Inferring interactions from microbiome data
bioRxiv (Cold Spring Harbor Laboratory)(2023)
摘要
Abstract Parameter inference of high-dimensional data is challenging and microbiome time series data is no exception. Methods aimed at predicting from point estimates exist, but often even fail to recover the true parameters from simulated data. Computational methods to robustly infer and quantify the uncertainty in model parameters are needed. Here, we propose a computational workflow addressing such challenges – allowing us to compare mechanistic models and identify the values and the certainty of inferred parameters. This approach allows us to infer which kind of interactions occur in the microbial community. In contrast to point-estimate inference, the distribution for the parameters, our outcome, reflects their uncertainty. To achieve this, we consider as many equations for the statistical moments of the microbiome as parameters. Our inference workflow, which builds upon a mechanistic foundation of microscopic processes, can take into account that commonly metagenomic datasets only provide information on relative abundances and hosts’ ensembles. With our framework, we move from qualitative prediction to quantifying the likelihood of certain interaction types in microbiomes.
更多查看译文
关键词
interactions
AI 理解论文
溯源树
样例
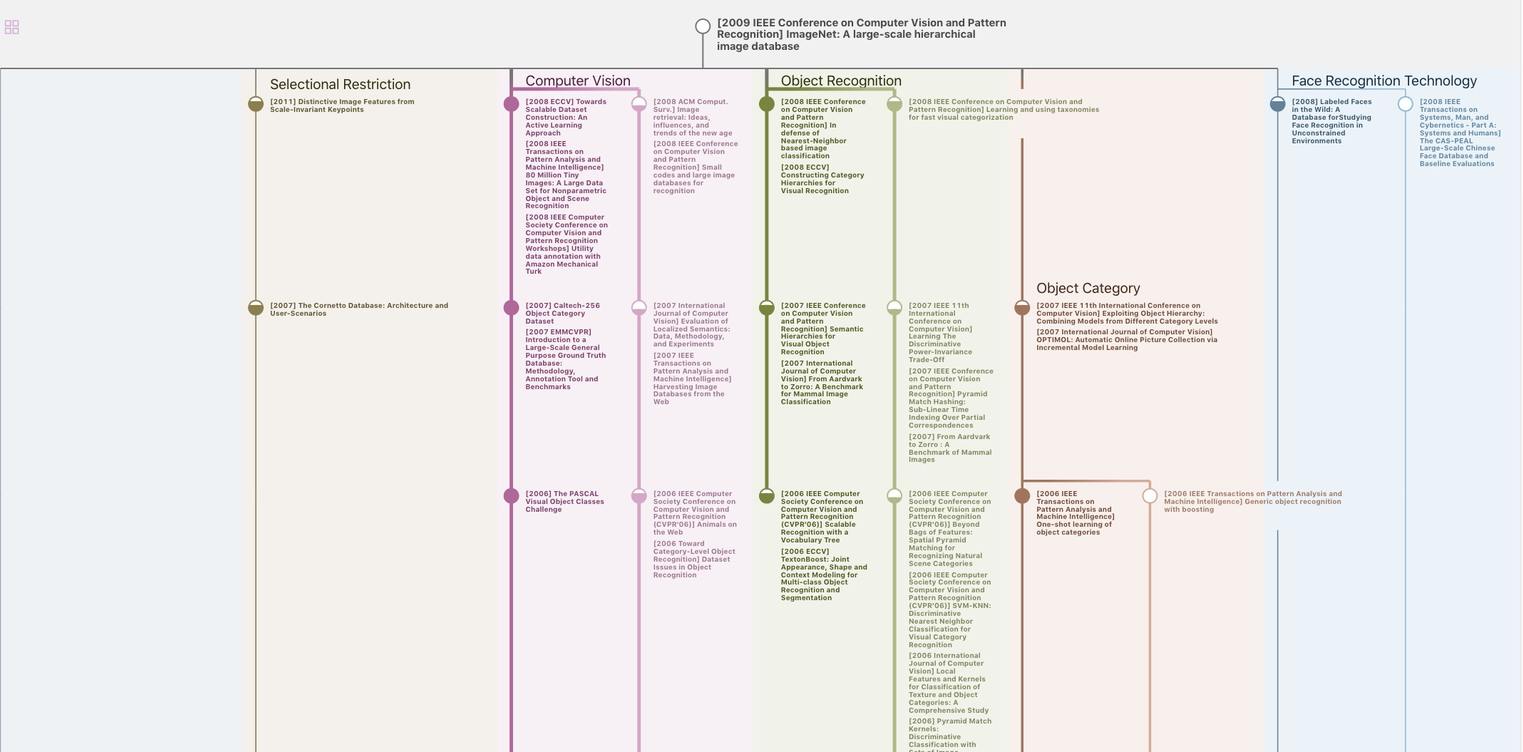
生成溯源树,研究论文发展脉络
Chat Paper
正在生成论文摘要