Facial Image Generation with Limited Training Data
Proceedings of the West Virginia Academy of Science(2023)
摘要
Deep learning models have a wide number of applications including generating realistic-looking images. These models typically require lots of data, but we wanted to explore how much quality is sacrificed by using smaller amounts of data. We built several models and trained them at different dataset sizes, then we assessed the quality of the generated images with the widely used FID measure. As expected, we measured an inverse correlation of -0.7 between image quality and training set size. However, we observed that the small-training-set results had problems not detectable by this experiment. We therefore present an experimental design for a follow-up study that would further explore the lower limits of training set size. These experiments are important for bringing us closer to understanding how much data is needed to train a successful generative model.
更多查看译文
关键词
facial image generation,training,data
AI 理解论文
溯源树
样例
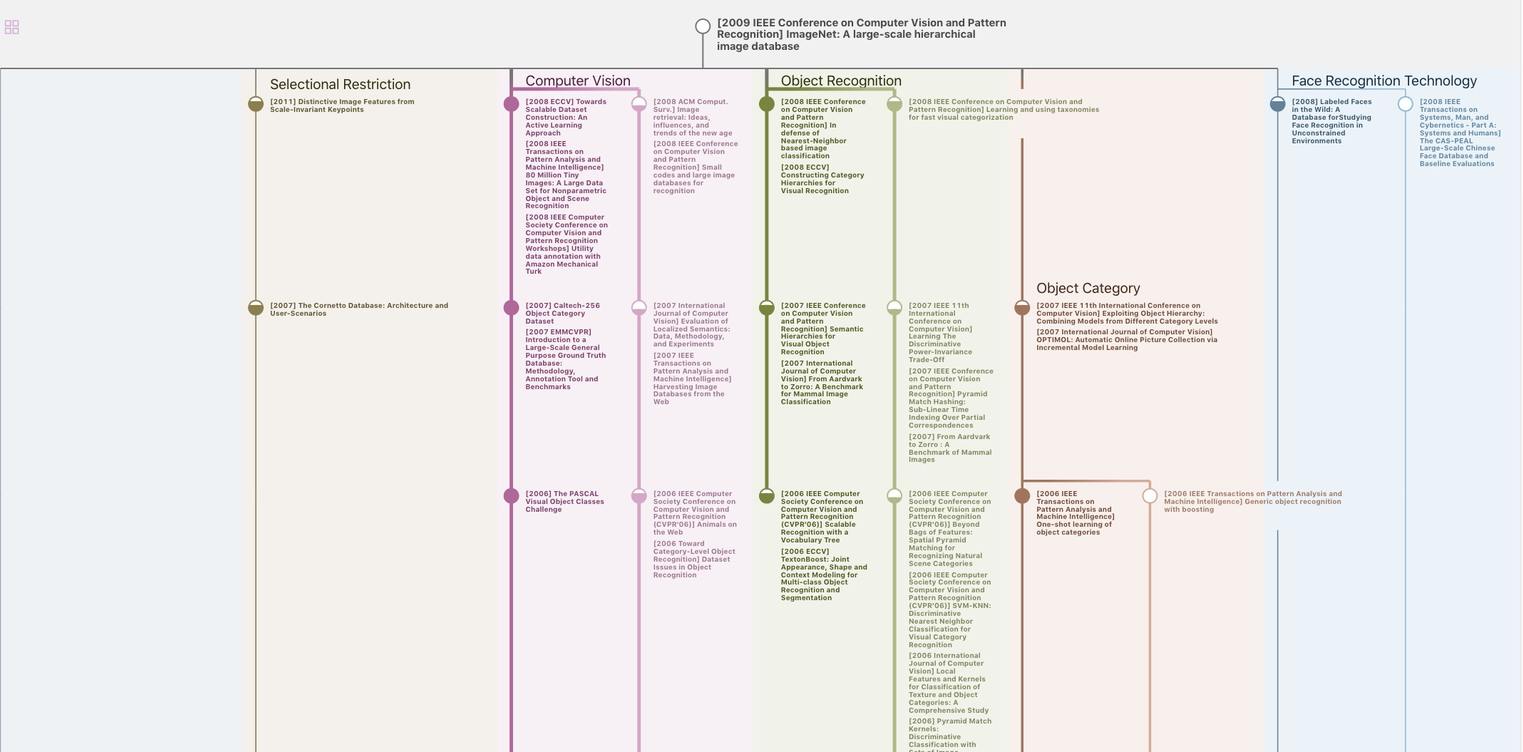
生成溯源树,研究论文发展脉络
Chat Paper
正在生成论文摘要