A Neural Tangent Kernel View on Federated Averaging for Deep Linear Neural Network
arXiv (Cornell University)(2023)
摘要
Federated averaging (FedAvg) is a widely employed paradigm for collaboratively training models from distributed clients without sharing data. Nowadays, the neural network has achieved remarkable success due to its extraordinary performance, which makes it a preferred choice as the model in FedAvg. However, the optimization problem of the neural network is often non-convex even non-smooth. Furthermore, FedAvg always involves multiple clients and local updates, which results in an inaccurate updating direction. These properties bring difficulties in analyzing the convergence of FedAvg in training neural networks. Recently, neural tangent kernel (NTK) theory has been proposed towards understanding the convergence of first-order methods in tackling the non-convex problem of neural networks. The deep linear neural network is a classical model in theoretical subject due to its simple formulation. Nevertheless, there exists no theoretical result for the convergence of FedAvg in training the deep linear neural network. By applying NTK theory, we make a further step to provide the first theoretical guarantee for the global convergence of FedAvg in training deep linear neural networks. Specifically, we prove FedAvg converges to the global minimum at a linear rate $\mathcal{O}\big((1-\eta K /N)^t\big)$, where $t$ is the number of iterations, $\eta$ is the learning rate, $N$ is the number of clients and $K$ is the number of local updates. Finally, experimental evaluations on two benchmark datasets are conducted to empirically validate the correctness of our theoretical findings.
更多查看译文
关键词
neural tangent kernel view,federated averaging,deep linear
AI 理解论文
溯源树
样例
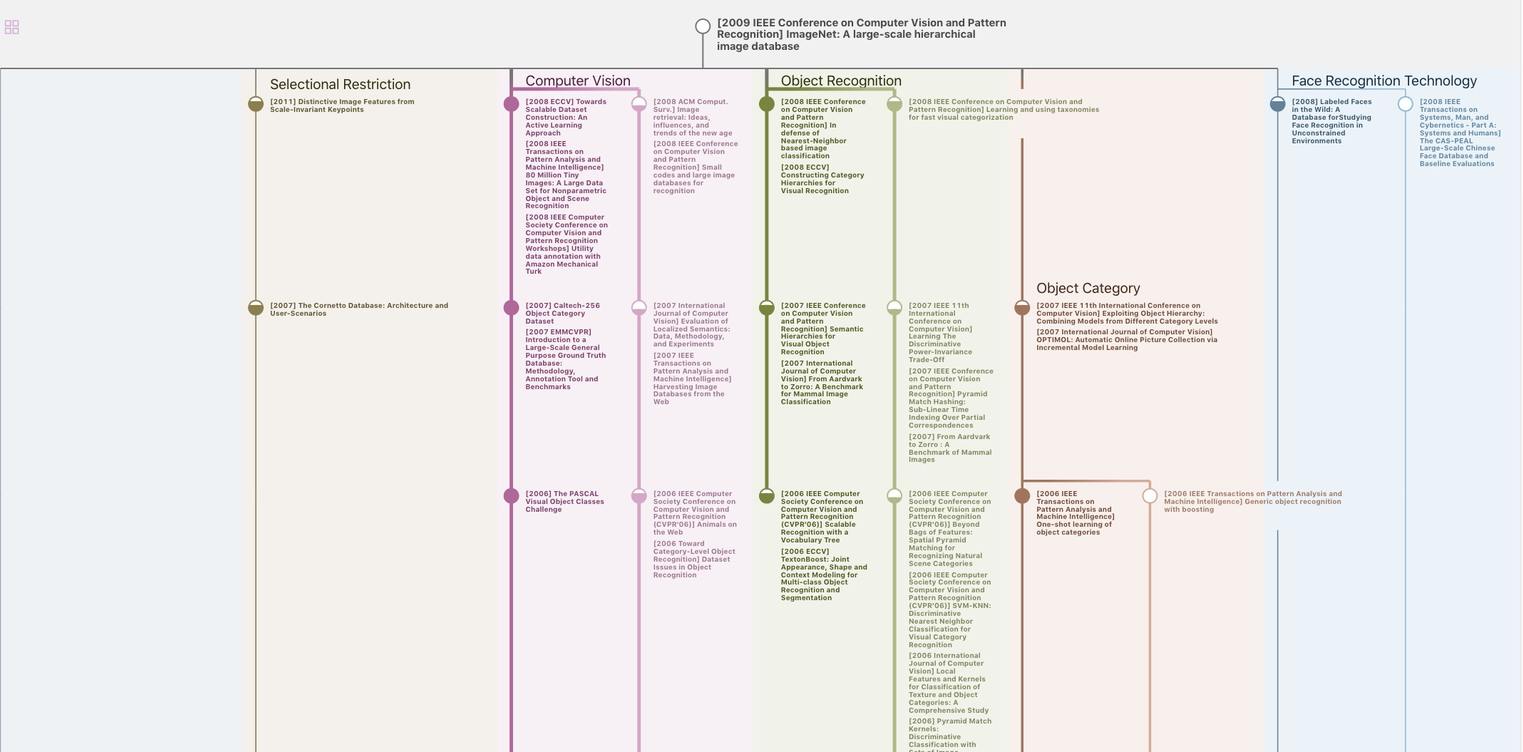
生成溯源树,研究论文发展脉络
Chat Paper
正在生成论文摘要