Non-rigid Interest Object Extraction Using Minimum Convex Coverage
Research Square (Research Square)(2023)
摘要
Abstract Interest object (IO) extraction, or called region of interest (ROI) selection, is a fundamental task in remote sensing (RS), computer vision, and machine learning. Due to the non-rigidity, e.g., shapelessness and color-uncertainty, of interest objects like fires, smokes, clouds, or top-view tree canopies in a RS image, an unideal results usually occurs in IO detection tasks because the model-training is carried on the pixels drawn from an inaccurate ROI, e.g., a bounding-box or convex region. Although it is simple, it fails to capture a complete interest object or sub-object, especially at the requirement of high-precision. In this work, we focus on the non-convex ROI and develop a method for the accurate pixel extraction. To speed pixel extraction, the given non-convex ROI is first decomposed into a minimum convex coverage, and then the ROI pixels can be quickly extracted out from the coverage by using convex operation. For the decomposition , we provide two strategies, named inner decomposition and outer decomposition. Computationally, batch-decision for pixel selection and logical operation for the decomposition are also presented to speed extraction. Theoretically , the existence of minimum coverage for both decompositions is proved. Finally, extensive experimental verifications are carried out on public and our collected RS images, and the results show the superiority of our method in terms of ease of use, rapidity and pixel-precision.
更多查看译文
关键词
object,non-rigid
AI 理解论文
溯源树
样例
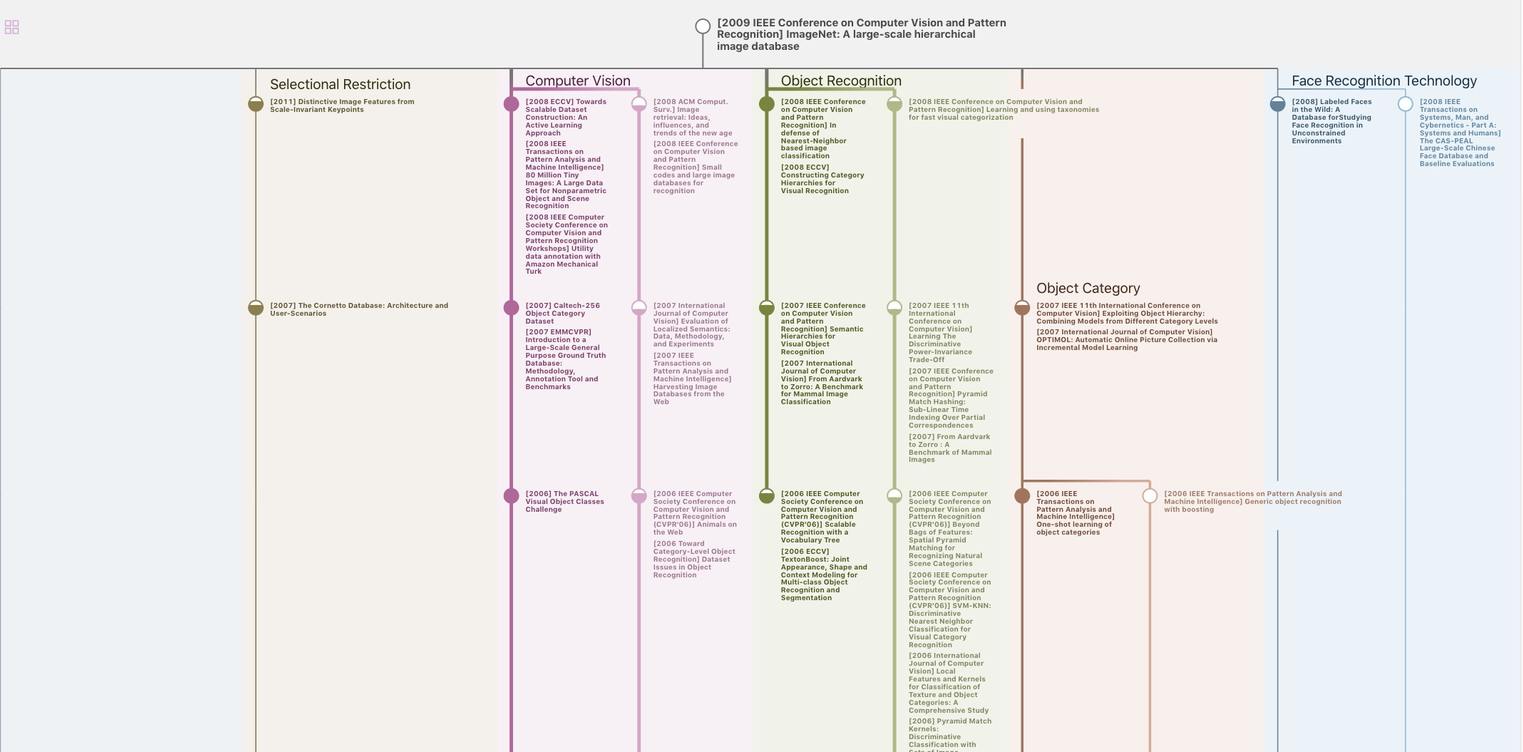
生成溯源树,研究论文发展脉络
Chat Paper
正在生成论文摘要