Chromosome 19 polygenic risk scores defined by deep learning model predict the rate of Alzheimer’ disease progression
Alzheimer's & Dementia(2023)
摘要
Background Genetic variants invariably play a crucial role in rate of disease progression. The effects of Alzheimer’s disease (AD) genetic risk factors on disease progression remains poorly understood. We developed a novel deep learning framework that could identify a personalized polygenic risk score (PRS) at the individual chromosomal level. We further used the individual’s PRS to predict the rate of cognitive decline and cerebrospinal fluid (CSF) biomarker changes. Method We applied a novel deep learning model to the 266,161 SNP chromosome 19 data of 313 AD and 457 cognitively unimpaired (CU) ADNI patients. The top 35 AD‐determining SNPs were determined and validated in an external dataset. Next, we used multiple regression to analyze the contribution of each combination of 3/35 SNPs to predict the rate of CSF accumulation (amyloid beta (Aβ), tau, phosphorylated tau (pTau), Aβ /tau, and Aβ/pTau) and the rate of cognitive decline in the memory, language, executive functioning, and visuospatial domains while controlling for age, sex, and education. Result All regression models were significant at the 0.05 level. The R‐squared adjusted values for each of the CSF models listed above were 0.917, 0.845, 0.902, 0.939, and 0.878, respectively. The majority of SNPs selected for the CSF regression models mapped to APOC1, APOC2, and TOMM40. The R‐squared adjusted values for the cognitive models were 0.188, 0.215, 0.436, and 0.266, respectively. The selected SNPs mapped to APOC1, TOMM40, VRK3, and NECTIN2. Given the less robust predictive power for cognitive change over time we increased the search to the top 150 AD SNPs. This produced R‐squared adjusted values of 0.426, 0.437, 0.607, and 0.557, respectively. The selected SNPS mapped to TOMM40, APOC1P1, ERCC1, IGSF23, ZNF556, CKM, APOC2, ZNF473, and CEACAM22P. Conclusion Our deep learning model PRS can successfully predict the AD cognitive and biomarker changes over time. Our model’s capability in deciphering the impact of SNPs on AD development could prove critical for early presymptomatic risk assessment and personalized therapeutic strategy approaches.
更多查看译文
关键词
polygenic risk scores,alzheimer,deep learning model,deep learning
AI 理解论文
溯源树
样例
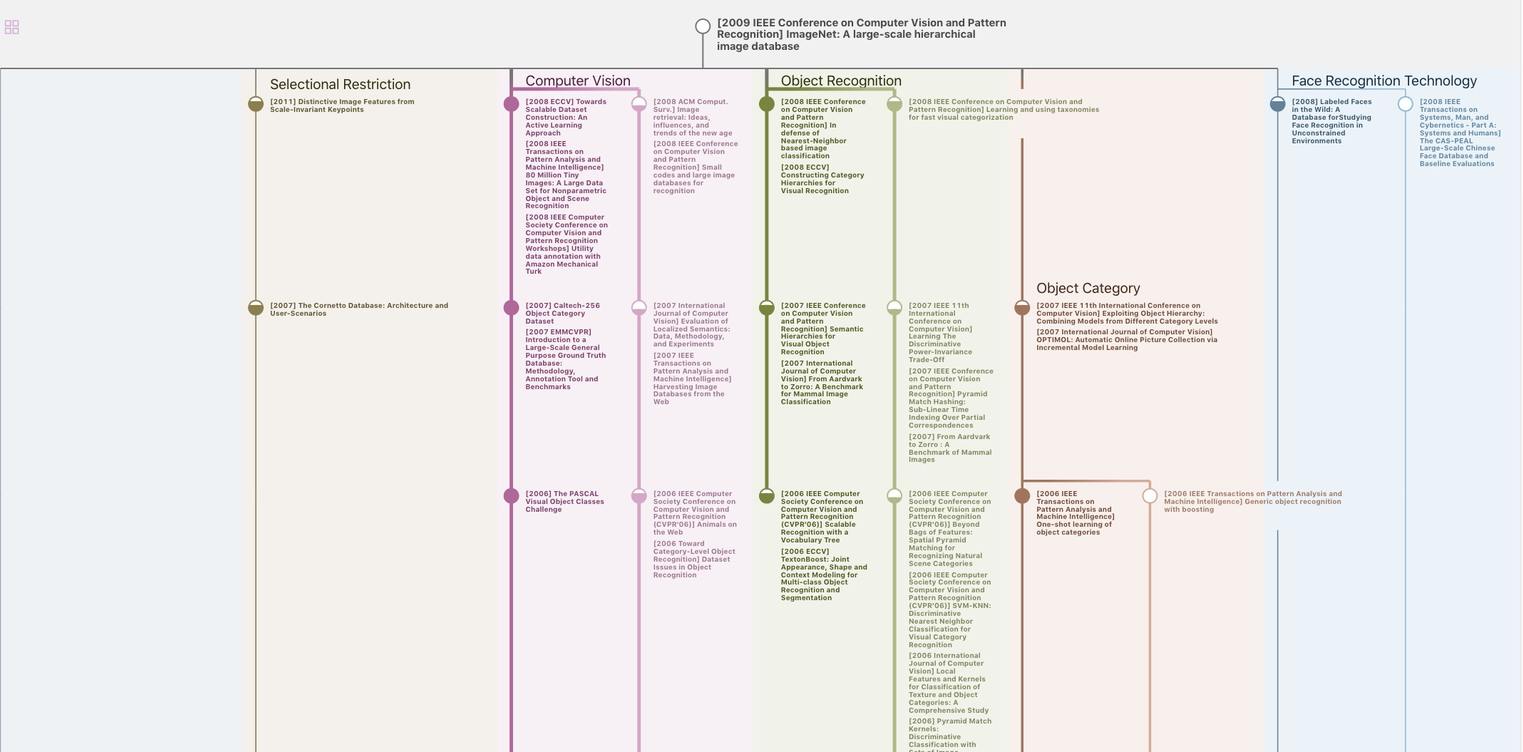
生成溯源树,研究论文发展脉络
Chat Paper
正在生成论文摘要