A Reinforcement Learning Framework with Region-Awareness and Shared Path Experience for Efficient Routing in Networks-on-Chip
arXiv (Cornell University)(2023)
摘要
Network-on-chip (NoC) architectures provide a scalable, high-performance, and reliable interconnect for emerging manycore systems. The routing policies used in NoCs have a significant impact on overall performance. Prior efforts have proposed reinforcement learning (RL)-based adaptive routing policies to avoid congestion and minimize latency in NoCs. The output quality of RL policies depends on selecting a representative cost function and an effective update mechanism. Unfortunately, existing RL policies for NoC routing fail to represent path contention and regional congestion in the cost function. Moreover, the experience of packet flows sharing the same route is not fully incorporated into the RL update mechanism. In this paper, we present a novel regional congestion-aware RL-based NoC routing policy called Q-RASP that is capable of sharing experience from packets using the same routes. Q-RASP improves average packet latency by up to 18.3% and reduces NoC energy consumption by up to 6.7% with minimal area overheads compared to state-of-the-art RL-based NoC routing implementations.
更多查看译文
关键词
efficient routing,reinforcement learning framework,reinforcement learning,region-awareness,networks-on-chip
AI 理解论文
溯源树
样例
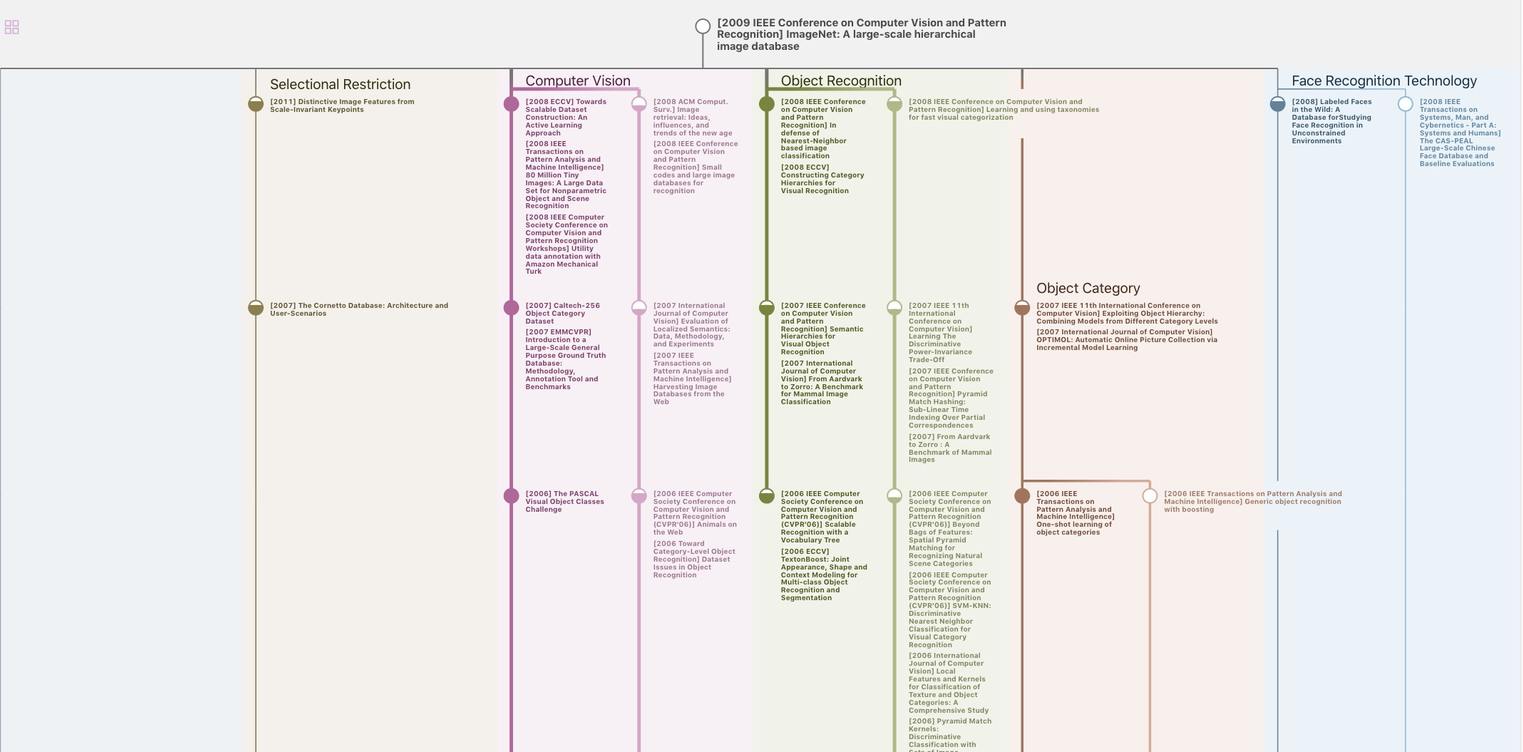
生成溯源树,研究论文发展脉络
Chat Paper
正在生成论文摘要