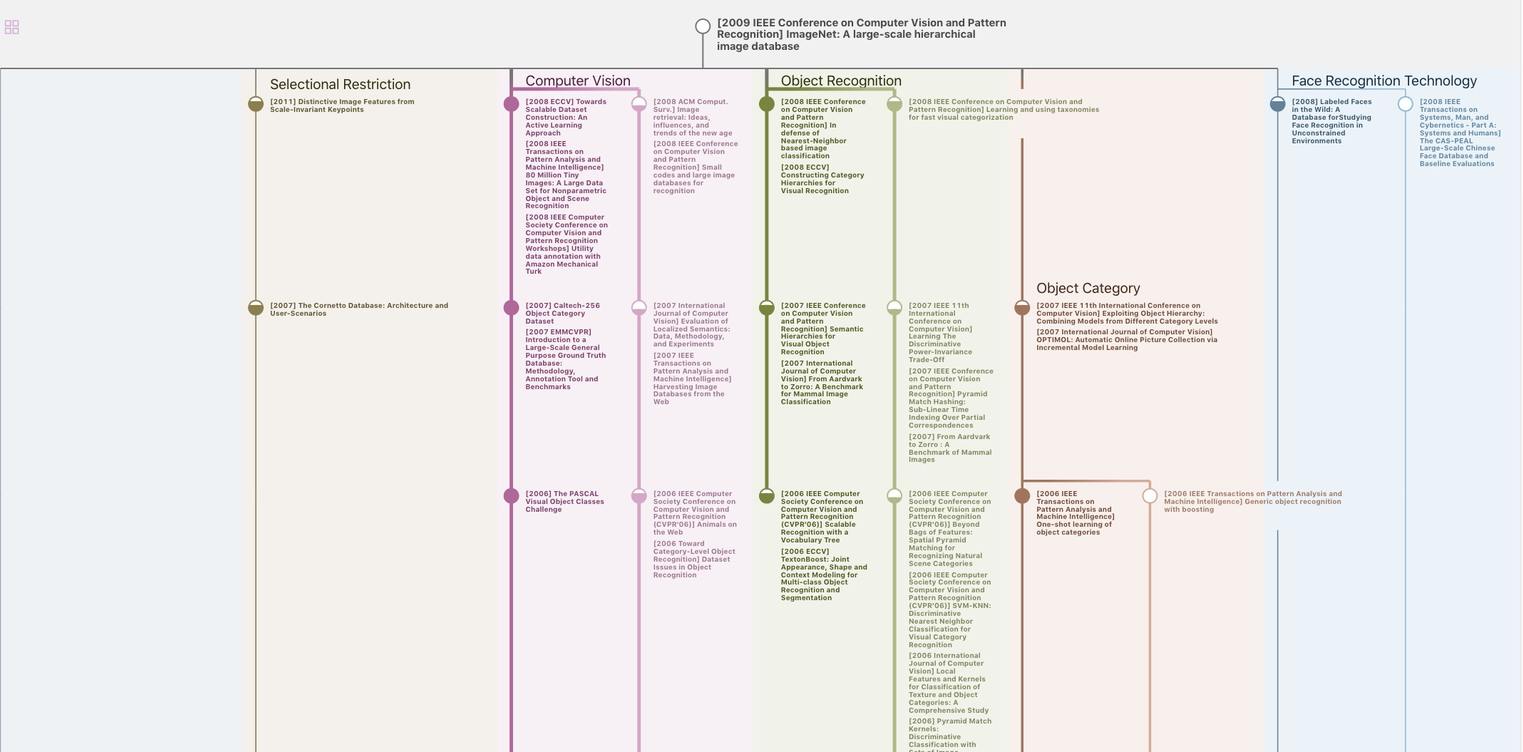
生成溯源树,研究论文发展脉络
Chat Paper
正在生成论文摘要