Accurate Prediction of HSE06 Band Structures for a Diverse Set of Materials Using -Learning
Chemistry of Materials(2023)
摘要
We used machine learning (ML) to accurately predict eigenvalues of the hybrid HSE06 functional using eigenvalues computed by the less computationally expensive PBE functional and associated electronic features based on the k-point resolved atomic band character. The ML model was trained by using eigenvalues from only one k-point for each of the 168 compounds in the training set. The HSE06 eigenvalues across all k-points were then predicted for a separate set of 169 compounds with a mean absolute error (MAE) of 0.13 eV, representing a significant improvement over the error of PBE-computed eigenvalues relative to that of HSE06 (MAE = 0.96 eV). These accurately predicted eigenvalues result in remarkably accurate predictions for the band structures, projected density of states, and band gaps, even though the model was not explicitly trained on these other properties. Finally, we demonstrate that our ML model has a similar accuracy for both ternary and quaternary compounds well outside the initial training set and on systems with 112 and 160 atoms, demonstrating its potential to rapidly predict HSE06-quality electronic structures of complex materials that are practically unfeasible for HSE06.
更多查看译文
关键词
hse06 band structures,band structures,materials,prediction
AI 理解论文
溯源树
样例
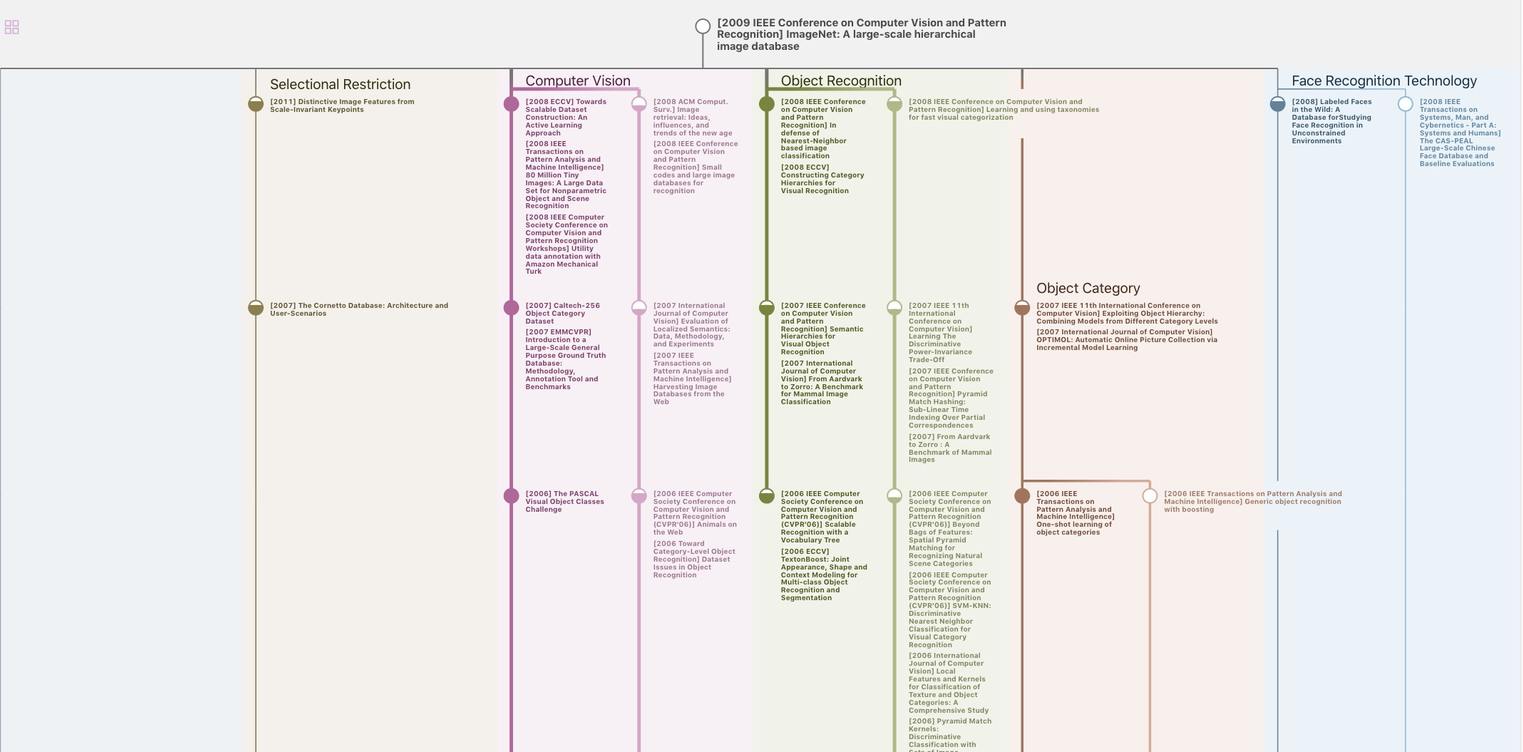
生成溯源树,研究论文发展脉络
Chat Paper
正在生成论文摘要