A new GRACE downscaling approach for deriving high-resolution groundwater storage changes using ground-based scaling factors
Authorea (Authorea)(2023)
摘要
To compensate for the intrinsic coarse spatial resolution of groundwater storage (GWS) anomalies (GWSA) from the Gravity Recovery and Climate Experiment (GRACE) satellites and make better use of current dense in situ groundwater-level data in some regions, a new statistical downscaling method was proposed to derive high-resolution GRACE GWS changes. A ground-based scaling factor (SFGB) method was proposed to downscale GRACE GWS changes that were corrected using gridded scaling factors estimated from ground-based GWS changes through forward modeling. The proposed method was applied in the North China Plain (NCP), where many observation wells and consistently measured specific yield are available. Importantly, the sensitivity of the proposed method was explored considering the uncertainties of in situ GWS changes due to variable specific yield and/or number of observation wells. Independent validation shows that SFGB can effectively recover GRACE GWSA at the 0.5º grid scale (r = 0.81, root mean square error = 40.51 mm/yr). The SFGB-corrected GWSA in the NCP was -32.60{plus minus}0.99 mm/yr (-4.6{plus minus}0.14 km3/yr) during 2004-2015, showing contrasting GWS trends in the piedmont west (loss) and the coastal east (gains). Uncertainties in SFGB-corrected GWSA arising from specific yield, groundwater-level, and both can be reduced by 90%, 65%, and 84%, respectively relative to ground-based GWSA. This study highlights the potential value of jointly using GRACE and in situ observation data to improve the accuracy of GRACE-derived GWSA at smaller scales. The new downscaling method and the improved groundwater storage change estimates would facilitate better groundwater management.
更多查看译文
关键词
high-resolution,ground-based
AI 理解论文
溯源树
样例
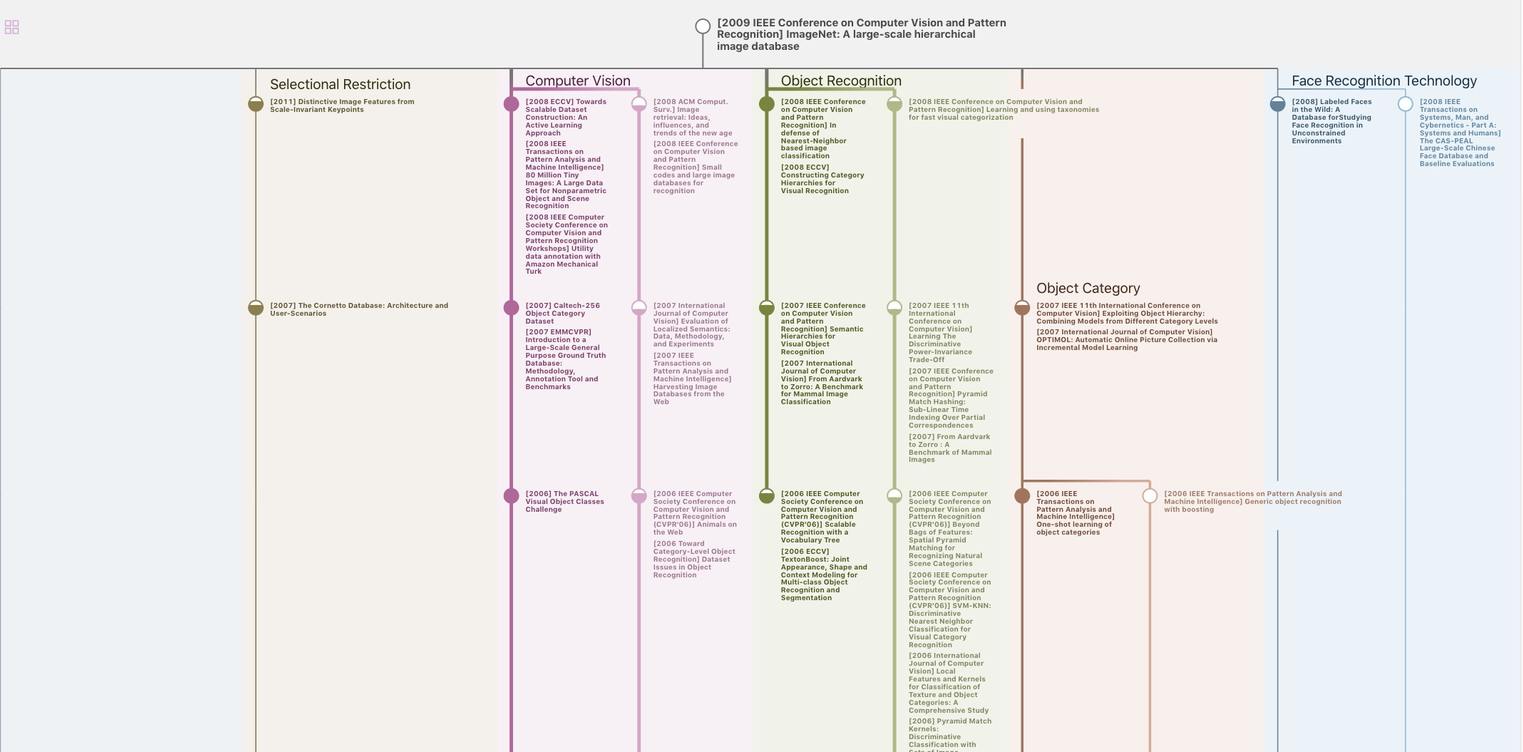
生成溯源树,研究论文发展脉络
Chat Paper
正在生成论文摘要