HemorrhageEvaluation AndDetectorSystem forUnderservedPopulations:HEADS UP
medRxiv (Cold Spring Harbor Laboratory)(2023)
摘要
Abstract Introduction Intracerebral hemorrhage (ICH) is the second most common cause of stroke and remains the second leading cause of disability impacting underserved areas. Since 2015, there has been a paradigm shift in managing ischemic stroke through applying AI and ML. However, ICH patients lack such protocol. Objective To create a rapid, cloud-based, and deployable ML method to detect ICH potentially across the Mayo Clinic enterprise then expand to involve underserved areas. Methods We utilized RSNA dataset for ICH. We made four total iterations using Google Cloud Vertex AutoML. We trained an AutoML model with 2,000 images followed by 6,000 images from both ICH positive and negative classes. Pixel values were measured by the Hounsfield units presenting a width of 80 Hounsfield and a level of 40 Hounsfield as the bone window. This was followed by a more detailed image preprocessing approach by combining the pixel values from each of the brain, subdural, and soft tissue window-based grayscale images into R(red)G(green)B(blue)-channel images to boost the binary ICH classification performance. Four experiments with AutoML were applied to study the impacts of training sample size and image preprocessing on model performance. Results Out of the four AutoML experiments, the best-performing model achieved a 95.8% average precision, 91.4% precision, and 91.4% recall. Based on this analysis, our binary ICH classifier HEADS UP is both accurate and performant. Conclusion HEADS UP , is a rapid, cloud-based, deployable ML method to detect ICH. This tool can help expedite the care of patients with ICH in resource-limited hospitals.
更多查看译文
关键词
<i>h</i>emorrhage<i>e</i>valuation,and<i>d</i>etector<i>s</i>ystem
AI 理解论文
溯源树
样例
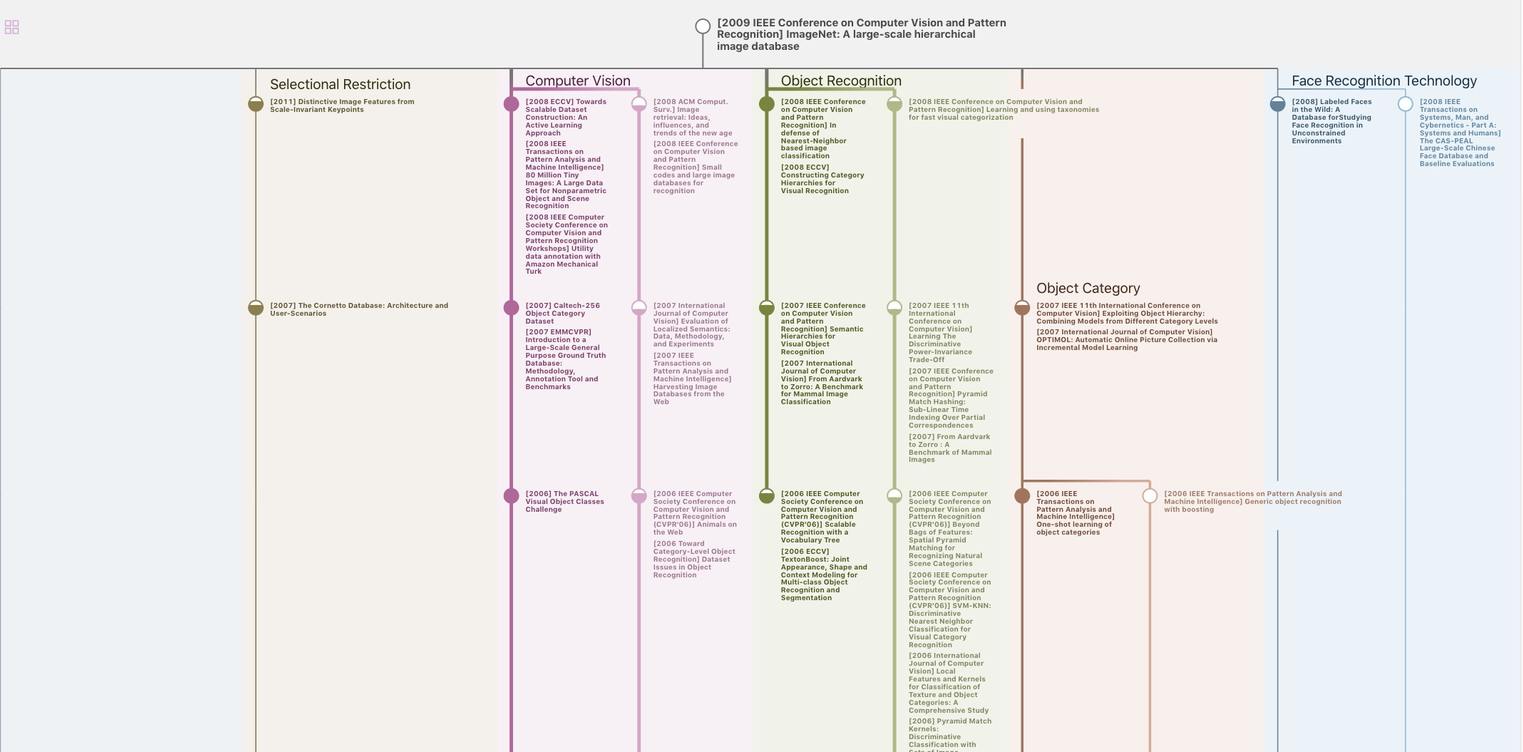
生成溯源树,研究论文发展脉络
Chat Paper
正在生成论文摘要