Neural network-based diffuse fluorescence tomography reconstruction algorithms to improve image quality
Authorea (Authorea)(2023)
摘要
Diffuse fluorescence tomography (DFT) is a promising noninvasive imaging modality. To date, traditional physics-based approaches cannot substantially improve the imaging quality due to inherent light scattering property and severe ill-poseness of inverse problem. Therefore, three neural networks, namely, back-propagation neural network (BPNN), convolutional neural network (CNN) and U-net trained in an end-to-end fashion are explored to improve imaging quality. To evaluate their performance, a series of simulation, phantom and in vivo experiments are conducted for single and double-target reconstructions, and the results are compared with the traditional algebraic reconstruction technique (ART). The numerical results demonstrate that the neural network-based algorithms significantly enhance the image quality in terms of target shape, size, location, as well as noise robustness. The subsequent phantom and in vivo experimental results verify the effectiveness of the well-trained networks to estimate experimental data. Comparatively, BPNN is competitive in computational efficiency and generalization capacity.
更多查看译文
关键词
diffuse fluorescence tomography reconstruction,image quality,network-based
AI 理解论文
溯源树
样例
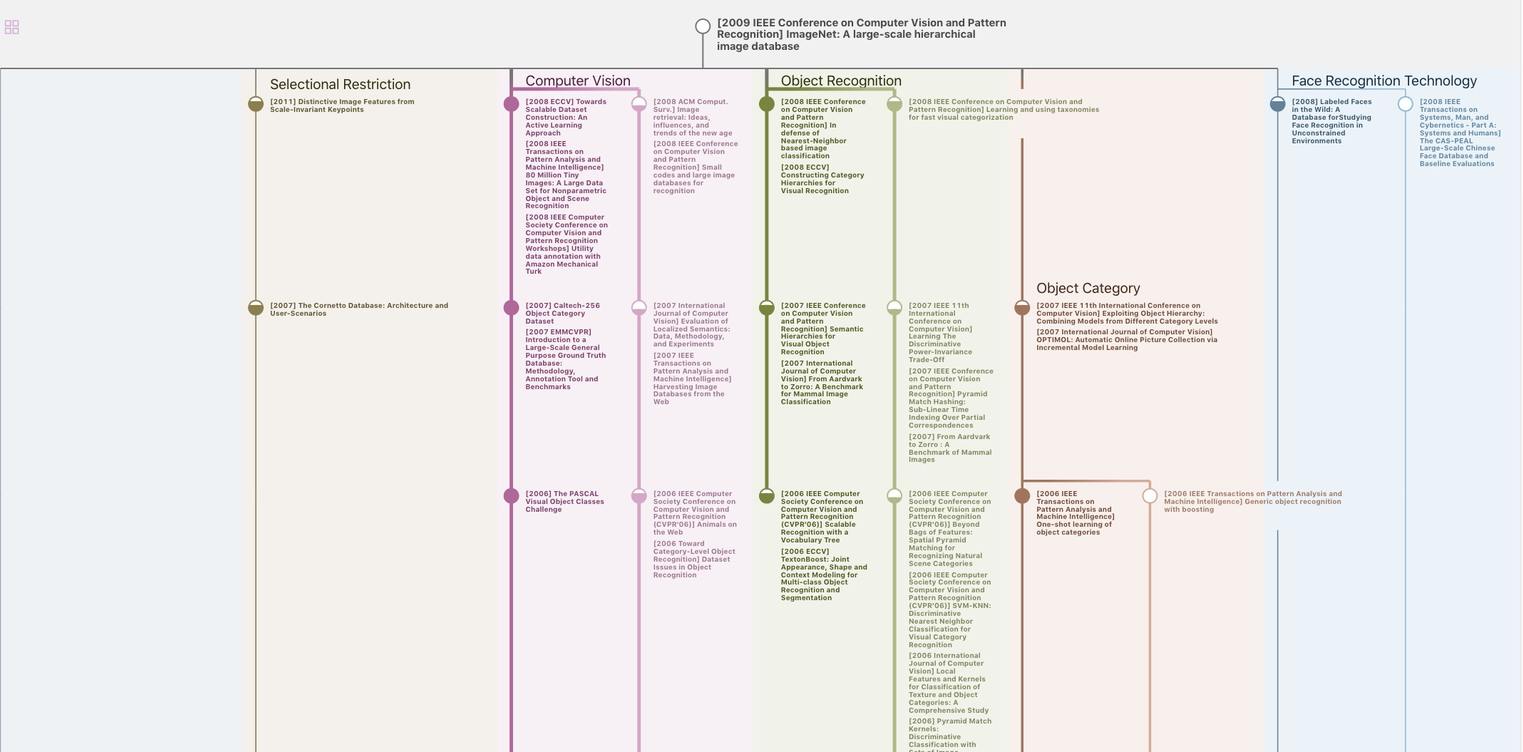
生成溯源树,研究论文发展脉络
Chat Paper
正在生成论文摘要