Manually-established abnormal karyotype dataset based on normal chromosomes effectively train artificial intelligence model for better cytogenetic abnormalities prediction
Research Square (Research Square)(2023)
摘要
Abstract With the advent of the utilization of machine learning techniques in the diagnosis of hematological diseases, endless potential can be foreseen, including digital images analysis. The application of machine-learning tool in cytogenetics contributes to the lightening of manpower burden, the improvement of recognition efficiency and the enrichment of cytogenetic maps, which paves the way for the development of digital pathology. Chromosome banding analysis is an essential technique for chromosome karyotyping, which comprises of one of important tools for the diagnostics in hematological malignancies. Its important role has been emphasized in clinic for dozens of years till now. The recognition of abnormal karyotypes is indispensable for disease classification and even diagnosis. However, a lack of abnormal karyotype images as reference dataset restricts its utilization in clinic, especially for uncommon hematological diseases. Here, to our best knowledge, we, for the first time, successfully generated abnormal karyotype images of t(9;22)(q34;q11)manually from normal karyotype images using machine learning, providing a proof-of-concept for establishing abnormal karyotypes of hematological malignancies as clinical reference. Moreover, to verify the reliability of generated abnormal dataset, artificial intelligence (AI)-recognizing models were further established based on ‘manually-built’ karyogram dataset and real karyotype dataset, respectively. The results showed that there was no difference between ‘manually-built’ karyotype dataset derived AI model (model-M) and real karyotype dataset derived AI model (model-R) regarding the recognition of t(9;22)(q34;q11) abnormality, with model-M (AUC=0.984, 95%CI 0.98-0.988) versus model-R (AUC=0.988, 95%CI 0.984-0.993) (p>0.05), which pointed out that our generated abnormal karyotype images were comparable to real images to assist the establishment of AI-recognising models. Collectively, our work demonstrates the potential application of machine learning in generating unlimited dataset from limited sources, helping to overcome the big challenge of AI in healthcare.
更多查看译文
关键词
abnormal karyotype dataset,abnormal chromosomes,artificial intelligence,artificial intelligence model,manually-established
AI 理解论文
溯源树
样例
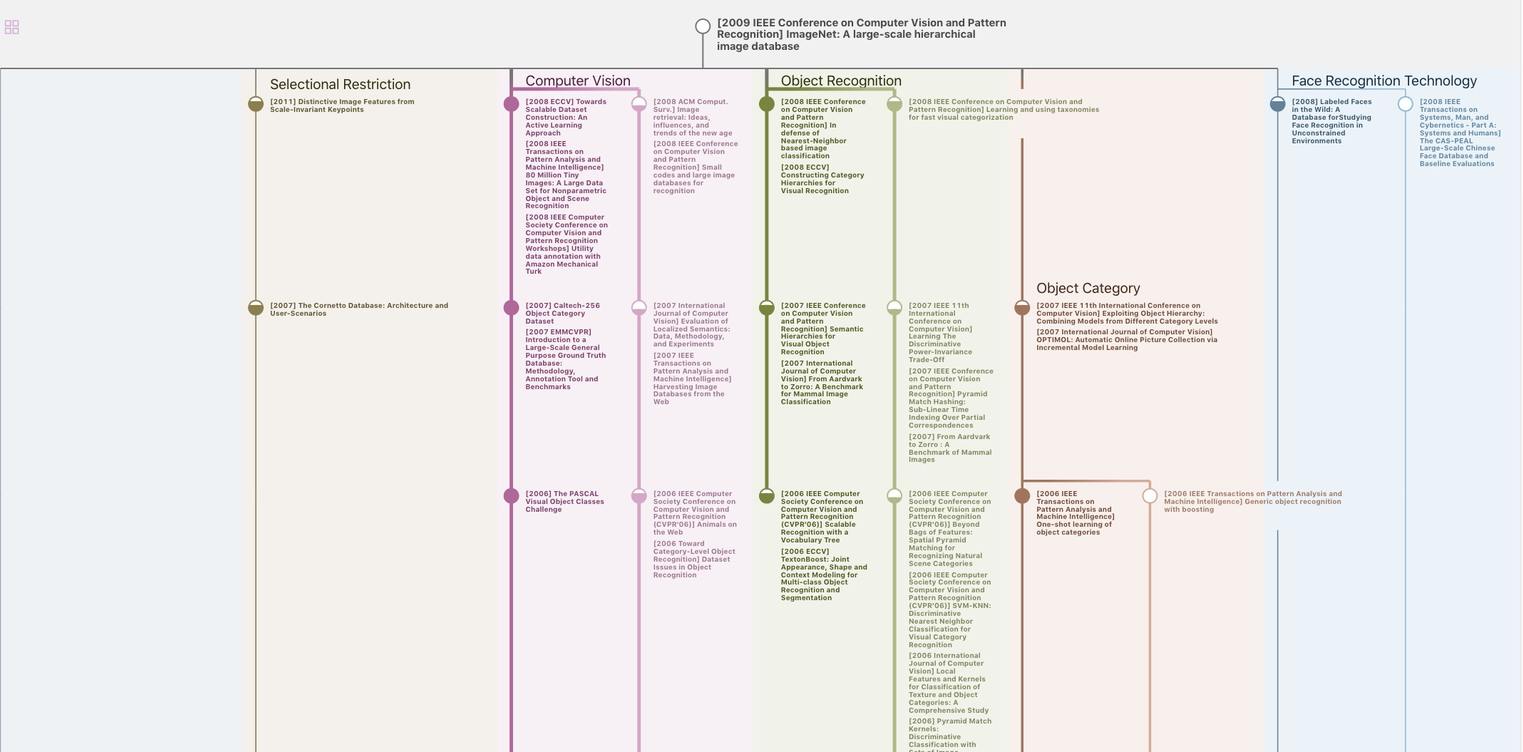
生成溯源树,研究论文发展脉络
Chat Paper
正在生成论文摘要