Mode-Aware Continual Learning for Conditional Generative Adversarial Networks
arXiv (Cornell University)(2023)
摘要
The main challenge in continual learning for generative models is to effectively learn new target modes with limited samples while preserving previously learned ones. To this end, we introduce a new continual learning approach for conditional generative adversarial networks by leveraging a mode-affinity score specifically designed for generative modeling. First, the generator produces samples of existing modes for subsequent replay. The discriminator is then used to compute the mode similarity measure, which identifies a set of closest existing modes to the target. Subsequently, a label for the target mode is generated and given as a weighted average of the labels within this set. We extend the continual learning model by training it on the target data with the newly-generated label, while performing memory replay to mitigate the risk of catastrophic forgetting. Experimental results on benchmark datasets demonstrate the gains of our continual learning approach over the state-of-the-art methods, even when using fewer training samples.
更多查看译文
关键词
conditional generative adversarial
AI 理解论文
溯源树
样例
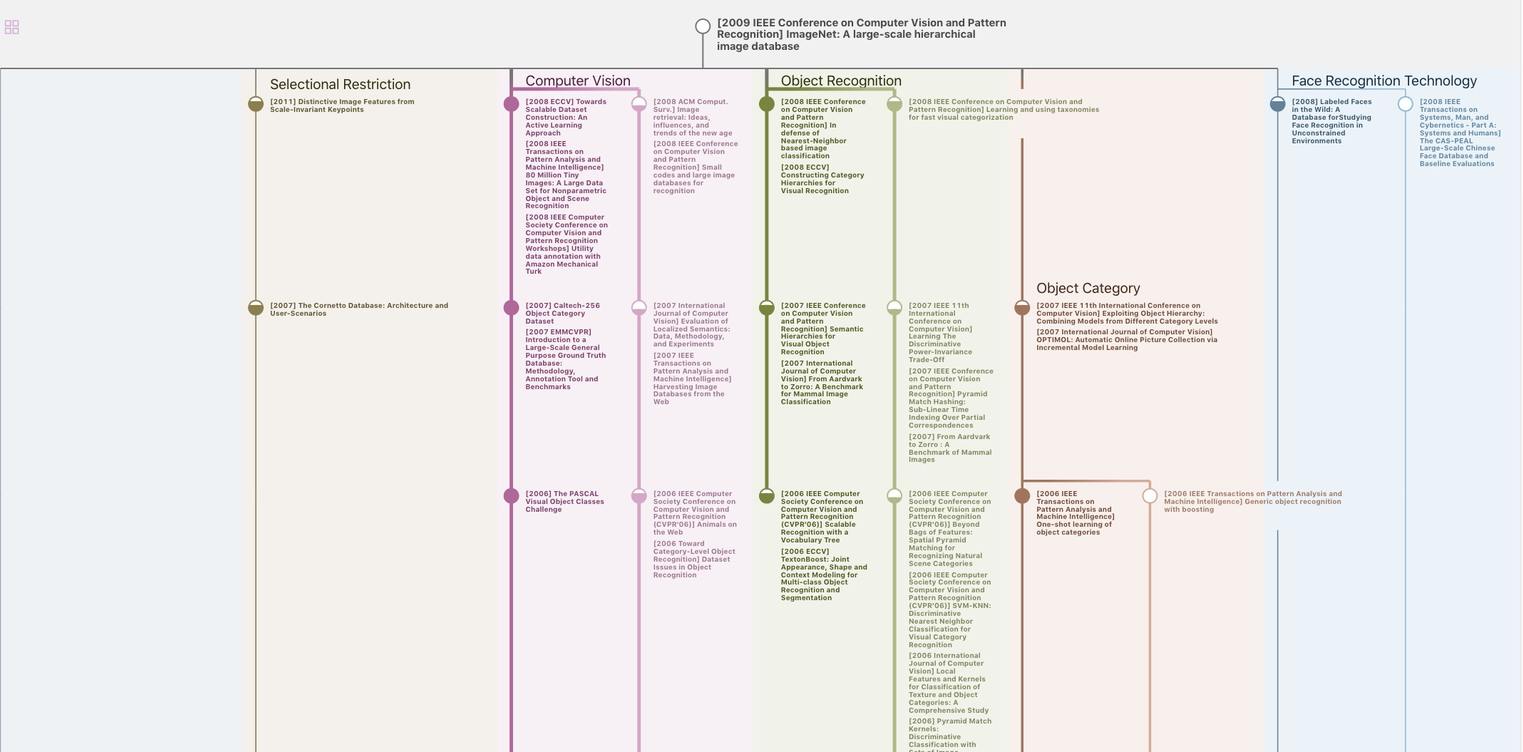
生成溯源树,研究论文发展脉络
Chat Paper
正在生成论文摘要