A Neighborhood-aware Multi-markovian Switching Particle Swarm Optimization Technique for Solving Complex and Expensive Problems
Research Square (Research Square)(2023)
摘要
Abstract Particle swarm optimization approach (PSO) has illustrated promising performance on a variety of optimization problems, two critical issues that must be addressed in PSO research are how to balance conflicts around exploration, exploitation and reasonably distribute computational resources. In this paper, a neighborhood-aware N states markovian switching PSO algorithm (NS-MJPSO$loc$) is proposed, where we have employed local search based approach. The neighborhood of PSO algorithm is a single particle itself in the early stages of optimization. The neighborhood will eventually expand to include all particles as the amount of generations increases. The search space is segmented into N potential states according to the size of problem. The value of N is dependent on the dimension of problem space. Every iteration serves a transition probability-based markovian switching technique to estimate the acceleration coefficients in an adaptable way. The transition probability determines the next state and the adequate values of an acceleration coefficients. Through adopting a local search strategy while interacting with all other particles in the group, perhaps every particle possesses chance of improving itself. Moreover, new features have been incorporated to accomplish accuracy and stability. The primary contributions of the proposed work are to enhance the topological structure of a recently developed PSO variant by using a local search ( loc ) mechanism rather than a global ( gbest ). The purpose of this idea is to develop a more adaptable and less sensitive novel version of PSO that can handle multi-modal, highly challenging problems. The proposed algorithm was then tested using a variety of uni-modal, multi-modal and rotated benchmark functions. The experimental findings show that the proposed NS-MJPSO loc outperform most of the current versions in terms of accuracy and convergence time, notably in multi-modal problems.
更多查看译文
关键词
Particle swarm optimization,Markov chain,Evolutionary factor,Large-scale optimization,Scalability
AI 理解论文
溯源树
样例
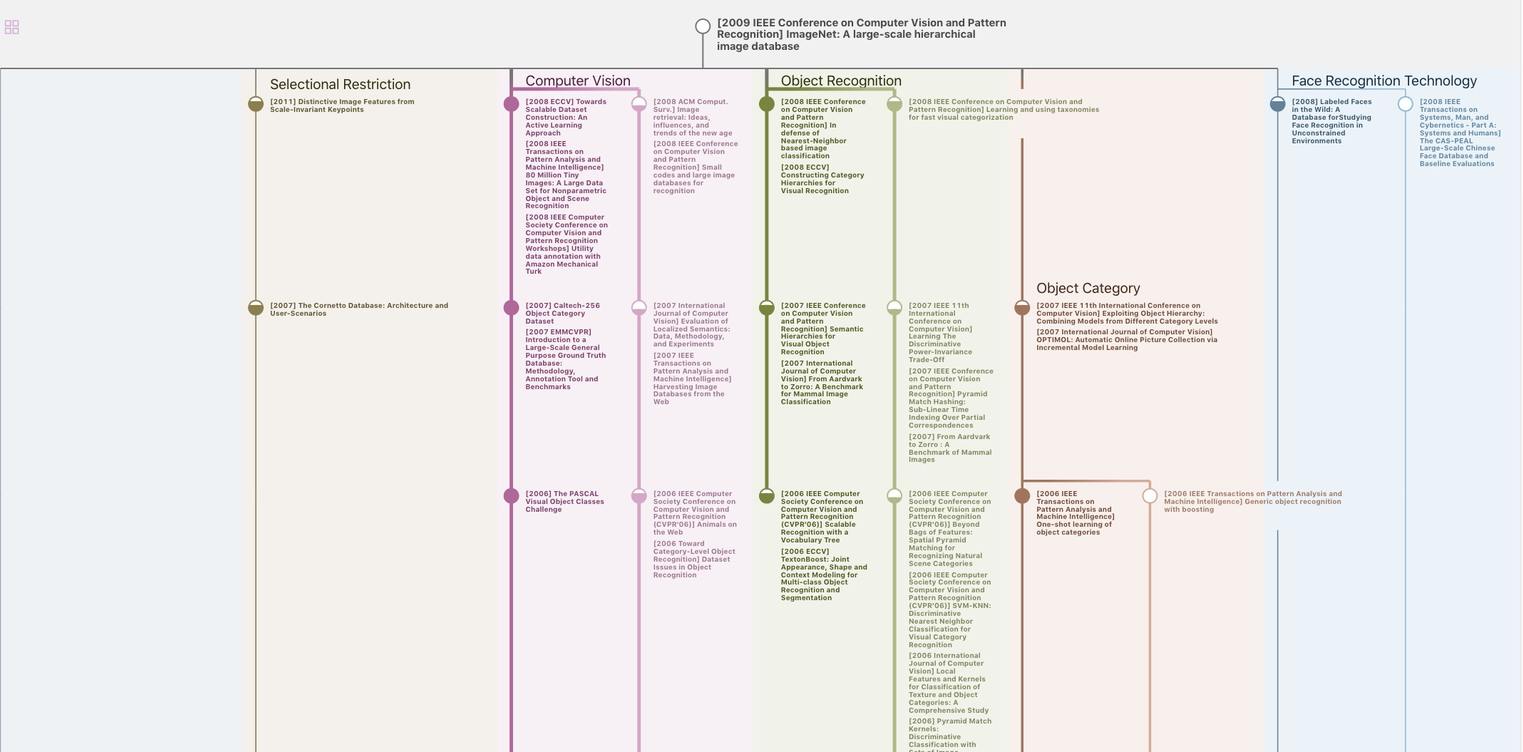
生成溯源树,研究论文发展脉络
Chat Paper
正在生成论文摘要