Acceleration of Molecular Simulations by Parametric Time-Lagged tSNE Metadynamics
JOURNAL OF PHYSICAL CHEMISTRY B(2024)
摘要
The potential of molecular simulations is limited by their computational costs. There is often a need to accelerate simulations using some of the enhanced sampling methods. Metadynamics applies a history-dependent bias potential that disfavors previously visited states. To apply metadynamics, it is necessary to select a few properties of the system-collective variables (CVs) that can be used to define the bias potential. Over the past few years, there have been emerging opportunities for machine learning and, in particular, artificial neural networks within this domain. In this broad context, a specific unsupervised machine learning method was utilized, namely, parametric time-lagged t-distributed stochastic neighbor embedding (ptltSNE) to design CVs. The approach was tested on a Trp-cage trajectory (tryptophan cage) from the literature. The trajectory was used to generate a map of conformations, distinguish fast conformational changes from slow ones, and design CVs. Then, metadynamic simulations were performed. To accelerate the formation of the alpha-helix, we added the alpha-RMSD collective variable. This simulation led to one folding event in a 350 ns metadynamics simulation. To accelerate degrees of freedom not addressed by CVs, we performed parallel tempering metadynamics. This simulation led to 10 folding events in a 200 ns simulation with 32 replicas.
更多查看译文
关键词
molecular simulations,time-lagged
AI 理解论文
溯源树
样例
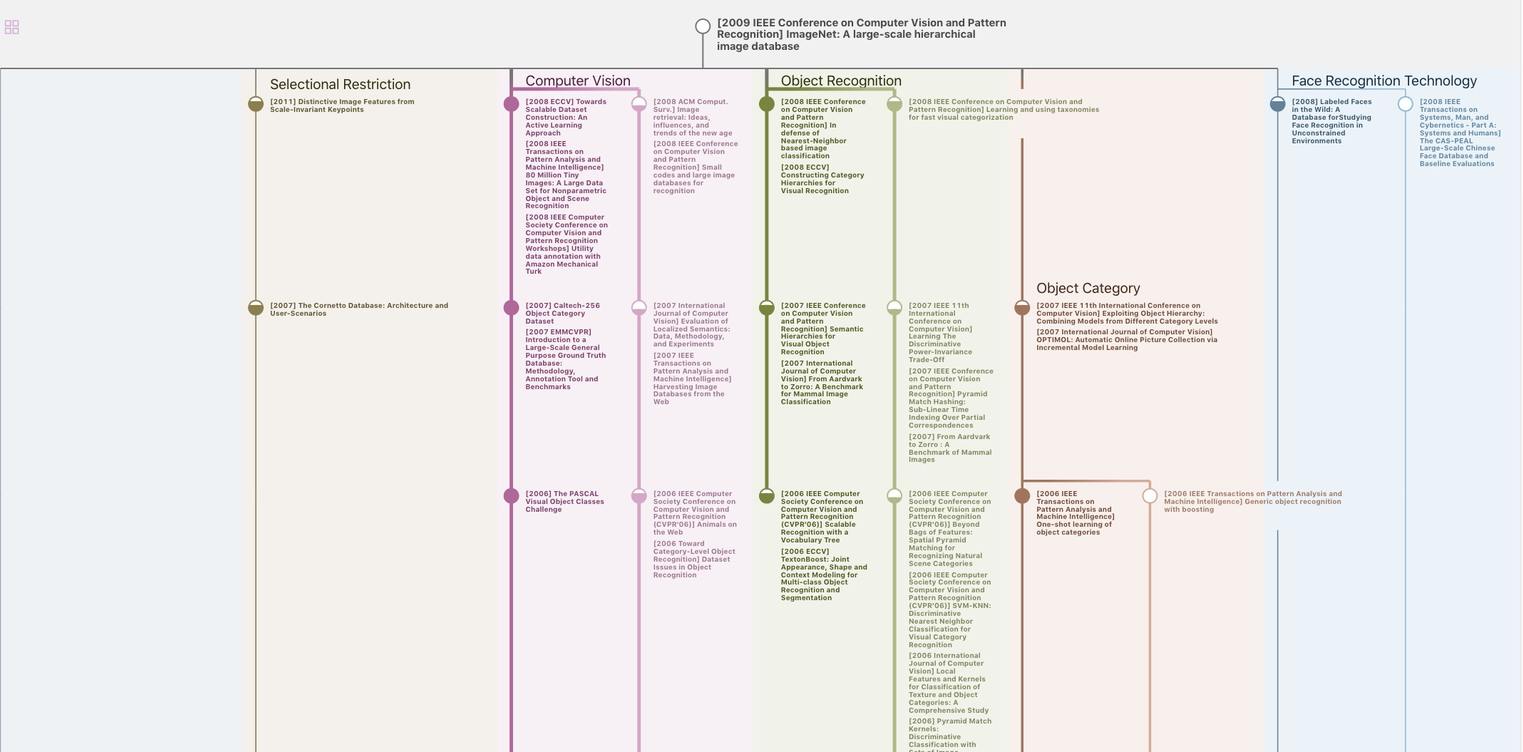
生成溯源树,研究论文发展脉络
Chat Paper
正在生成论文摘要