Predicting biological brain age using deep learning methods
Alzheimer's & Dementia(2023)
摘要
Background The interplay of genetic and environmental factors can trigger a cascade of neuropathological changes leading to individual differences in brain aging. The apparent age of the brain (brain age) can differ from chronological age, potentially reflecting different resilience mechanisms. Brain age has also the potential as biomarker to predict future cognitive impairments and dementia. In this study, we developed a biological measure of brain age, based on the differences between predicted brain age (PBA) and chronological age (CA) using deep learning model (DLM). Method The sample included 16734 T1‐w MRI from multiple time‐points of 15115 healthy individuals, aged 32‐96 yrs., from: 1) ADNI (n = 1489); 2) AIBL (n = 957); GENIC (n = 406); 4) UK Biobank (n = 13882). Healthy status was defined as absence of dementia/cognitive impairment, neuro‐psychiatric disorders, and/or self‐report of good health. Medical diagnoses were collected through physician‐/self‐reports or medical records following the International Classification of Diseases. A DLM based on convolutional neural networks was trained to develop an algorithm to predict brain age from raw MRI images registered to the MNI space using training (90%) and hold‐out test (10%) sets. To assess the model generalizability, we applied the algorithm in the Gothenburg H70‐Birth Cohort 1944 (n = 792 septuagenarians). Result The hold‐out method achieved a mean absolute error (MAE) error, between PBA and CA, of 3.04 yrs. In the H70 cohort the mean PBA was 73.9±1.49 [64.7‐86.1] years. Conclusion The model shows accurate predictions, comparable with those from previous studies using other computation methods. As next steps, we will evaluate hyperparameter optimization and implementation of a cross‐validation methodology. We also seek to include further cohorts of healthy individuals with heterogeneous age‐span to increase the model reliability and generalizability. This will enable to address e.g., differences between normal and pathological aging as well as exploring resilience mechanisms.
更多查看译文
关键词
biological brain age,deep learning methods
AI 理解论文
溯源树
样例
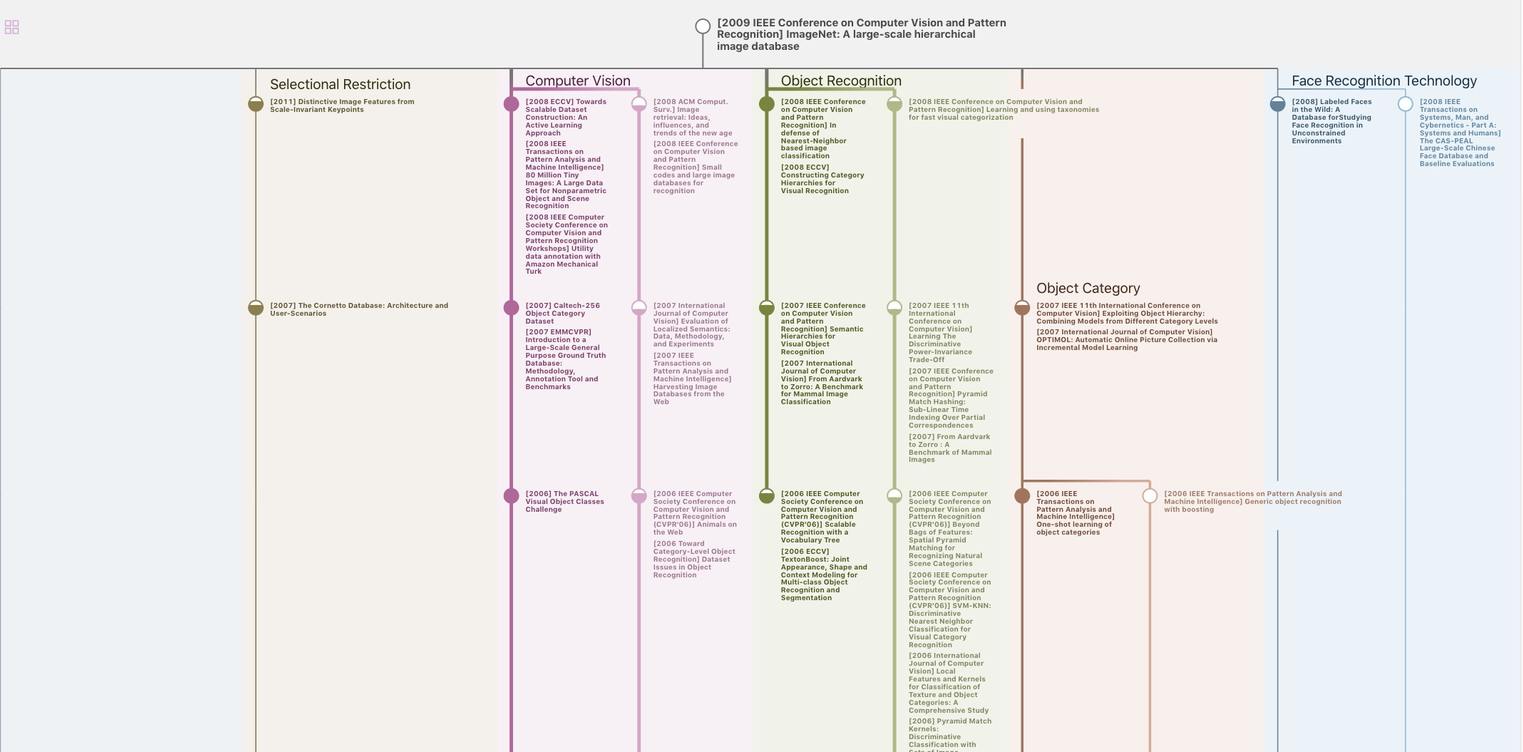
生成溯源树,研究论文发展脉络
Chat Paper
正在生成论文摘要