Quantum-noise-limited optical neural networks operating at a few quanta per activation
Research Square (Research Square)(2023)
摘要
Abstract A practical limit to energy efficiency in computation is ultimately from noise, with quantum noise [1] as the fundamental floor. Analog physical neural networks [2], which hold promise for improved energy efficiency and speed compared to digital electronic neural networks, are nevertheless typically operated in a relatively high-power regime so that the signal-to-noise ratio (SNR) is large (>10). We study optical neural networks [3] operated in the limit where all layers except the last use only a single photon to cause a neuron activation. In this regime, activations are dominated by quantum noise from the fundamentally probabilistic nature of single-photon detection. We show that it is possible to perform accurate machine-learning inference in spite of the extremely high noise (signal-to-noise ratio ~1). We experimentally demonstrated MNIST handwritten-digit classification with a test accuracy of 98% using an optical neural network with a hidden layer operating in the single-photon regime; the optical energy used to perform the classification corresponds to 0.008 photons per multiply-accumulate (MAC) operation, which is equivalent to 0.003 attojoules of optical energy per MAC. Our experiment also used >40× fewer photons per inference than previous state-of-the-art low-optical-energy demonstrations [4, 5] to achieve the same accuracy of >90%. Our training approach, which directly models the system’s stochastic behavior, might also prove useful with non-optical ultra-low-power hardware.
更多查看译文
关键词
optical neural networks,few quanta,neural networks,quantum-noise-limited
AI 理解论文
溯源树
样例
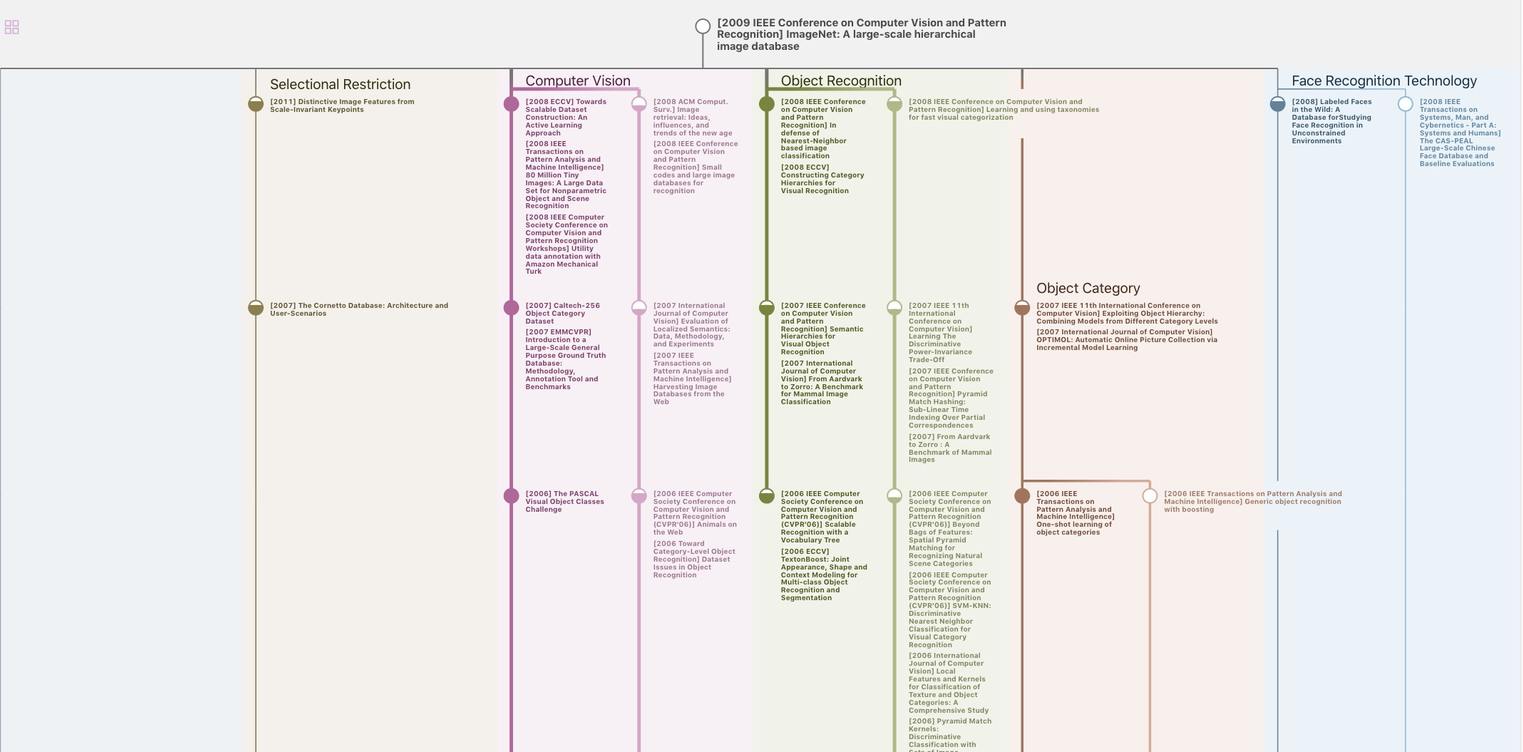
生成溯源树,研究论文发展脉络
Chat Paper
正在生成论文摘要