Nimg-45. external evaluation of a machine learning model employing radiomics to identify regions of local recurrence in glioblastoma from postoperative mri
Neuro-oncology(2023)
摘要
The standard surgical goal for operable glioblastomas worldwide is the complete excision of the enhancing tumor. Nevertheless, the peritumoral region harbors infiltrating cells leading to recurrence. Our study aims to evaluate a predictive model designed to predict potential regions of recurrence using voxel-based radiomics analysis on postoperative magnetic resonance imaging (MRI). This retrospective, multi-institutional study involved glioblastoma patients who had undergone complete resection. The training cohort comprised 49 patients from two Spanish institutions, with model evaluation using an external dataset of 27 patients from a Norwegian institution and the public Ivy-Gap dataset. We used follow-up MRI scans for ground truth definition of recurrence, while postoperative multiparametric MRI scans provided the data for extracting voxel-based radiomic features. Our method employed an unsupervised, hybrid approach, which combined a deep neural network and instance optimization, to align the MRI sequences for each patient, registering the follow-up with the postoperative scans. To generate ground truth labels, we segmented the peritumoral region in the postoperative scans, identified as the area showing T2/FLAIR signal alterations, in addition to the enhancing tumor visible in the registered follow-up scan. Overlapping voxels between the peritumoral and enhancing tumor regions were classified as recurrence, while non-overlapping voxels were labeled as nonrecurrence. Radiomic features were subsequently extracted from the postoperative peritumoral region. We trained four machine learning classifiers to predict recurrence. Among these, the Categorical Boosting (CatBoost) classifier demonstrated excellent performance on the test dataset, achieving an average area under the curve (AUC) of 0.83 ± 0.07, and an accuracy of 0.80 ± 0.12, based on voxel-wise comparison with the ground truth. Our study resulted in a method that accurately predicts the area of future tumor recurrence in glioblastoma patients' MRI scans. This innovation could potentially tailor surgical and radiotherapy treatment strategies to these specific areas, possibly extending patient survival.
更多查看译文
关键词
glioblastoma,radiomics,mri,machine learning model,machine learning
AI 理解论文
溯源树
样例
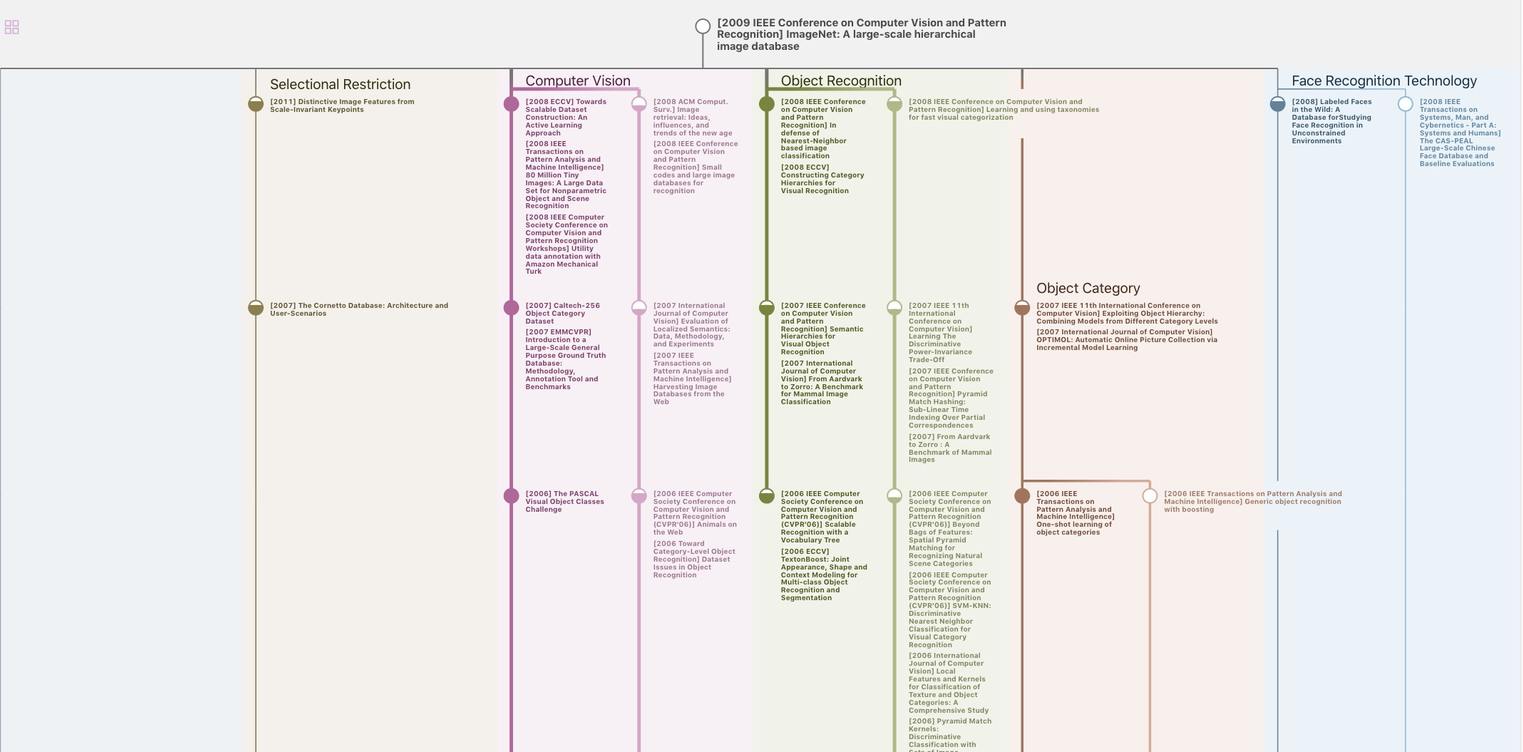
生成溯源树,研究论文发展脉络
Chat Paper
正在生成论文摘要