Optimizing Lung Cancer Prediction Using Evaluating Classification Methods and Sampling Techniques
Journal Medical Informatics Technology(2023)
摘要
Lung cancer is an extremely aggressive type of cancer and one of the leading causes of death globally. The focus of this study is to improve the detection and prediction of lung cancer by evaluating different approaches for classification and sampling. The research utilizes a dataset comprising 1000 patients and 24 Attributes. The primary goal is to compare the effectiveness of classification methods like Logistic Regression, AdaBoost, and GradientBoosting, in conjunction with diverse sampling techniques such as Random Over-Sampling, RandomUnder-Sampling, and SMOTE by Level Considering, for predicting lung cancer. The assessment metrics includeaccuracy, precision, recall, and F1-score. The experimental findings demonstrate that Gradient Boosting (GBoost) attains flawless accuracy, precision, recall, and F1-score results of 100% when identifying lung cancer instances within the dataset. This highlights the effectiveness of GBoost in accurately predicting lung cancer occurrence. The findings of this research aim to contribute significantly to the development of more effective diagnostic and predictive methods for lung cancer.
更多查看译文
关键词
lung cancer prediction,lung cancer,evaluating classification methods
AI 理解论文
溯源树
样例
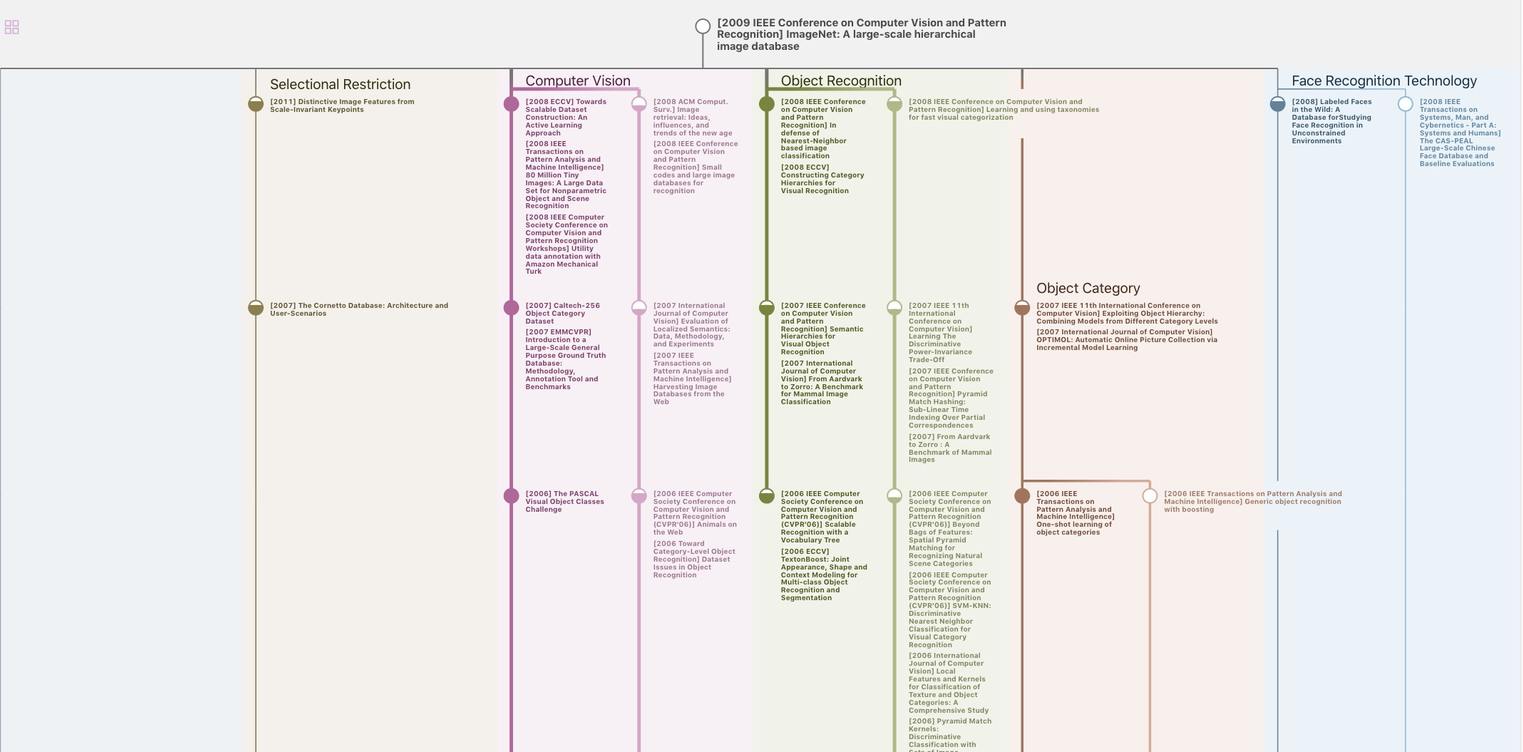
生成溯源树,研究论文发展脉络
Chat Paper
正在生成论文摘要