Towards a Quantitative Understanding of Microstructure-Property Relationships
Springer series in materials science(2023)
摘要
Abstract 100 years ago, the concept of tortuosity was introduced by Kozeny in order to express the limiting influence of the microstructure on porous media flow. It was also recognized that transport is hindered by other microstructure features such as pore volume fraction, narrow bottlenecks, and viscous drag at the pore surface. The ground-breaking work of Kozeny and Carman makes it possible to predict the macroscopic flow properties (i.e., permeability) based on the knowledge of the relevant microstructure characteristics. However, Kozeny and Carman did not have access to tomography and 3D image analysis techniques, as it is the case nowadays. So, their descriptions were developed by considering simplified models of porous media such as parallel tubes and sphere packings. This simplified setting clearly limits the prediction power of the Carman-Kozeny equations, especially for materials with complex microstructures. Since the ground-breaking work of Kozeny and Carman many attempts were undertaken to improve the prediction power of quantitative expressions that describe the relationship between microstructure characteristics (i.e., tortuosity τ , constrictivity β , porosity ε , hydraulic radius r h ) and effective transport properties (i.e., conductivity σ eff , diffusivity D eff , permeability к ,). Due to the ongoing progress in tomography, 3D image-processing, stochastic geometry and numerical simulation, new possibilities arise for better descriptions of the relevant microstructure characteristics, which also leads to mathematical expressions with higher prediction power. In this chapter, the 100-years evolution of quantitative expressions describing the micro–macro relationships in porous media is carefully reviewed,—first, for the case of conduction and diffusion,—and second, for flow and permeability. The following expressions are the once with the highest prediction power: $$\sigma_{eff} \left( {or D_{eff} } \right) = \varepsilon^{1.15} \beta^{0.37} /\tau_{{dir_{geodesic} }}^{4.39} ,$$ σ eff o r D eff = ε 1.15 β 0.37 / τ d i r geodesic 4.39 , for conduction and diffusion, and $$\kappa_{I} = 0.54\left( {\frac{\varepsilon }{{S_{V} }}} \right)^{2} \frac{{\varepsilon^{3.56} \beta^{0.78} }}{{\tau_{dir\_geodesic}^{1.67} }},$$ κ I = 0.54 ε S V 2 ε 3.56 β 0.78 τ d i r _ g e o d e s i c 1.67 , $$\kappa_{II} = \frac{{\left( {0.94r_{min} + 0.06r_{max} } \right)^{2} }}{8} \frac{{\varepsilon^{2.14} }}{{\tau_{dir\_geodesic}^{2.44} }},$$ κ II = 0.94 r min + 0.06 r max 2 8 ε 2.14 τ d i r _ g e o d e s i c 2.44 , both, for permeability in porous media.
更多查看译文
关键词
quantitative understanding,microstructure-property
AI 理解论文
溯源树
样例
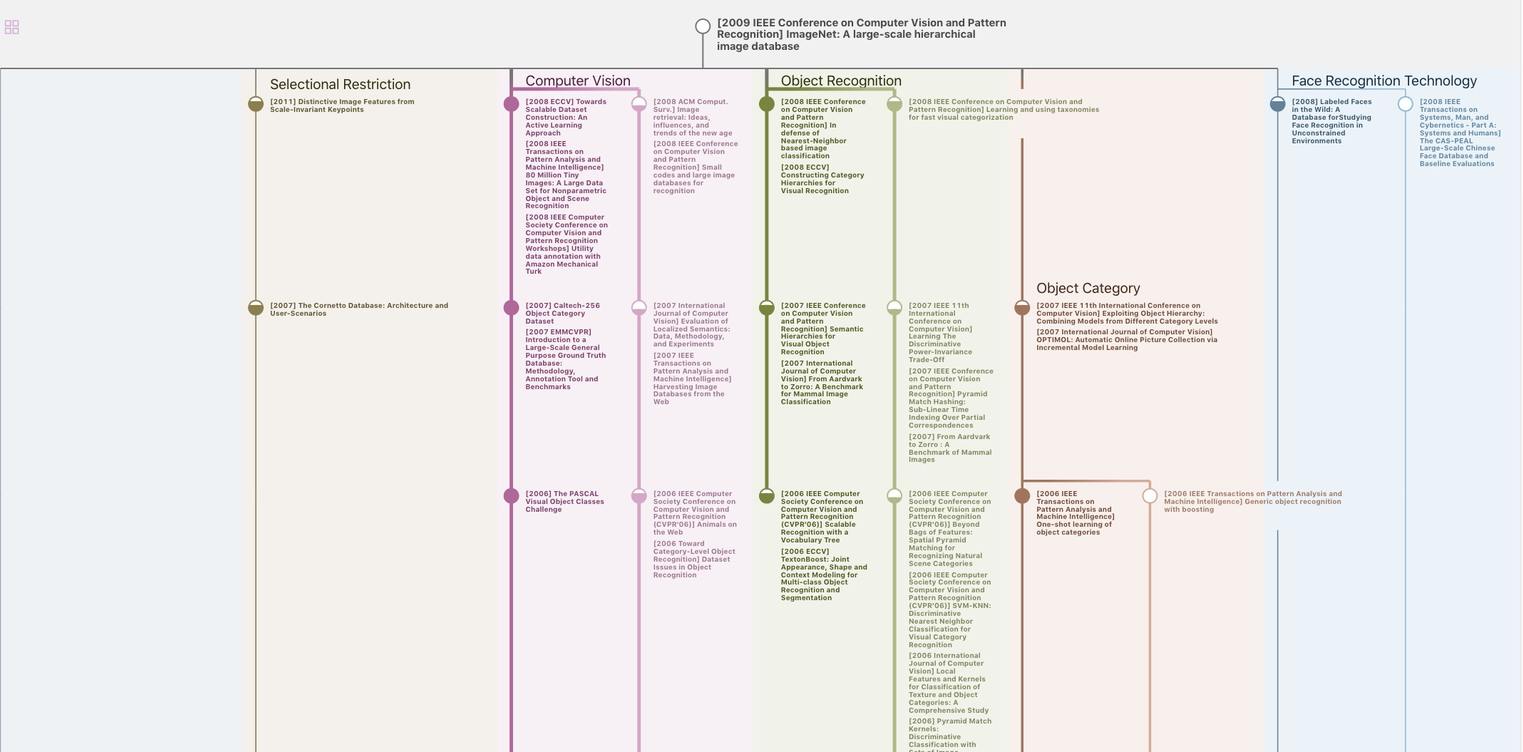
生成溯源树,研究论文发展脉络
Chat Paper
正在生成论文摘要